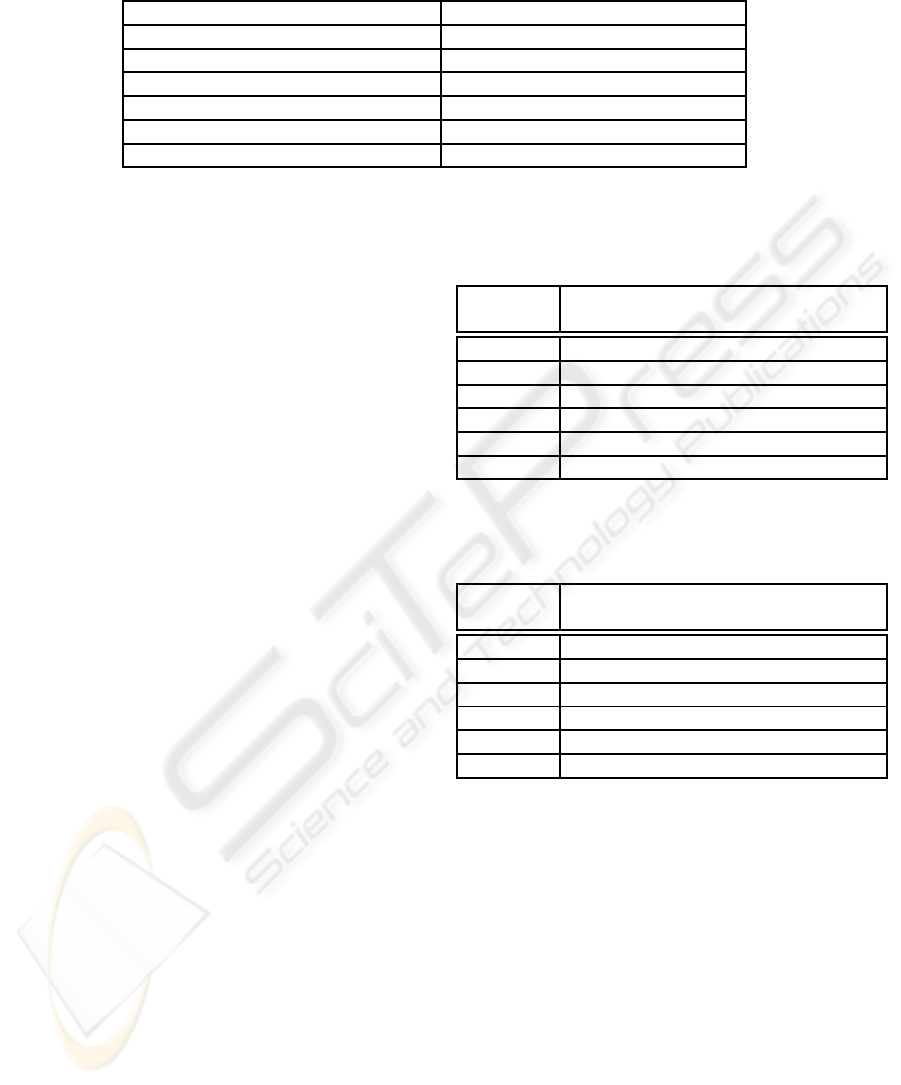
Table 3: Comparison of classification accuracy (%) for breast cancer dataset 1 (testing set) using NNCA-PS and different
classifiers, based on 100 experiments.
Algorithms Best (%) Average (%) Std (%)
PCA/MDC (Guo and Nandi, 2006) 88.7 88.6 N/A
FLDA/MDC (Guo and Nandi, 2006) 88.9 88.6 N/A
MLP (Guo and Nandi, 2006) 97.3 96.2 1.7
SVM (Guo and Nandi, 2006) 96.7 96.3 0.8
GP/MDC (Guo and Nandi, 2006) 98.9 97.4 1.5
NNCA-PS 99.5 97.2 1.2
ing; this process has been repeated 100 times. The tar-
get information, class labels, of the training samples
is used to guide the clustering process of the testing
samples using NNCA-PS algorithm. Table 3 shows
comparison results of NNCA-PS along with different
methods for classification. As shown, the best clas-
sification accuracy is achieved by NNCA-PS (99.5%),
with the lowest being 88.7% obtained by PCA/MDC
which gives comparable results as FLDA/MDC. Al-
though the average classification accuracy obtained
by GP/MDC are comparable with NNCA-PS, it gives
0.6% less than the best performance of NNCA-PS
with higher standard deviation in classification accu-
racy. Therefore, the proposed method is more robust
compared with other methods.
In order to reduce the amount of a priori knowl-
edge, a small number of objects from the entire
dataset are used as labelled objects. In these exper-
iments, the effect of the number of labelled objects on
the classification accuracy are investigated. We ran-
domly selected a fraction from the entire dataset to be
labelled objects. For each fraction, this process is re-
peated one hundred times without replacement. The
best, average, and standard deviation of classification
accuracy are obtained over one hundred runs for each
fraction of labelled objects. For breast cancer dataset
1, as demonstrated in Table 4, the best and average
classification accuracies increase with the increas-
ing fraction of the labelled objects. As shown, the
best and average classification accuracy of 98.2% and
96.3% respectively were achievedat 30% labelled ob-
jects, with the lowest being 96.2% and 91.5% for best
and average accuracies respectively at 5% labelled
objects. By examining the average and standard de-
viation of the classification performance, when 5%
of the entire dataset are labelled, the average perfor-
mance is the lowest, while it has the highest standard
deviation compared with the other fractions of la-
belled objects. For breast cancer dataset 2 as recorded
in Table 5, the standard deviations is lower than the
standard deviations of breast cancer dataset 1. It is
conjectured that the clusters on breast cancer dataset
2 are more compact with those in breast cancer dataset
Table 4: Classification accuracy (%) for breast cancer
dataset 1 (entire dataset) using NNCA with partial super-
vision (NNCA-PS), based on 100 experiments.
labelled
objects % Best (%) Average (%) Std (%)
5 96.2 91.5 2.3
10 96.3 93.1 1.8
15 97.0 94.4 1.3
20 97.2 95.3 1.0
25 97.6 95.6 0.9
30 98.2 96.3 0.7
Table 5: Classification accuracy (%) for breast cancer
dataset 2 (entire dataset) using NNCA with partial super-
vision (NNCA-PS), based on 100 experiments.
labelled
objects % Best (%) Average (%) Std (%)
5 98.0 96.0 1.2
10 98.1 96.3 1.1
15 98.5 96.7 0.9
20 98.7 97.0 0.8
25 98.7 97.4 0.7
30 99.2 97.9 0.5
1, as indicated in (Salem and Nandi, 2005). For 5%
labelled objects and higher, the best classification ac-
curacy is higher than 98% with a small decrease in the
standard deviation and a significant increase in the av-
erage classification accuracy as demonstrated in Table
5.
When comparing the proposed NNCA-PS with
RACAL for breast cancer data classification, where
a small number of objects from the entire dataset
are used as labelled objects. The average classi-
fication accuracy for breast cancer dataset 1 using
NNCA-PS is 1% higher than RACAL algorithm while
it achieves comparable accuracy for breast cancer
dataset 2 as demonstrated in Tables 6 and 7. More-
over, the standard deviation of the classification per-
formance of NNCA-PS for breast cancer dataset 1 is
lower than RACAL which favors compact clusters,
DEVELOPMENT OF A PARTIAL SUPERVISION STRATEGY TO AUGMENT A NEAREST NEIGHBOUR
CLUSTERING ALGORITHM FOR BIOMEDICAL DATA CLASSIFICATION
331