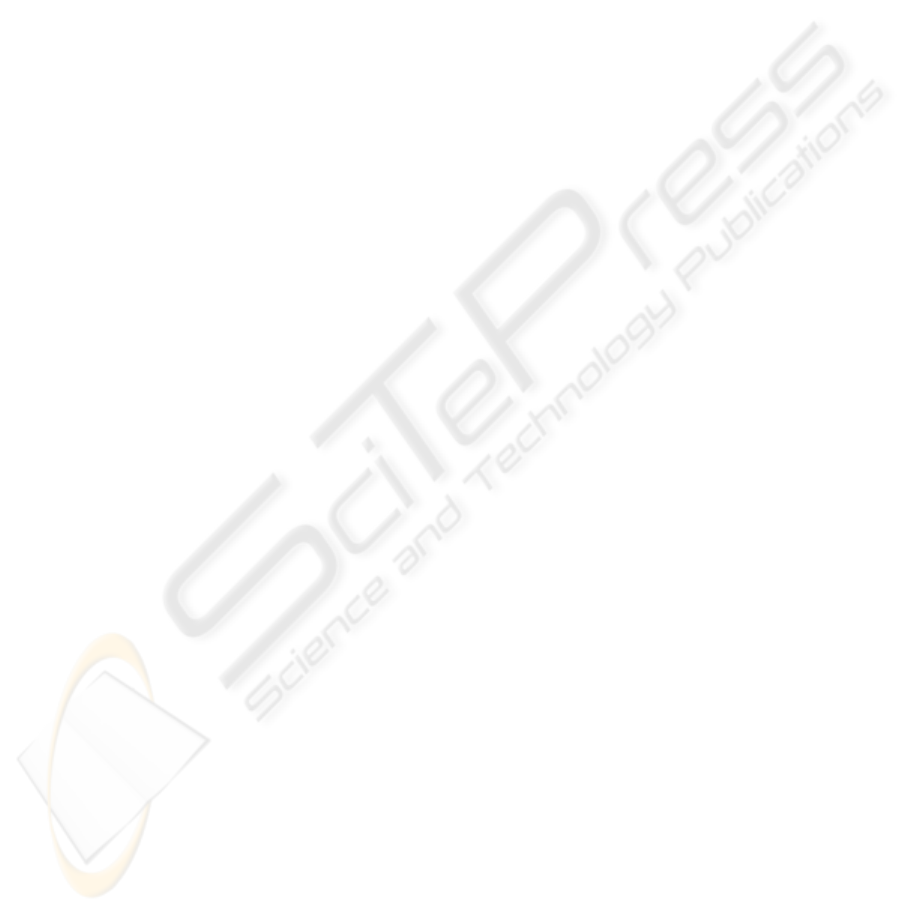
8. Aussenac-Gilles, N., Sörgel, D.: Text analysis for ontology and terminology engineering.
Applied Ontology. n°1. pp. 35-46
9. Daille, B.: Recent Trends in Computational Terminology. Special issue of Terminology
10:1 (2004). Edited by Béatrice Daille, Kyo Kageura, Hiroshi Nakagawa and Lee-Feng
Chien, Benjamins publishing company
10. Harris, Z.: Mathematical Structures of Language. 1968, reprint 1979. R.E. Krieger
Publishing Company, Inc.
11. http://wordnet.princeton.edu/
12. Kiryakov, A., Popov, B., Terziev, I., Manov, D., Ognyanoff, D.: Semantic Annotation,
Indexing, and Retrieval.Elsevier's Journal of Web Sematics, Vol. 2, Issue (1), 2005
13. Grice, H.P.: Meaning. Philosophical Review n°66. pp 377-88, 1957
14. Cruse, D.A.: Lexical Semantics. Cambridge University Press 1986
15. Ushold, M., Gruninger, M.: Ontologies: Principles, Methods and Applications. Knowledge
Engineering Review, Vol. 11, n° 2, June 1996. Also available from AIAI as AIAI-TR-191
16. Gruber, T.: A Translation Approach to Portable Ontology Specifications. Knowledge
Systems Laboratory September 1992 - Technical Report KSL 92-71 Revised April 1993.
Appeared in Knowledge Acquisition, 5(2):199-220, 199
17. Sapir, E.: Language. An Introduction to the study of speech. Docer Publications, 2004.
Originally published by Harcourt, Brace and Company, 1921)
18. Whorf, B.L.: Language, Thought and Reality. The MIT Press, 1956
19. Baader, F., Calvanese, D., McGuiness, D., Nardi, D., Patel-Schneider, P.: The Description
Logic Handbook. Franz Baader, Diego Calvanese, Deborah McGuinness, Daniele Nardi,
Peter Patel-Schneider, editors. Cambridge University Press, 2003
20. Wright, J. M., Fox, M.S., Adam, D.: 84 "SRL/1.5 Users Manual." Technical
report;Robotics Institute, Carnegie-Mellon University 1984.
21. Woods 75. What’s in a Link: Foundations for Semantic Networks. Representation and
Understanding: Studies in Cognitive Science, 35-82, edited by D.G. Bobrow and A.M.
Collins, New York: Academic Press, 1975.
22. OWL Web Ontology Language: http://www.w3.org/TR/owl-features/
23. Guarino, N., Carrara, M., Giaretta, P.: An Ontology of Meta-Level Categories. of
Knowledge Representation and Reasoning: Proceedings of the Fourth International
Conference (KR94), Morgan Kaufmann, San Mateo, CA.
24. Kaplan, A.: Towards a consistent logical framework for ontological analysis. FOIS’01.
October 17-19, 2001, Ogunquit, USA.
25. Roche, C.: The ‘Specific-Difference’ Principle: a Methodology for Building Consensual
and Coherent Ontologies. IC-AI’2001: Las Vegas, USA, June 25-28 2001
26. Spies, M., Roche, C.: Aristotelian ontologies and OWL modelling. Third International
Workshop on Philosophy and Informatics. Saarbrücken, Germany - May 3-4, 2006
56