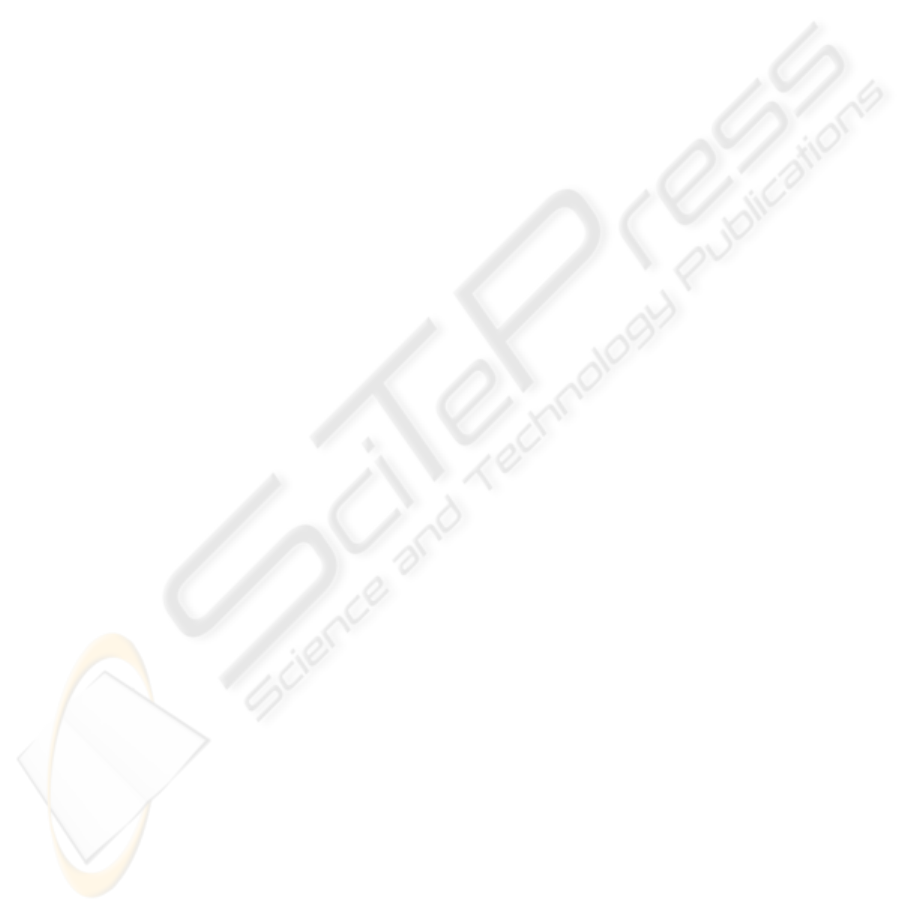
employing a small number of electrodes –ideally
two, or even a single, electrode(s). It may be
possible to obtain higher classification rates by
utilising a single electrode that is more relevant to
the specific mental task rather than using a
combination of electrodes, all of which are not as
relevant to the task. This is also advantageous as it
decreases the feature dimensionality.
5 CONCLUSIONS
This paper presents the results of initial
investigations in the search for appropriate features
and classifier towards the development of a thought-
to-speech converter. The results indicate that the use
of an SVM for the classification of AR coefficients
is more appropriate than LDA and NN and will be
utilised in the development of the proposed system.
The proposed system is promising as it offers the
ability to communicate more efficiently via direct
conversion of thoughts into speech. In order to
ensure optimal operation other aspects of the system
must also be investigated. Firstly, a more extensive
set of features and classifiers will be examined such
that the optimal combination in terms of maximising
accuracy is determined – computational efficiency is
not a consideration as the system will be customised
and capable of parallel processing. Secondly, these
investigations suggest that different combinations of
mental tasks seem to be more appropriate for
different subjects. We are going to look into finding
a combination of tasks that are more intuitive and
more closely related to the concept of MC, as this
could improve classification accuracy and facilitate
easier operation.
REFERENCES
Altschuler, E.L., and Dowla, F.U., 1998.
Encephalolexianalyzer. United States Patent Number:
5,840,040. November 24.
Burges, C. J. C., 1998. A tutorial on Support Vector
Machines for Pattern Recognition. In Data Mining and
Knowledge Discovery, U. Fayyad, Ed. Boston: Kluwer
Academic Publishers, pp. 121-167.
Coe, L., 2003. Telegraph: A History of Morse’s invention
and its predecessors in the United States. McFarland &
Company.
French, T., 1993. McElroy, World’s Champion Radio
Telegrapher. Artifax Books.
Guger, C., Schlogl, A., Neuper, C., Walterspacher, D.,
Strein, T., and Pfurtscheller, G., 2000. Rapid
Prototyping of an EEG-based Brain-Computer
Interface (BCI). In IEEE Trans. on Neural Systems
and Rehab. Eng., Vol. 9, issue 1, pp.49-58, March.
Gysels, E., and Celka, P., 2004. Phase synchronisation for
the recognition of mental tasks in a brain-computer
interface. In IEEE Trans. on Neural Systems and
Rehab. Eng., Vol. 12, issue 4, pp. 406-415.
Huan, N.-J., and Palaniappan, R., 2004. Neural network
classification of autoregressive features from
electroencephalogram signals for brain-computer
interface design. In Journal of Neural Eng., Vol. 1,
pp.142-150.
Keirn, Z.A., and Aunon, J.I., 1990. A new mode of
communication between man and his surroundings. In
IEEE Trans. Biomed. Eng.,, Vol. 37, pp.1209-1214.
Levine, S.P., Gauger, J.R.D., Bowers. L.D., and Khan,
K.J., 1986. A comparison of Mouthstick and Morse
code text inputs. In Augmentative and Alternative
Communication, Vol. 2, issue 2, pp.51-55.
Lopes da Silva, F., 1998. EEG analysis: Theory and
Practice. In Electroencephalography: Basic
Principles, Clinical Applications and Related Fields,
Ch. 6, pp.1135-1163.
Palaniappan, R., Paramesan, R., Nishida, S., and Saiwaki,
N., 2002. A new brain-computer interface design using
Fuzzy ARTMAP. In IEEE Trans. On Neural Systems
and Rehab. Eng., Vol. 10, issue 3, pp.140-148.
Palaniappan, R., 2005. Brain computer interface design
using band powers extracted during mental tasks. In
Procs. of the 2
nd I
nternational IEEE EMBS Conf. on
Neural Eng., Arlington, Virginia. March 16-19.
Park, H.-J., Kwon, S.-H., Kim, H.-C., and Park, K.-S.,
1999. Adaptive EMG-driven communication for the
disability. In Procs. of 1
st
Joint BMES/EMBS Conf.
Serving Humanity, Advancing Technology. Atlanta,
USA, October 13-19. p.656.
Scherer, R., Muller, G. R., Neuper, C., Graimann, B., and
Pfurtscheller, G., 2004. An Asynchronously controlled
EEG-based virtual keyboard: improvement of the
spelling rate. In IEEE Trans. on Biomed. Eng., Vol.
51, issue 6, pp.979-984.
Vaughan, T.M., et al., 2003. Brain-computer Interface
Technology: a review of the second international
meeting (Guest Editorial). In IEEE Trans. on Neural
Systems and Rehab. Eng., Vol. 11, issue 2, pp.94-109.
Wolpaw, J.R., et al., 2000. Brain-Computer Interface
Technology: a review of the First International
meeting. In IEEE Trans. on Rehab. Eng., Vol. 8. issue
2, pp.164-173.
Wolpaw, J.R., Birbaumer, N., McFarland, D.J.,
Pfurtscheller, G., and Vaughan, T.M., 2002. Brain-
Computer Interfaces for communication and control.
In Clinical Neurophysiology, Vol. 113, pp.767-791.
Wright, J.J., Kydd, R.R., and Sergejew, A.A., 1990.
Autoregression Models of EEG. In Biological
Cybernetics, Vol. 62, pp.201-210.
BIODEVICES 2008 - International Conference on Biomedical Electronics and Devices
16