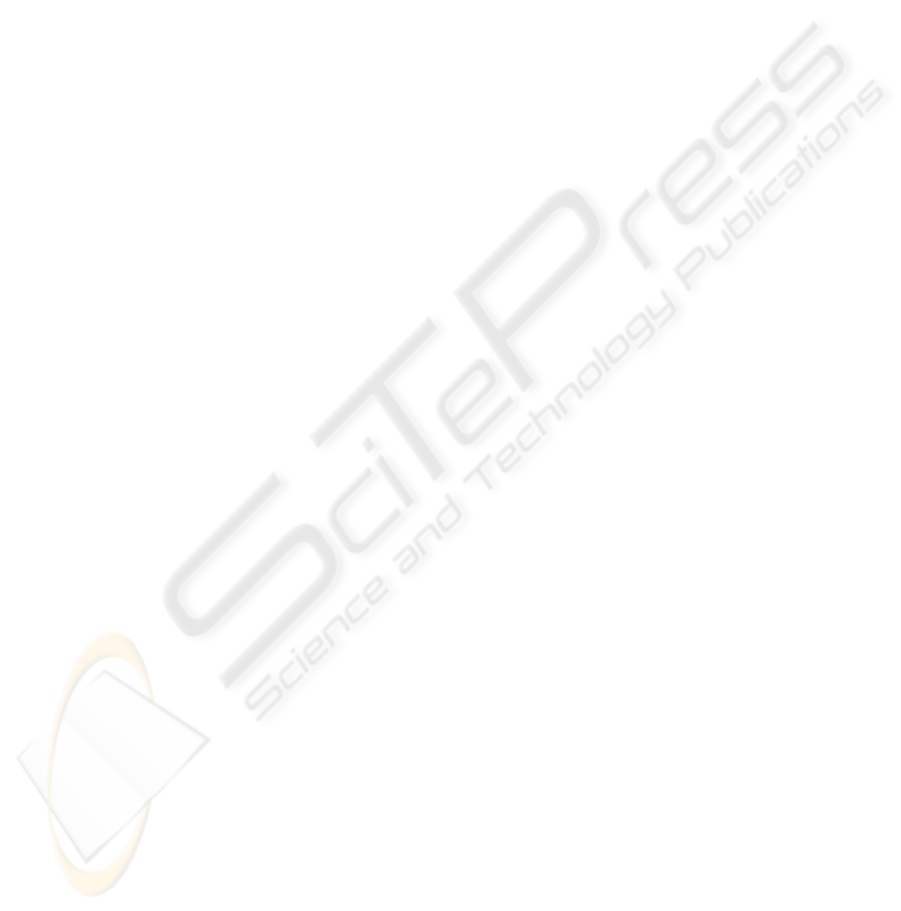
function method in pattern recognition learning.
Automation and Remote Control, 25:821–837.
Boser, B. E., Guyon, I. M., and Vapnik, V. N. (1992).
A training algorithm for optimal margin classi-
fiers. In Proc. of the 5th Annual ACM Workshop
on Computational Learning Theory, pages 144–
152.
Canu, S., Grandvalet, Y., and Rakotomam, A. (2003).
SVM and Kernel Methods Matlab Toolbox. Per-
ception Systems et Information, INSA de Rouen,
Rouen, France.
Cortes, C. and Vapnik, V. (1995). Support-vector net-
works. Machine Learning, 20(3):273–297.
Fisher, R. A. (1936). The use of multiple measure-
ments in taxonomic problems. Annals of Eugen-
ics, 7(2):179–188.
Guo, H. and Nandi, A. K. (2006). Breast cancer diag-
nosis using genetic programming generated fea-
ture. Pattern Recognition, 39:980–987.
Lam, W., Keung, C., and Ling, C. X. (2002). Learning
good prototypes for classification using filtering
and abstraction of instances. Pattern Recogni-
tion, 35(7):1491–1506.
Mandelbrot, B. B. (1997). The Fractal Geometry of
Nature, Chapter 5. W. H. Freeman and Com-
pany, New York.
Mangasarian, O. L., Street, W. N., and Wolberg, W. H.
(1995). Breast cancer diagnosis and prognosis
via linear programming. Operations Research,
43(4):570–577.
Mangasarian, O. L. and Wild, E. W. (2006). Multisur-
face proximal support vector machine classifica-
tion via generalized eigenvalues. IEEE Trans-
actions on Pattern Analysis and Machine Intelli-
gence, 28:69–74.
Marshall, E. (1993). Search for a killer: Focus shifts
from fat to hormones in special report on breast
cancer. Science, 259:618–621.
Mika, S., R
¨
atsch, G., Weston, J., Sch
¨
olkopf, B., and
Muller, K. (1999). Fisher discriminant analysis
with kernels. In Proc. of IEEE Neural Networks
for Signal Processing Workshop, pages 41–48.
Mu, T. and Nandi, A. K. (2007). Breast cancer de-
tection from FNA using SVM with different pa-
rameter tuning systems and SOM–RBF classi-
fier. Journal of the Franklin Institute, 344(3-
4):285–311.
Mu, T., Nandi, A. K., and Rangayyan, R. M. (2007a).
Pairwise Rayleigh quotient classifier with appli-
cation to the analysis of breast tumors. In Proc.
of the 4th IASTED Int’l Conf. on Signal Process-
ing, Pattern Recognition, and Applications, SP-
PRA, pages 356–361, Innsbruck, Austria.
Mu, T., Nandi, A. K., and Rangayyan, R. M. (2007b).
Strict 2-surface proximal classifier with applica-
tion to breast cancer detection in mammograms.
In Proc. of the 32nd Int’l Conf. on Acoustics,
Speech, and Signal Processing, ICASSP, vol-
ume 2, pages 477–480, Honolulu, HI.
Sch
¨
olkopf, B., Smola, A. J., Williamson, R., and
Bartlett, P. (2000). New support vector algo-
rithms. Neural Computation, 12:1207–1245.
Shawe-Taylor, J. and Cristianini, N. (2004). Kernel
Methods for Pattern Analysis. Cambridge Uni-
versity Press, Cambridge, UK.
Street, W. N., Mangasarian, O. L., and Wolberg, W. H.
(1995). An inductive learning approach to prog-
nostic prediction. In Proc. of the 12th Int’l Conf.
on Machine Learning, ICML, pages 522–530,
Morgan Kaufmann.
Street, W. N., Wolberg, W. H., and Mangasarian, O. L.
(1993). Nuclear feature extraction for breast tu-
mor diagnosis. In Proc. of IST/SPIE Symposium
on Electronic Imaging: Science and Technology,
volume 1905, pages 861–870, San Jose, CA.
Wolberg, W. H., Street, W. N., and Mangasarian, O. L.
(1993). Breast cytology diagnosis via digital im-
age analysis. Analytical and Quantitative Cytol-
ogy and Histology, 15(6):396–404.
Wolberg, W. H., Street, W. N., and Mangasarian, O. L.
(1994). Machine learning techniques to diagnose
breast cancer from fine-needle aspirates. Cancer
Letter, 77:163–171.
Wolberg, W. H., Street, W. N., and Mangasarian, O. L.
(1995). Image analysis and machine learning ap-
plied to breast cancer diagnosis and prognosis.
Analytical and Quantitative Cytology and His-
tology, 17(2):77–87.
BIOSIGNALS 2008 - International Conference on Bio-inspired Systems and Signal Processing
348