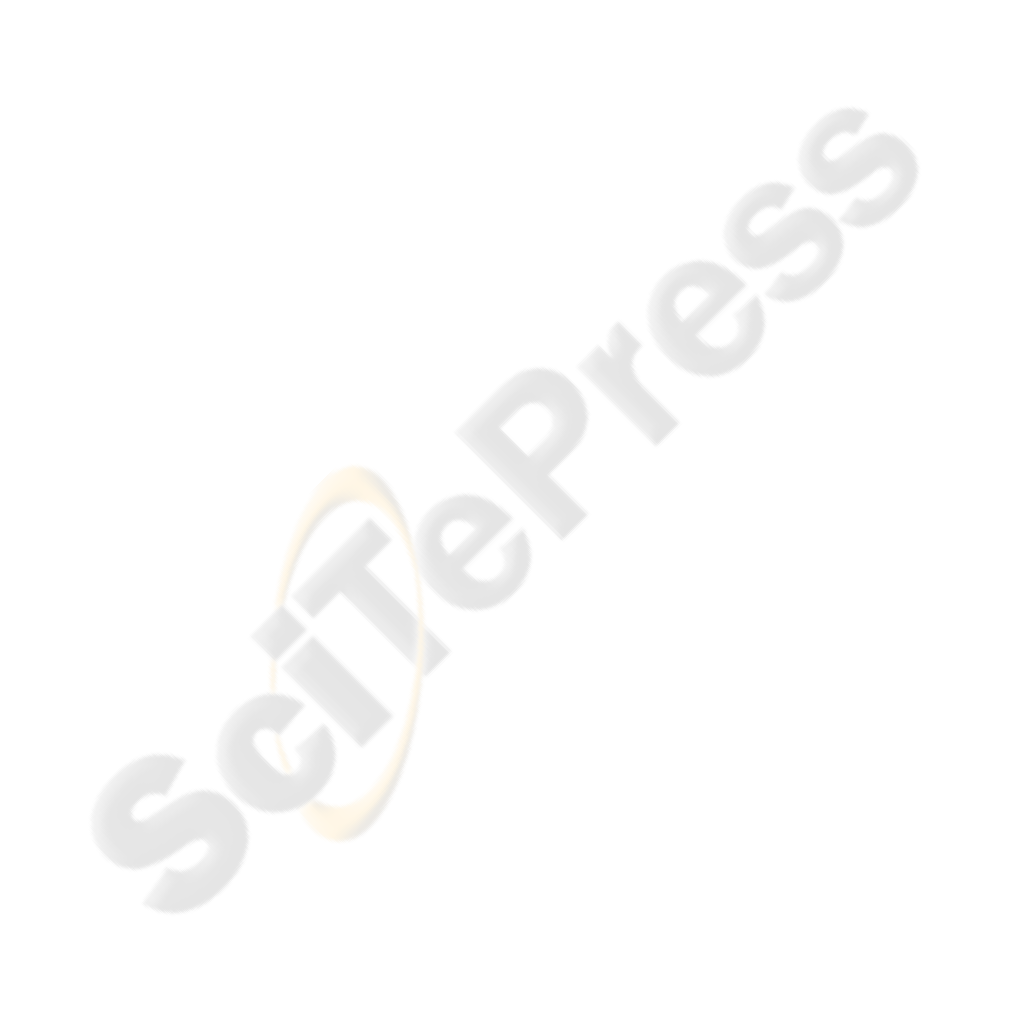
as possible and having enough test cells per well to
classify the staining pattern in accordance to the WS
criterion. In the two trials, the overall system mis-
classified only one out of the 37 wells, attaining an
hit rate equal to 97.3% and outperforming the results
reported in (Soda, 2007) (see section 1).
7 CONCLUSIONS
In this paper we have presented a system that supports
the staining pattern classification of IIF slides, whose
results show high accuracy. The approach, which pro-
vides a degree of redundancy that lowers the effect of
cell misclassifications, is based on the reliability es-
timation. The latter is unusual among the classifier
aggregation strategies.
We are currently engaged in populating a larger
database to consider not only the most relevant and
recurrent staining patterns, but also the minor ones.
Furthermore, we should apply boosting techniques to
improve binary recognition performance, especially
in the case of nuclear samples. The research goal is
a comprehensive CAD supporting all phases of IIF
diagnosis, i.e. both fluorescence intensity and staining
pattern classification.
ACKNOWLEDGEMENTS
The authors thank A. Afeltra and A. Rigon for their
collaboration in IIF images annotation. This work has
been funded by DAS s.r.l of Palombara Sabina (www.
dasitaly.com).
REFERENCES
Allwein, E. L., Schapire, R. E., and Singer, Y. (2001). Re-
ducing multiclass to binary: a unifying approach for
margin classifiers. J. Mach. Learn. Res., 1:113–141.
Bio-Rad Laboratories Inc. (2004). PhD System. USA.
Center for Disease Control (1996). Quality assurance for
the indirect immunofluorescence test for autoantibod-
ies to nuclear antigen (IF-ANA): approved guideline.
NCCLS I/LA2-A, 16(11).
Cordella, L., Foggia, P., Sansone, C., Tortorella, F., and
Vento, M. (1999). Reliability parameters to improve
combination strategies in multi-expert systems. Pat-
tern Analysis & Applications, 2(3):205–214.
Cordella, L., Foggia, P., Sansone, C., Tortorella, F., and
Vento, M. (2000). A cascaded multiple expert system
for verification. In 1st Int. Work. on Multiple Classifier
Systems, pages 330–339. Springer-Verlag.
Das s.r.l. (2004). Service Manual AP16 IF Plus. Italy.
De Stefano, C., Sansone, C., and Vento, M. (2000). To reject
or not to reject: that is the question: an answer in case
of neural classifiers. IEEE Transactions on Systems,
Man, and Cybernetics–Part C, 30(1):84–93.
Giacinto, G. and Roli, F. (2001). Dynamic classifier selec-
tion based on multiple classifier behaviour. Pattern
Recognition, 34(9):1879–1881.
Gunes, V., Menard, M., Loonis, P., and Petit-Renaud, S.
(2003). Combination, cooperation and selection of
classifiers: a state of the art. Int. Jour. of Pattern
Recognition and Artificial Intelligence, 17(8):1303–
1324.
Jelonek, J. and Stefanowski, J. (1998). Experiments on
solving multiclass learning problems by n
2
classi-
fier. In 10th Eur. Conf. on Mach. Learn., pages 172–
177. Springer-Verlag Lecture Notes in Artificial Intel-
ligence.
Kuncheva, L. (2002). Switching between selection and fu-
sion in combining classifiers: an experiment. IEEE
Transactions on Systems, Man and Cybernetics,
32(2):146–156.
Kuncheva, L., Bezdek, J., and R.P.W.Duin (2001). Decision
template for multiple classifier fusion: an experimen-
tal comparison. Pattern Recognition, 34:299–314.
Perner, P., Perner, H., and Muller, B. (2002). Mining knowl-
edge for HEp-2 cell image classification. Journal Ar-
tificial Intelligence in Medicine, 26:161–173.
Rigon, A., Soda, P., Zennaro, D., Iannello, G., and Afeltra,
A. (2007). Indirect immunofluorescence (IIF) in au-
toimmune diseases: Assessment of digital images for
diagnostic purpose. Cytometry - In press.
Sack, U., Knoechner, S., Warschkau, H., and et al.
(2003). Computer-assisted classification of HEp-2
immunofluorescence patterns in autoimmune diagnos-
tics. Autoimmunity Reviews, 2:298–304.
Soda, P. (2007). Early experiences in the staining pattern
classification of HEp-2 slides. In Computer Based
Medical Systems, pages 219–224. IEEE Computer So-
ciety.
Soda, P. and Iannello, G. (2006). A multi-expert system to
classify fluorescent intensity in antinuclear autoanti-
bodies testing. In Computer Based Medical Systems,
pages 219–224. IEEE Computer Society.
Soda, P. and Iannello, G. (2007). Reliability estimation in
the classifier aggregation framework. Technical re-
port, Universit
`
a Campus Bio-Medico di Roma.
Xu, L., Krzyzak, A., and Suen, C. (1992). Method of
combining multiple classifiers and their application to
handwritten numeral recognition. IEEE Transactions
on Systems, Man and Cybernetics, 22(3):418–435.
HEALTHINF 2008 - International Conference on Health Informatics
236