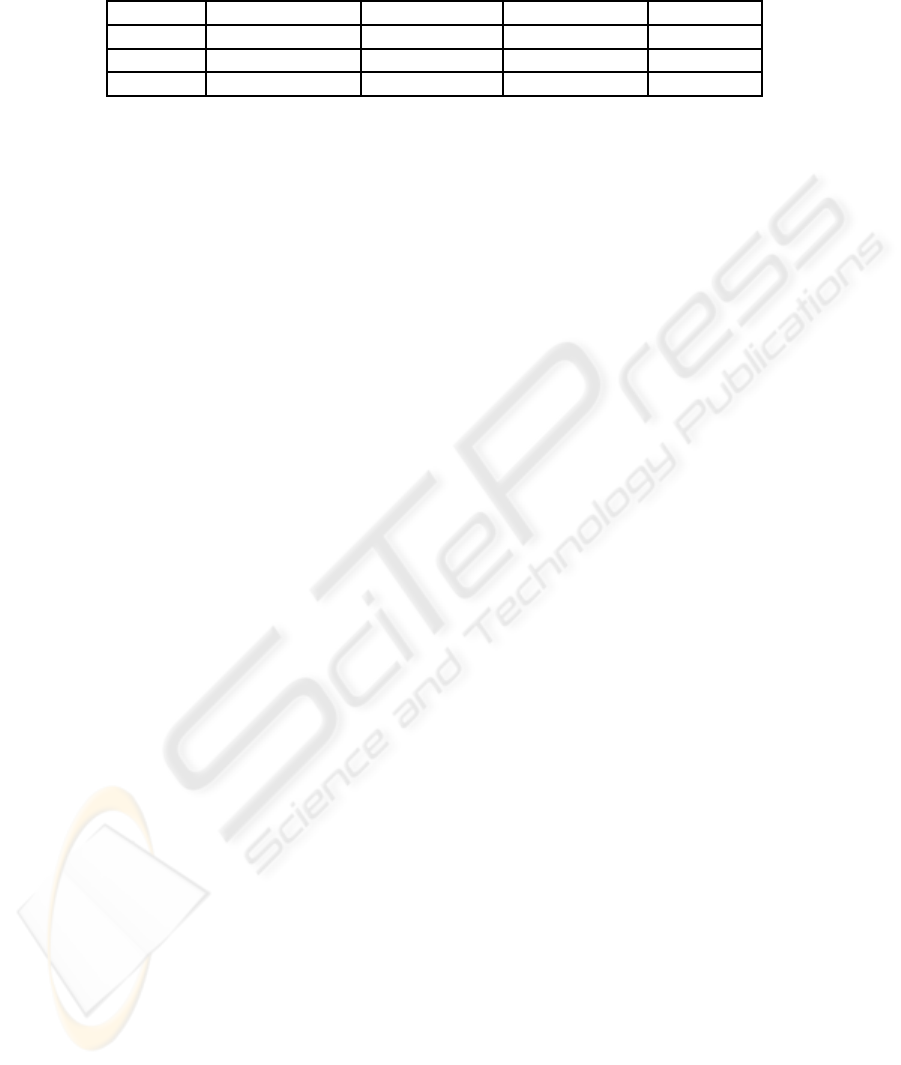
Table 2: The best classification accuracy (%) using original features, one KPCA-extracted features, one KGDA-extracted
features and one GP-generated features respectively, with a MLP, a KNN and a MDC classifier respectively on breast cancer
dataset.
Classifier Original Feature KPCA Feature KGDA Feature GP Feature
MLP 97% 90% 93.5% 98.5%
KNN 87.5% 85.5% 93% 98.5%
MDC 84% 94.5% 93.5% 98.5%
7 CONCLUSIONS
It is now clear from Figure 4 that values of the single
feature obtained from our proposed method cluster
naturally into largely non-overlapping groups. Thus
no computationally complex classifier may be needed
for successful classification, instead some simple
thresholds are enough. Summarizing all the results
obtained from different approaches for breast cancer
diagnosis problem, it can be said that performances
from a single GP-generated feature are the most accu-
rate and reliable in all experiments. From the results
of different pattern recognition problems, GP is not
only capable of reducing the dimensionality, but also
achieving a significant improvement in the classifica-
tion accuracy. Using the single feature generated by
GP makes a significant contribution to the improve-
ment in classification accuracy and robustness, com-
pared with other sets of features extracted by KPCA
and KGDA.
Generally in pattern recognition problems, there is
a reliance on the classifier to find the discriminating
information from a large feature set in case of stand-
alone MLP. In this paper, GP as a machine learning
method is proposed for nonlinear feature extraction
for breast cancer diagnosis. This approach is able
to learn directly from the data just like conventional
methods (such as FLDA and PCA), but in an evolu-
tionary process. Under this framework, an effective
feature can be formed for pattern recognition prob-
lems without the knowledge of probabilistic distribu-
tion of data.
From the experimental results it can be seen that
with the combination of a simple form of classifier
MDC, GP outperforms the other two feature extrac-
tors which are using more sophisticate classifier MLP,
indicating an overwhelming advantage of GP in fea-
ture extraction for breast cancer diagnosis.
ACKNOWLEDGEMENTS
H. Guo would like to acknowledge the financial sup-
port of the Overseas Research Studentship Commit-
tee, UK, the University of Liverpool and the Univer-
sity of Liverpool Graduates Association (HK)
REFERENCES
Cancer research UK.
Benyahia, I. and Potvin, J. (1998). Decision support for
vehicle dispatching using genetic programming. IEEE
Trans. Syst., Man, Cybern. Part.A, 28(3):306–314.
Brameier, M. and Banzhaf, W. (2001). A comparison of
linear genetic programming and neural networks in
medical data mining. IEEE Trans. on Evolutionary
Computation, 5(1):17–26.
D.J. Newman, S. Hettich, C. B. and Merz, C. (1998). UCI
repository of machine learning databases.
E.Osuna, Freund, R., and Girosi, F. (1997). Support Vec-
tor Machines: Training and Applications. MIT, Tech.
Rep.
Guo, H. and Nandi, A. K. (2006). Breast cancer diagnosis
using genetic programming generated feature. Pattern
Recognition, 39(5):980–987.
Jain, R. and Abraham, A. (2004). A comparative study
of fuzzy classification methods on breast cancer data.
Australas. Physical Engineering Sciences Medicine,
27(4):213–218.
Kermani, B. G., White, M. W., and Nagle, H. T. (1995).
feature extraction by genetic algorithms for nerual net-
works in breast cancer classification. volume 1, pages
831–832. New York, USA.
Kishore, J. K., Patnaik, L. M., Mani, V., and Arawal, V. K.
(2000). Application of genetic programming for mul-
ticategory pattern classification. IEEE Trans. on Evo-
lutionary Computation, 4(3):242–258.
Kotani, M., Ozawa, S., Nasak, M., and K.Akazawa (1997).
Emergence of feature extraction function using ge-
netic programming. In Knowledge-Based Intelligent
Information Engineering Systems, Third International
Conference, pages 149–152.
Koza, J. R. (1992). Genetic Programming: On the Pro-
gramming of Computers by Means of Natural Selec-
tion. MIT Press, Cambridge.
Muller, K. R., Mika, S., Ratsch, G., Tsuda, K., and
Scholkopf, B. (2001). An introduction to kernel-based
learning algorithms. IEEE Trans. on Neural Networks,
12(2):181–201.
BIOSIGNALS 2008 - International Conference on Bio-inspired Systems and Signal Processing
340