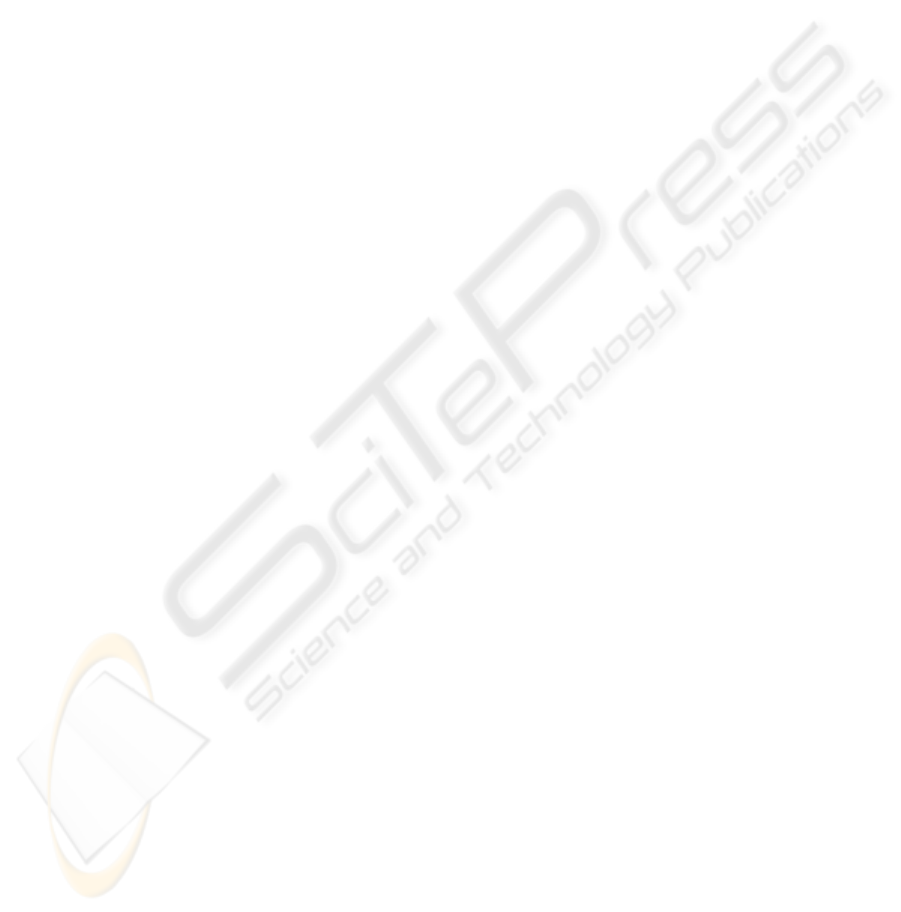
10 frames are used for the background representation. Small f
m
values until frame no
900 shown in Figure 1(a) illustrate that the initial background representation is
successful. Moreover, when a motion is detected in the scene, the plot starts to
fluctuate. The significant increases in the plot correspond to appearance of a new
foreground object whereas the sharp drops refer to the stopping objects that integrated
into the background representation. As it is expected, when the contribution of the last
observation is small means for smaller values of
, the reconstruction error reaches to
higher levels without a major change in the characteristics of the distribution (Figure
1(a)). It should also be noted that, since consecutive frames are very similar in
surveillance video, convergence is quickly achieved in a small iteration number.
We have tested the dynamic background modeling performances of the batch NMF
and the proposed incremental NMF representations on the same video frames. Rank
is set to
r=2 for both models and
is set to 0.2 for INMF. Figure 1(b) illustrates
distribution of the reconstruction error versus frame number. It is observable that the
reconstruction error of INMF remains much smaller than that of batch NMF.
Furthermore, although the error is small for both decompositions at the beginning, it
never drops to the initial value for the batch NMF. This is because the batch process
cannot adapt the background representation according to content changes properly.
This makes it unsuitable to an on-line video content analysis. However, the proposed
incremental NMF is capable of updating the initial background model according to
the dynamic changes. For this reason, the reconstruction error drops to the initial
value whenever all of the moving objects become part of the background.
Figure 2 visually demonstrates performance in tracking the foreground objects and
updating the background model for the NMF, INMF and IPCA. Figure 2(a) illustrates
frame 971 taken from the dataset1 training camera 1 video sequence of PETS2001
dataset. In this frame, a new car (car 1) enters to the scene as the green car (car 2) in
the corner starts to leave from the parking lot. In Figure 2b, which corresponds to
frame number 1436, car 1 parked to a slot next to the red car and stopped. Car 2 left
out its parking slot and moved to the left, thus it is about leaving the scene. In
addition, two new walking men exist in this frame. Therefore, it is expected that a
powerful method should detect the moving objects which are the two men and car 2 in
this scene. In fact, as it is shown in Figure 2(c), (d) and (e), three of the methods are
capable of detecting these foreground objects. However, as it is observable in Figure
2(c), the batch NMF also detects the parked car 1 as a foreground object.
Furthermore, the old location of car 2 is also not cleared. The reason for their
existence is that the batch NMF is not capable of updating the background model and
fails to include car 2 into the background model and to remove car 2’s old location
from the background. However, adaptive updating of the background model is
achieved by the INMF successfully (Figure 2(d)). Hence, the proposed INMF is
capable of controlling the algorithm’s adaptability to dynamic content changes. As it
can be seen in Figure 2(e), the IPCA shows a similar performance.
Superiority of the INMF on IPCA becomes much clearer under the illumination
changes that may frequently occur in an outdoor surveillance video scene. The
distribution of
f
m
for the frames 2600 to 2990 of dataset2 training camera 2 of
PETS2001 database is plotted in Figure 3. Plots are obtained by the INMF with
r=2,
=0.05 and IPCA with r=2,
=0.95. As it is shown, the minimum reconstruction
error obtained by the IPCA remains much higher than the error of INMF. Furthermore
113