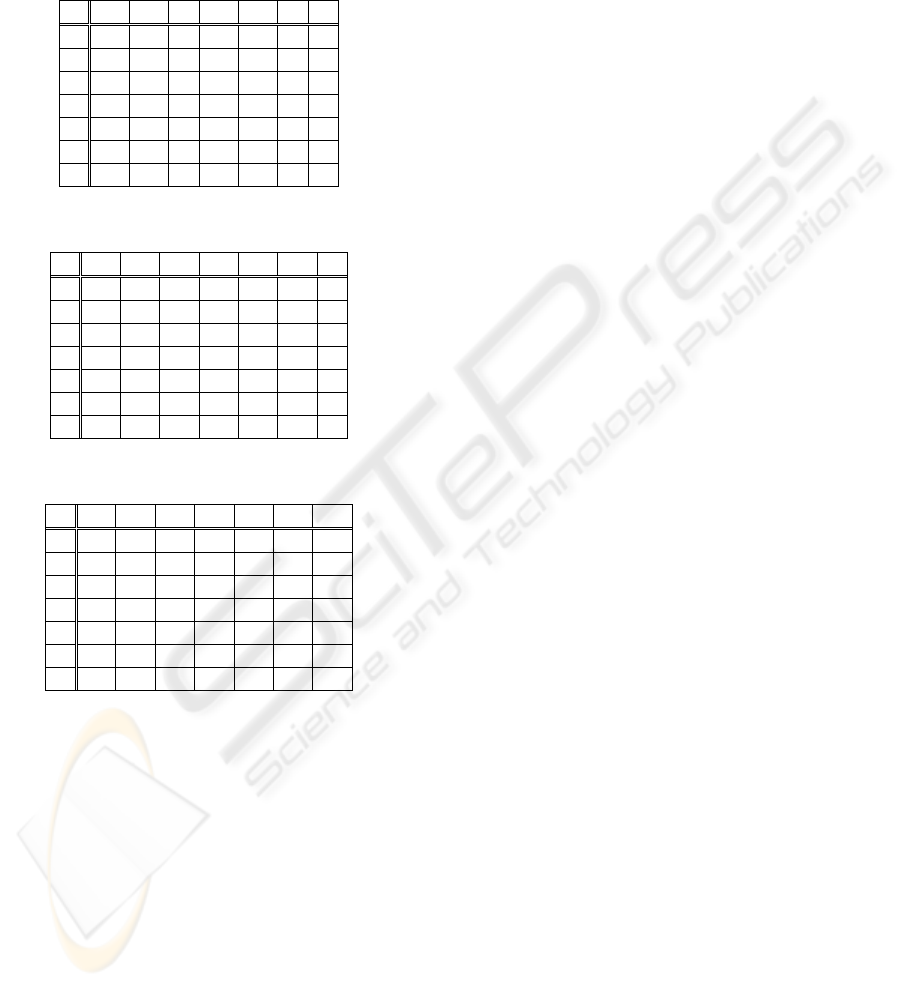
signals in the input feature vector was 2.46%. When
it is combined in the input vector, the features from
both the EMG and MMG signals, the classification
error decreased to 1.24%.
Table 5: Classification based on MMG signals.
1 2 3 4 5 6 7
1 9 0 2 0 0 0 0
2 1 9 2 0 1 0 0
3 3 4 9 0 0 0 0
4 0 0 0 10 1 0 2
5 0 0 0 0 10 1 0
6 0 0 0 0 0 7 1
7 0 0 0 0 0 2 7
Table 6: Classification based on EMG signals.
1 2 3 4 5 6 7
1 13 0 1 0 0 0 0
2 0 13 0 0 0 0 0
3 0 0 12 0 0 0 1
4 0 0 0 10 0 0 0
5 0 0 0 0 12 0 0
6 0 0 0 0 0 10 0
7 0 0 0 0 0 0 9
Table 7: Classification based on MMG and EMG signals.
1 2 3 4 5 6 7
1 13 0 1 0 0 0 0
2 0 13 0 0 0 0 0
3 0 0 12 0 0 0 0
4 0 0 0 10 0 0 0
5 0 0 0 0 12 0 0
6 0 0 0 0 0 10 0
7 0 0 0 0 0 0 10
5 CONCLUSIONS
The results obtained during the experiment imply
that efficient identifying hand movements based
only on one MMG sensor is very difficult.
Especially the first three movements are being
confused during the identification process. The
reason for such error is because those movements
are caused by similar muscles and therefore sounds
propagating during those movements are much alike.
The EMG based identification system gives
much greater accuracy. The neural network taught
with EMG based data badly recognizes only a small
percent of test examples. Using the information
obtained from both mechanomyogram and
electromyogram improves results of the EMG-based
recognition. Therefore it can be concluded that the
mechanomyographic sensors can be used as a
enhancement to a EMG prosthesis system improving
the accuracy of identification and count of the
supported range of movements. LVQ network
proved produced sufficient and satisfactory
recognition ratio, therefore proving its usefulness in
the biosignal-based prosthesis control problem.
Further improvement could be achieved by applying
more complex neural network architectures in the
recognition process and also by modifying the
feature extraction algorithm. Those are the key
areas for future investigation of the problem.
REFERENCES
Orizio, C., 1993, Muscle sound: Bases for the introduction
of a Mechanomyographic signal in muscle studies,
Critical Reviews in Biomedical Engineering, 21(3),
pp. 201-243.
Asres, A., Dou, H. F., Zhou, Z. Y., Zhang, Y. L., Zhu, S.
C., 1996, A combination of AR and neural network
technique for EMG pattern identification, Proc. Ann.
Int. Conference IEEE Eng. Med. Biol. Soc., vol. 4, pp.
1464-1465.
Grossman, A., Silva, J., and Chau, T., 2004, Functional
Mapping of Multiple Mechanomyographic Signals to
Hand Kinematics, Canadian Conference on Electrical
and Computer Engineering, vol. 1, pp. 493-496.
Wołczowski, A., 2001, Smart Hand: The Concept of
Sensor based Control, Proc. of 7th IEEE Int. Symp. on
‘Methods and Models in Automation and Robotics’,
Międzyzdroje.
Goldenberg, M. S., Yack, H. J., Cerny F. J., and Burton,
H. W., 1991, Acoustic myography as an indicator of
force during sustained contractions of a small hand
muscle, J. Appl. Physiol., vol. 70, nº. 1, pp. 87-91.
Silva, J., Heim, W., and Chau, T., 2004, MMG-Based
Classification of Muscle Activity for Prosthesis
Control, Proc. 26
th
Annual Int. Conference of the
IEEE Engineering in Medicine and Biology Society,
vol. 2, pp. 968-971.
Tarata, M. T., 2003, Mechanomyography versus
Electromyography, in monitoring the muscular
fatigue, BioMedical Engineering Online, 2:3.
Ouamer, M., Boiteux, M., Petitjean, M., Travens, L., and
Sal’es, A., 1999, Acoustic myography during
voluntary isometric contraction reveals non-
propagative lateral vibrations, J. Biomech., vol. 32,
nº. 12, pp. 1279-1285.
Krysztoforski, K., Wołczowski A., 2005, Measurement
stand for recording EMG signals . Adv. of Robotics:
Industrial and medical robotic systems, WKL,
Warsaw.
Kohonen, Teuvo K., 1995, Self-Organizing Maps,
Springer, Berlin.
IDENTIFICATION OF HAND MOVEMENTS BASED ON MMG AND EMG SIGNALS
539