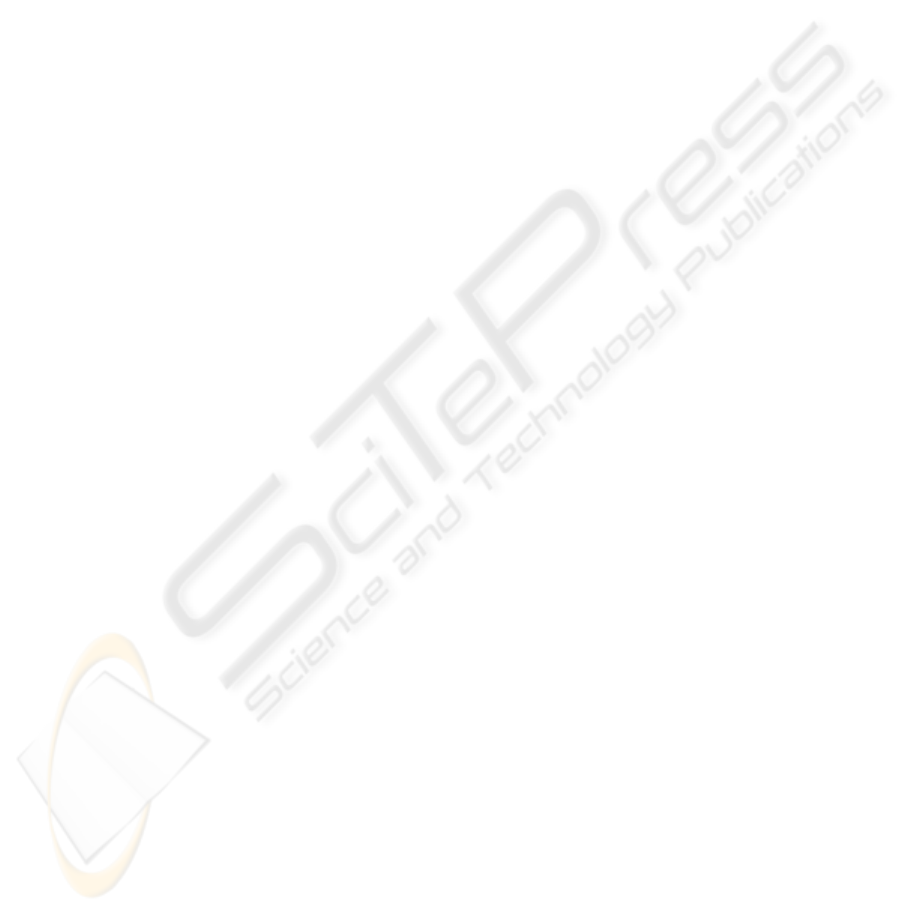
statistical properties expressed by small number of support vectors and high value of
estimated VC dimension.
The system is fast enough. The TSVM solution for a data set of several hundreds
points is of order 20-30 seconds, the recognition time is about 0.1 s.
It can be concluded that the transductive support vector machine is an efficient tool
of computer-aided medical data recognition. It enables the improvement of results for
labelled data by exploring much larger set of unlabelled data.
References
1. AHCPR 1998: U.S. Department of Health and Human Services, Public Health Service,
Agency for Healthcare Policy and Research (AHCPR). Signal-averaged electrocardiogra-
phy. Health Technology Assessment No. 11. AHCPR Pub. No. 98-0020. Rockville (MD):
AHCPR; 1998 May
2. Bennett K., A. Demiriz: Semi-supervised support vector machines, Advances in Neural
Information Processing Systems NIPS 12, MIT Press, 1998, 368-374
3. Breithardt G., Cain M., El-Sherif N., et al. Standards for analysis of ventricular late poten-
tials using high resolution or signal-averaged electrocardiography. A statement by a task
force committee of the European Society of Cardiology, the American Heart Association,
and the American College of Cardiology. Circulation 1991. 83 (4): 1481-1488
4. Brembilla-Perrot B. et al.: Value of non-invasive and invasive studies in patients with
bundle branch block, syncope and history of myocardial infarction. Europace (2001) 3,
187–194
5. Cain et al. ACC expert consensus document. Signal Averaged electrocardiography. J.
Amer. Coll. Cardiol 1996; 27:238-49
6. Chapelle O. , B. Schölkopf, A. Zien (eds.): Semi-Supervised Learning, MIT Press, Cam-
bridge, 2006
7. Collobert R., F. Sinz, J. Weston, L. Bottou: Large Scale Transductive SVMs, Journal of
Machine Learning Research 7 (2006), 1687-1712
8. Gomes J.A.: Signal averaged electrocardiography – concepts, methods and applications.
Kluwer Academic Publishers, 1993
9. Heidari H. et all: Analysis of the Sustained Ventricular Arrhythmias from SAECG Using
Artificial Neural Network and Fuzzy Clustering Algorithm; Proc. 20th Annual Interna-
tional Conference of the IEEE Engineering in Medicine and Biology Society, Vol. 20, No
1,1998
10. Jankowski S., J. Tijink, G. Vumbaca, M. Balsi, G. Karpiński: Morphological analysis of
ECG Holter recordings by support vector machines, Medical Data Analysis, Third Interna-
tional Symposium ISMDA 2002, Rome, Italy, October 2002, Lecture Notes in Computer
Science LNCS 2526 (eds. A. Colosimo, A. Giuliani, P. Sirabella) Springer-Verlag, Berlin
Heidelberg New York 2002, pp. 134-143
11. Jankowski S., A. Oręziak, A. Skorupski, H. Kowalski, Z. Szymański, E. Piątkowska-Janko:
Computer-aided Morphological Analysis of Holter ECG Recordings Based on Support
Vector Learning System, Proc. IEEE International Conference Computers in Cardiology
2003, Tessaloniki, pp. 597-600
12. Joachims T.: Transductive Inference for text classification using support vector machines,
Proc. 16
th
International Conference on Machine Learning ICML, San Francisco 1999, 200-
209
169