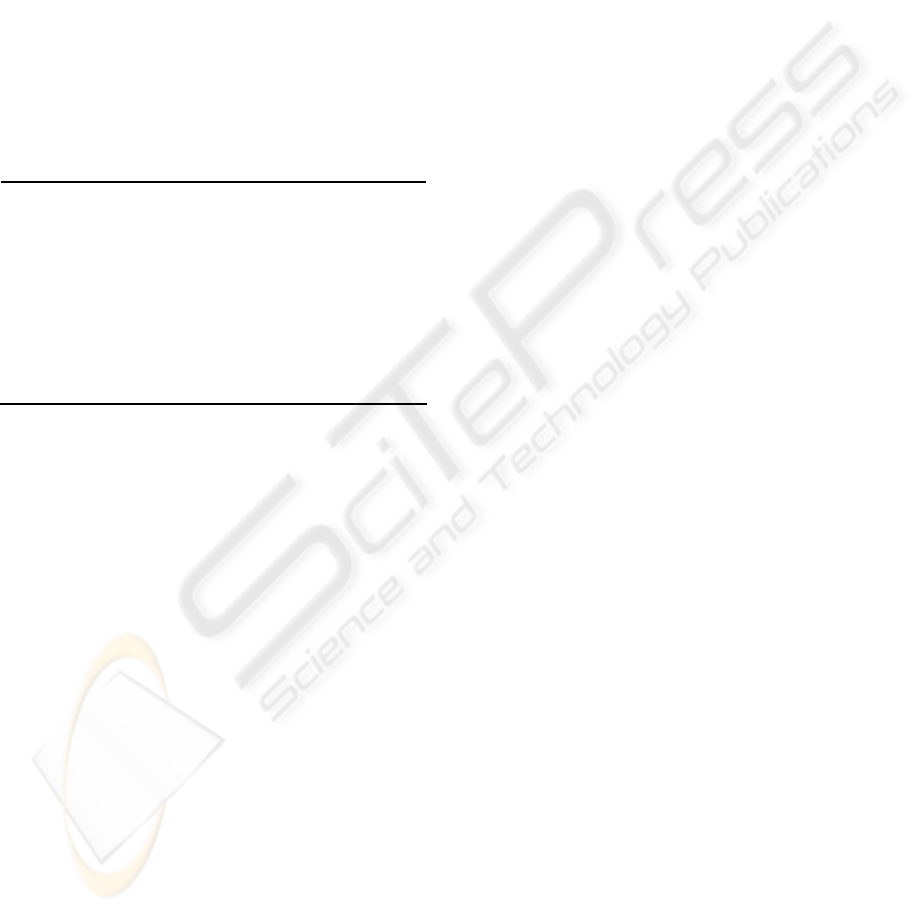
to the sum of entropy and Chen’s fractal dimension
for left upper/down respect of right upper/down
quarter of brain was calculated. Entropy results
confirmed the medical observations (Table 2).
Graphs of entropy and Chen’s fractal dimension for
the higher level of pixel intensity (225-256) in
regions of brain for which the differences of these
measures between both hemispheres are bigger than
10% are showed on Fig. 3. Histograms of entropy
and fractal dimension (Fig.4) show significant
differences for the higher level of pixel intensity.
Table 2: Number of slices for which the rate of the
difference to the sum of S or Df in the left and right
hemispheres in range with the biggest intensity of pixels
(from 193 to 256) has values bigger than 0.1 for four
regions of brain (UL-upper left, UR-upper right, DL-down
left, DR-down right).
Pacjent
name
Number of slices
for entropy
UL-UR-DL-DR
Slices number
for fractal
dimension
UL-UR-DL-DR
CHM 1-7-7-0 1-1-0-4
KOS 3-7-8-0 6-1-1-3
SIE 0-9-3-5 1-0-0-0
SZY 10-0-9-0 0-0-0-0
TWO 3-3-2-0 1-0-0-5
ZIE 1-7-4-2 1-1-0-0
5 CONCLUSIONS
The above presented methods of cerebral SPECT
images analysis based on simple image processing
methods and calculation of basic statistical
parameters are effective tools for a preliminary
assessment of cerebral perfusion in diagnosis of
epileptic and/or cerebral ischemic patients. It was
found that for reduced perfusion entropy increases
and Chen’s fractal dimension decreases. Entropy
based on the intensity histograms permits on
automatic perfusion asymmetry evaluation between
left and right brain hemisphere taking into account
only the bigger intensities of pixels (in the range
from 193 to 256). Entropy is a better measure to
estimate the global intensity however without
information about spatial distribution. For
identification of epileptic seizure localization
(concentration of high intensity pixels) Chen’s
fractal dimension seems to be the better measure.In
further work calculations for more patients and for
group of healthy volunteers should be done. Chen’s
fractal dimension could be calculated for less-
dimensional matrices (8 x 8) in sliding window to
construct map of fractal dimension of the whole
brain. It will allow to estimate better the utility of
this method to localize the epileptic seizure and to
compare different regions of interest (ROIs).
ACKNOWLEDGEMENTS
We acknowledge thanks to prof. Leszek Królicki
and dr Adam Bajera from the Department of Nuclear
Medicine of the Medical Academy of Warsaw for
providing databases – the SPECT images registered
for epileptic patients.
This work was supported by Institute of
Biocybernetics and Biomedical Engineering Polish
Academy of Sciences under Grant St/18/07 and
ST/21/07.
REFERENCES
Chen C., Daponte J., Fox M., 1989. Fractal feature
analysis and classification in medical imaging. IEEE
Trans. Med.. Imaging, 8, 133-142.
Kuczyński K., Mikołajczyk P., 2003. Dopasowanie i
segmentacja obrazów medycznych w oparciu o
elementy teorii informacji (in polish). In XIII Krajowa
Konferencja Naukowa Biocybernetyka i Inżynieria
Biomedyczna, Gdańsk, pp. 806-811.
Oczeretko E.S., 2006. Wymiar fraktalny w analizie
sygnałów i obrazów biomedycznych (in polish),
Wydawnictwo Uniwersytetu w Białymstoku,
Białystok.
Shannon C.E., 1948. A Mathematical Theory of
communication. The Bell System Technical Journal,
27, 379-423, 623-659.
Prószyński B., 2006. Radiologia obrazowa RTG, TK,
USG, MR i radioizotopy (in polish), PZWL,
Warszawa.
Russ J.C., 1995. Image processing handbook. 2
nd
edition.
CRC Press, Boca Raton, Ann Arbor, London.
ANALYSIS OF DIFFERENCES BETWEEN SPECT IMAGES OF THE LEFT AND RIGHT CEREBRAL
HEMISPHERES IN PATIENTS WITH EPILEPTIC SYMPTOMS
211