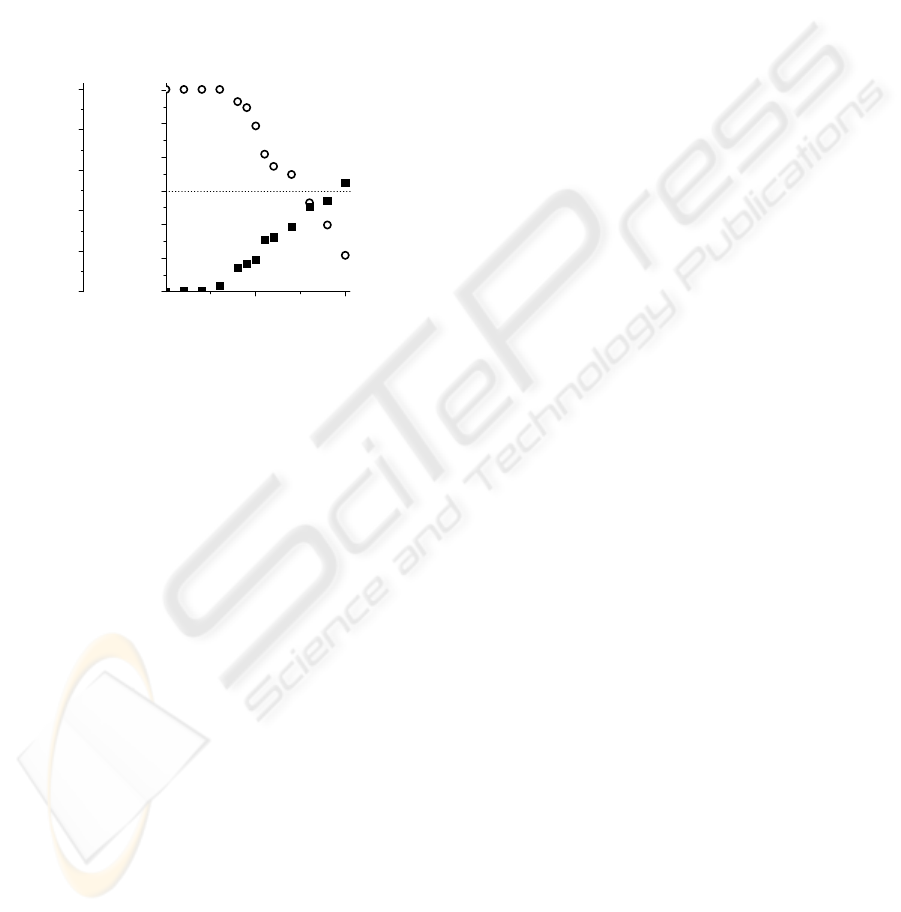
less than 1V (○), respectively. This figure shows
that not more than σ’’ =1.8μs is displayed below
V
w
=1.5V, and σ’’ =2.0μs is displayed above
V
w
=1.5V. The fluctuation of time shows that the
influence of reinforcement is displayed below σ’’
=1.8μs, and the influence of suppression appears at
σ’’ =2.0μs. As well, the rate at which V
w
becomes
not more than 1V is 100% within the range of σ’’
=0.6μs or less. This suggests a neural network with
STDP that has a learning function with tolerance for
the fluctuation of time of 0.6μs or less.
5 CONCLUSIONS
In this paper, we focus on STDP and we construct
neuro devices with STDP to study the effect of
STDP on the ability to extract phase differences.
Using these devices, we construct a neural network
that extracts phase difference information. As a
result, it is possible to extract the phase differences
of pulse-type neuro devices with STDP, representing
the reinforcement component of synaptic weight.
Moreover, we investigated the noise tolerance of the
proposed model. As a result, we demonstrated pulse-
type neuro devices with STDP that have a learning
function with tolerance for white noise of 0.8V or
less, and for fluctuation of time of 0.6μs or less.
That is to say, we showed that pulse-type neuro
devices with STDP had a learning function with
noise tolerance for the thermal noise and the
fluctuation of the time.
In our future work, we will construct an integrated
circuit with pulse-type neuro devices with STDP.
ACKNOWLEDGEMENTS
This work was supported in part by Amano Institute
of Technology.
REFERENCES
Hebb, D. O, 1949. “The organization of behavior, A
Neuropsychological Theory”, New York.
Bi G-q., Poo M-m., 1998. “Synaptic modifications in
cultured hippocampal neurons, Dependent on spike
timing, synaptic strength, and postsynaptic Cell Type”,
J. Neurosci.
Nishiyama M., Hong K., Mikoshiba K., Poo M-m., &
Kato K., 2000. “Calcium stores regulate the polarity
and input specificity of synaptic modification”,
Nature.
Patrick D. R., Curtis C. B., 2002. “Spike Timingdependent
synaptic plasticity in biological systems”, Bio.Cybern.
Sakai Y., and Yoshizawa S., 2003. “Mechanisms of
synaptic competition and regulation in spike-time-
dependent synaptic plasticity rules”, IEICE Technical
Report.
Tsukada M., Aihara T., Kobayashi Y., Shimazaki H., 2005.
“Spatial analysis of spike-timing-dependent LTP and
LTD in the CA1 area of hippocampal slices using
optional imaging”, Hippocampus.
Zhang li I., Tao HW., Holt CE., Harris WA., Poo M-m.,
1998. “A critical window for cooperation and
competition among developing retinotectal synapses”,
Nature.
Gerstner W, K, R., van Hemmen JL., Wagner H., 1996.
“A neuronal learning rule for sub-millisecond
temporal coding, Nature.
Fukai T. and Kanemura S., 2001. “Noise-tolerant stimulus
discrimination by synchronization with depressing
synapses”, Biological Cybernetics.
Saeki K., Hayashi Y.,, Sekine Y., 2006. “ Extraction of
Phase Information Buried in Fluctuation of a Pulse-
type Hardware Neuron Model Using STDP”, 2006
International Joint Conference on Neural Networks
(IJCNN2006).
B-i-P Adria and Murray A, F., 2004, “Synchrony
Detection and Amplification by Silicon Neurons With
STDP Synapses”, IEEE Trans. on Neural Networks.
Sekine Y., 1999. “Pulse-Type Hardware Neuron Model”,
Computer Today.
Saeki K., Sekine Y., and Aihara K., 1999,“A Study on a
Pulse-type Hardware Neuron Model using CMOS,”
International Symposium on Nonlinear Theory and Its
Applications (NOLTA99).
Sekine Y., Sumiyama M., Saeki, K. and Aihara K., 2001.
“ A Λ-Type Neuron Model using Enhancement-Mode
MOSFETs”, IEICE Trans.
1.0 2.0
0
20
40
60
80
100
0.0
0.5
1.0
1.5
2.0
2.5
3.0
ratio
V
W
σ’’ [
s]
Ratio that becomes V
W
less
than 1V [%]
V
W
[V]
Figure 8: Synaptic weight control voltage to fluctuation
of the time.
BIODEVICES 2008 - International Conference on Biomedical Electronics and Devices
268