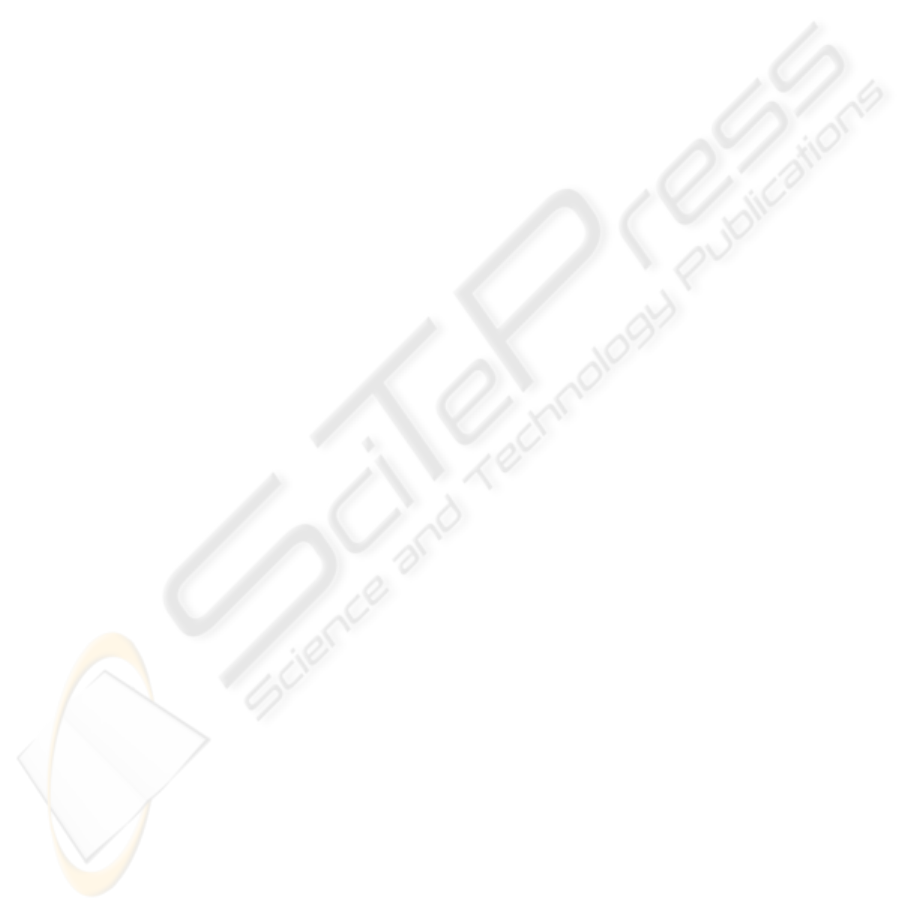
The exact and objective information about the ex-
amination from particular date can especially help in
longer time diagnostics when repeating of the exami-
nation is no longer possible.
Our method may be divided into two phases. At
first, it attempts to correctly identify the position of
brain stem in processed image. This phase is crucial
in overall diagnostics and this paper focuses mostly
on this part. In the second phase, we detect the ob-
jects of interest in the brain stem. The detection of
existence, shape, size, and echogenicity of these ob-
jects is a valuable contribution to the diagnostics of
Parkinson’s disease.
Achieved results obtained during testing make us
believe that the method we have developed for the de-
tection and analysis of the brain stem in transcranial
ultrasound images is successful. From the tested im-
ages, we obtained good results. In 76% of cases, the
position of the brain stem was correctly determined.
ACKNOWLEDGEMENTS
Presented results had been obtained during solving
the grant project code T401940412 supported by the
Academy of Sciences of the Czech Republic.
REFERENCES
Ballard, D.H., B. C. (1982). Computer vision. Prentice-
Hall, Englewood Cliffs, NJ.
Becker, G., B. U. G. C. e. a. (1995). Transcranial color-
coded real-time sonography of intracranial veins. nor-
mal values of blood flow velocities and findings in
superior sagittal sinus thrombosis. Journal of Neu-
roimaging, 5:87–94.
Berg, D., B. G. Z. B. T. O. H. E. e. a. (1999). Vulnerability
of the nigrostriatal system as detected by transcranial
ultrasound. Neurology, 53:1026–1031.
Berg, D., S. C. B. G. (2001). Echogenicity of the substantia
nigra in parkinson’s disease and its relation to clinical
findings. Journal of Neurology, 248:684–689.
Binder, T., S. M. M. D. S. H. B. T. M. G. P. G. (1999). Ar-
tificial neural networks and spatial temporal contour
linking for automated endocardial contour detection
on echocardiograms: A novel approach to determine
left ventricular contractile function. 25(7):1069–1076.
Bogdahn, U., B. G. S. F. (1998). Echoenhancers and tran-
scranial color duplex sonography. Blackwell Science,
Berlin.
Bosch, J.G., M. S. L. B. N. F. K. O. S. M. R. J. (2002). Au-
tomatic segmentation of echocardiographic sequences
by active appearance motion models. 21(11):1374–
1383.
Boukerroui, D., B. D. N. J. B. O. (2003). Segmentation
of ultrasound images - multiresolution 2d and 3d al-
gorithm based on global and local statistics. Pattern
Recognition Letters, 24(4-5):779–790.
Heitz, F., P. P. B. P. (1994). Multiscale minimization of
global energy functions in some visual recovery prob-
lems. 59:125–134.
Kerr, A.T., P. M. F. F. H. J. (1986). Speckle reduction in
pulse echo imaging using phase insensitive and phase
sensitive signal processing techniques. 8:11–28.
Klinger, J.W.J., V. C. F. T. A. L. T. (1988). Segmentation
of echocardiographic images using mathematical mor-
phology. 35(11):925–934.
Lee, J. (1980). Digital image enhancement and noise ltering
by use of local statistics. PAMI-2(2):165–168.
Lin, N., Y. W. D. J. (2003). Combinative multi-scale level
set framework for echocardiographic image segmen-
tation. 7(4):529–537.
Magnin, P.A., v. R. O. T. F. (1982). Frequency compound-
ing for speckle contrast reduction in phased array im-
ages. Ultrasonic Imaging, 4:267–281.
Mignotte, M., M. J. (2001). A multiscale optimization ap-
proach for the dynamic contour-based boundary de-
tection issue. 25(3):265–275.
Mishra, A., D. P. G. M. K. (2006). A ga based approach for
boundary detection of left ventricle with echocardio-
graphic image sequences. 21(11):967–976.
Noble, J.A., B. D. (2006). Ultrasound image segmentation:
A survey. IEEE Transactions on medical imagining,
25(8):987–1010.
Rakotomamonjy, A., D. P. M. P. (2000). Wavelet-based
speckle noise reduction in ultrasound b-scan images.
22:73–94.
Rekeczky, C., T. A. V. Z. R. T. (1999). Cnn-based spa-
tiotemporal nonlinear filtering and endocardial bound-
ary detection in echocardiography. 27(1):171–207.
Ressner, P., v. D. H. P. K. P. (2007). Hyperechogenicity of
the substantia nigra in parkinson’s disease. Journal of
Neuroimaging, 17(Issue 2):164–167.
Sattar, F., F. L. S. G. L. B. (1997). Image enhancement
based on nonlinear multi-scale method. 6:888–895.
Sojka, E. (2006). A motion estimation method based on
possibility theory. In Proceedings of IEEE ICIP, pages
1241–1244.
ˇ
Skoloud
´
ık, D., F. T. B. P. L. K. R. P. Z. O. H. P. H. R. K. P.
(2007). Reproducibility of sonographic measurement
of the substantia nigra. Ultrasound in Medicine & Bi-
ology, 33(9):1347–1352.
Yan, J.Y., Z. T. (2003). Applying improved fast marching
method to endocardial boundary detection in echocar-
diographic images. 24(15):2777–2784.
A NEW METHOD FOR DETECTION OF BRAIN STEM IN TRANSCRANIAL ULTRASOUND IMAGES
483