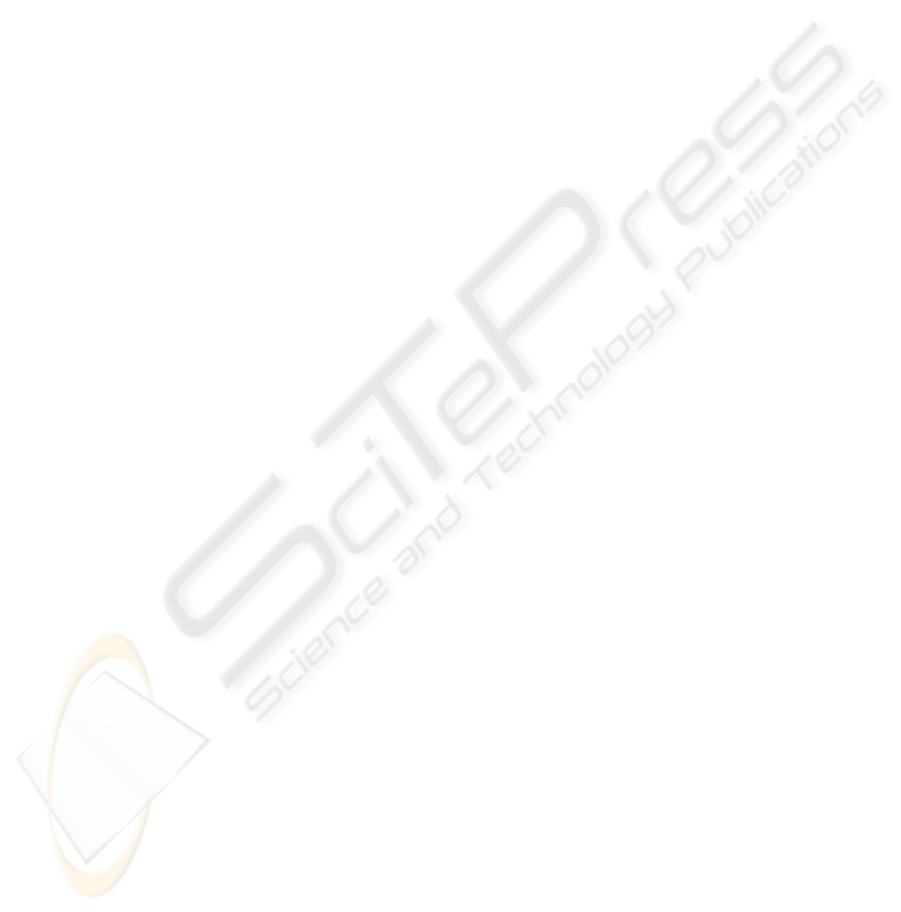
plitude threshold as ε = 0.01 and the window width as
λ = 0.0625 sec.
The algorithm output is composed by 32 chirps
which should correspond to the howls and barks (with
all their harmonics) emitted by the wolves along the
duration of the recording (about five sec). The result
is shown in Fig. 5. Since our aim is giving an estimate
of how many individuals are emitting in a recording,
we plot a zoom of the separating algorithm result for
the time interval (1, 2.5). Here, the number of chirps
reduces to six. However, it seems that one couple of
them are harmonics, the couples formed by the chirp
around 1000 Hz and the highest IF chirp. Therefore,
we may conclude that at least five wolves are emitting
in this interval of time. A similar analysis is carried
out with other time subintervals until all the recorded
signal is analyzed.
5 CONCLUSIONS
A combined algorithm for signal enhancement and
voice separation is utilized for wolf population count-
ing. Although field recorded wolf chorus signals
posses a complex structure due to noise corruption
and nonlinear multi-component character, the out-
come of our algorithm provides us with accurate es-
timates of the number of individuals emitting in a
given recording. Thus, the algorithm seems to be a
good complement or, even, an alternative to existent
methodologies, mainly based in wolf traces collection
or in the intrusive attaching of electronic devices to
the animals. Clearly, our algorithm is not limited to
wolves emissions but to any signal which may reason-
ably be modelled as an addition of chirps, opening its
utilization to other applications. Drawbacks of the al-
gorithm are related to the expert dependent election of
some parameters, such as the amplitude threshold, or
to the execution time when denoising and separation
of long duration signals must be accomplished. We
are currently working in the improvement of these as-
pects as well as in the recognition of components cor-
responding to the same emissor, such as harmonics of
a fundamental chirp, pursuing the full automatization
of the counting algorithm.
ACKNOWLEDGEMENTS
The first three authors are supported by Project
PC0448, Gobierno del Principado de Asturias, Spain.
Third and fourth authors are supported by the Spanish
DGI Project MTM2004-05417.
REFERENCES
Auger, F. (1991). Repr´esentation temps-fr´equence des sig-
naux non-stationnaires: Synthe`ese et contributions.
th`ese de doctorat, Ecole Centrale de Nantes.
B. Dugnol, C. Fern´andez, G. G. (2007a). Wolves counting
by spectrogram image processing. Appl. Math. Com-
put., 186:820–830.
B. Dugnol, C. Fern´andez, G. G. J. V. (2007b). Implementa-
tion of a chirplet transform method for separating and
counting wolf howls. Preprint 1, Dpt. Mathematics,
Univ. of Oviedo, Spain.
B. Dugnol, C. Fern´andez, G. G. J. V. (2007c). Implemen-
tation of a diffusive differential reassignment method
for signal enhancement. an application to wolf pop-
ulation counting. Appl. Math. Comput. To appear.
E-version: doi:10.1016/j.amc.2007.03.086.
B. Dugnol, C. Fern´andez, G. G. J. V. (2007d). On pde-
based spectrogram image restoration. application to
wolf chorus noise reduction and comparison with
other algorithms. In E. Damiani, A. Dipanda, K.
Y. L. L. P. S., editor, Signal processing for image
enhancement and multimedia processing, volume 34
of Multimedia systems and applications. Springer
Verlag. To appear. (e-version in http://www.u-
bourgogne.fr/SITIS/06/Proceedings/index.htm).
E. Chassandre-Mottin, I. Daubechies, F. A. P. F. (1997).
Differential reassignment. IEEE Signal Processing
Letters, 4(10):293–294.
F. Auger, P. F. (1995). Improving the readability of
time-frequency and time-scale representations by the
method of reassignment. IEEE Trans. Signal Process-
ing, 43(5):1068–1089.
H. M. Ozaktas, Z. Zalevsky, M. A. K. (2001). The Frac-
tional Fourier Transform with Applications in Optics
and Signal Processing. Wiley, Chichester.
K. Kodera, R. Gendrin, C. d. V. (1978). Analysis of time-
varying signals with small bt values. IEEE Trans-
actions on Acoustics, Speech and Signal Processing,
26(1):64–76.
L.
´
Alvarez, P. L. Lions, J. M. M. (1992). Image selective
smoothing and edge detection by nonlinear diffusion.
ii. SIAM J. Numer. Anal., 29(3):845–866.
L. Angrisani, M. D. (2002). A measurement method based
on a modified version of the chirplet transform for
instantaneous frequency estimation. IEEE Trans. In-
strum. Meas., 51:704–711.
L. Llaneza, V. P. Field recordings obtained in wilderness
in asturias (spain) in the 2003 campaign. Asesores en
Recursos Naturales, S.L.
Mallat, S. (1998). A wavelet tour of signal processing. Aca-
demic Press, London.
S. Mann, S. H. (1995). The chirplet transform: Physi-
cal considerations. IEEE Trans. Signal Processing,
43(11):2745–2761.
Skonhoft, A. (2006). The costs and benefits of ani-
mal predation: An analysis of scandinavian wolf re-
colonization. Ecological Economics, 58(4):830–841.
BIOSIGNALS 2008 - International Conference on Bio-inspired Systems and Signal Processing
84