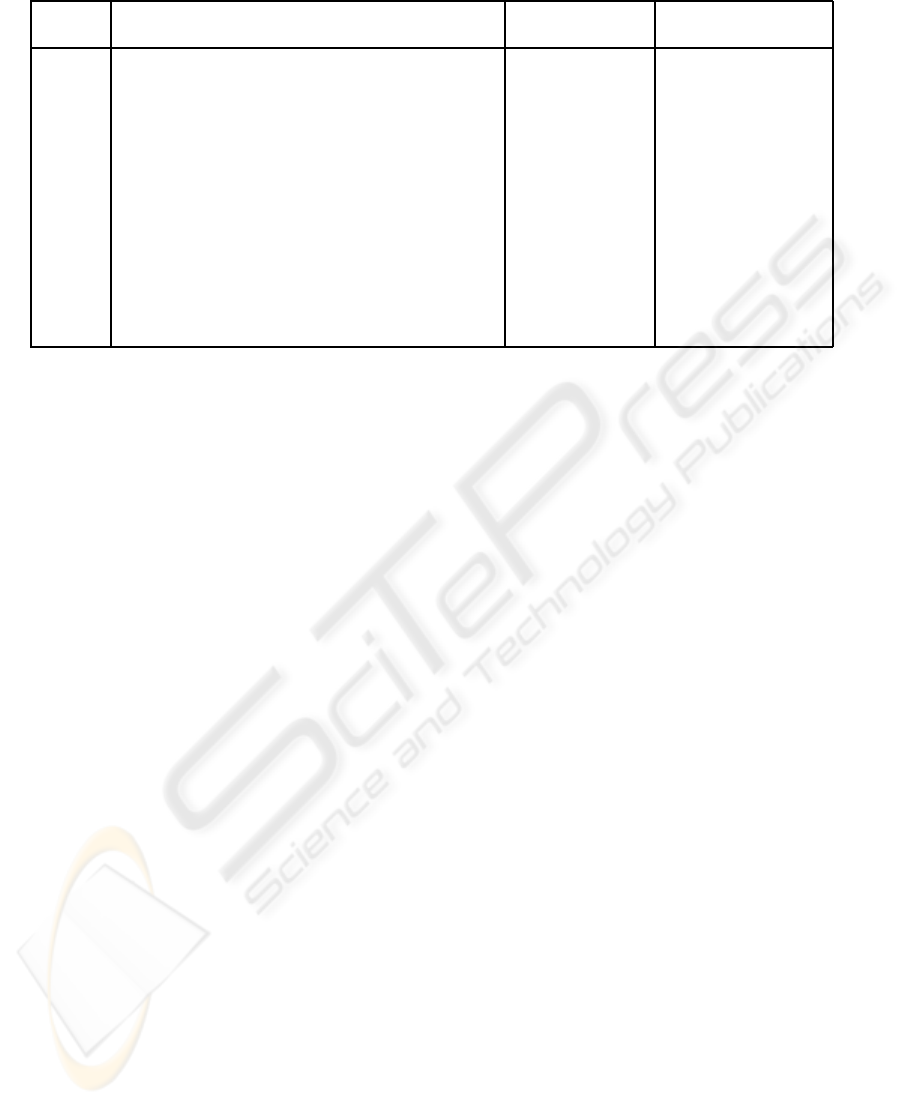
Table 2: Manually measured widths for an image cross-sections.
Cross- Manually measured width (in Micron) Mean width (µ) Standard Deviation
section One Two Three Four Five (in pixel) (σ
m
)
1 112.42 117.53 107.31 117.53 112.42 22.2 0.8366
2 107.31 112.42 107.31 117.53 107.31 21.6 0.8944
3 66.43 76.65 61.32 71.54 61.32 13.2 1.3088
4 61.32 71.54 61.32 71.54 56.21 12.6 1.3416
5 56.21 66.43 56.21 66.43 66.43 12.2 1.0954
6 107.31 107.31 102.2 102.2 97.09 20.2 0.8366
7 56.21 66.43 45.99 61.32 66.43 11.6 1.6733
8 86.87 107.31 102.2 107.31 97.09 19.6 1.6733
9 132.86 127.75 112.42 132.86 107.31 24 2.3452
10 45.99 51.1 35.77 56.21 35.77 8.8 1.7889
11 40.88 56.21 35.77 45.99 45.99 8.8 1.4832
12 35.77 51.1 45.99 56.21 40.88 9 1.5811
13 35.77 45.99 35.77 45.99 30.66 7.6 1.3416
rowing, branching coefficients, etc.) for diagnosing
various diseases. Currently, we are working on the
blood vessels’ bifurcation and cross-over detection
where the measured width is contributing as an im-
portant information for perceptual grouping process.
ACKNOWLEDGEMENTS
We would like to thank David Griffiths (Research As-
sistant, The University of Melbourne and Eye and Ear
Hospital, Melbourne, Australia) for providing us with
the manually measured width images and data.
REFERENCES
Bezdek, J. (1981). Pattern recognition with fuzzy objective
function algorithms. Plenum Press, USA.
Bhuiyan, A., Nath, B., and Chua, J. (2007a). An adaptive
region growing segmentation for blood vessel detec-
tion from retinal images. Second International Con-
ference on Computer Vision Theory and Applications,
pages 404–409.
Bhuiyan, A., Nath, B., Chua, J., and Kotagiri, R. (2007b).
Blood vessel segmentation from color retinal images
using unsupervised classification. In the proceedings
of the IEEE International Conference of Image Pro-
cessing.
Brinchman-hansan, O. and Heier, H. (1986). Theoritical re-
lations between light streak characterstics and optical
properties of retinal vessels. Acta Ophthalmologica,
179(33).
DRIVE-database (2004). http://www.isi.uu.nl/research/
databases/drive/, image sciences institute, university
medical center utrecht, the netherlands.
Gao, X., Bharath, A., Stanton, A., Hughes, A., Chapman,
N., and Thom, S. (2001). Measurement of vessel
diameters on retinal images for cardiovascular stud-
ies. Proceedings of Medical Image Understanding
and Analysis, pages 1–4.
Geusebroek, J., Boomgaard, R. V. D., Smeulders, A. W. M.,
and Geerts, H. (2001). Color invariance. IEEE Trans-
actions on Pattern Analysis and Machine Intelligence,
23(2):1338–1350.
Gonzalez, R. C., Woods, R. E., and Eddins, S. L. (2004).
Digital image processing using matlab. Prentice Hall.
Hoover, A., Kouznetsova, V., and Goldbaum, M. (2000).
Locating blood vessels in retinal images by piece-wise
threshold probing of a matched filter response. IEEE
Transactions on Medical Imaging, 19(3):203–210.
Kruizinga, P. and Petkov, N. (1999). Nonlinear operator for
oriented texture. IEEE Transactions on Image Pro-
cessing, 8(10):1395–1407.
Lowell, J., Hunter, A., Steel, D., Basu, A., Ryder, R.,
and Kennedy, R. L. (2004). Measurement of reti-
nal vessel widths from fundus images based on 2-d
modeling. IEEE Transactions on Medical Imaging,
23(10):1196–1204.
Wu, D., Zhang, M., and Liu, J. (2006). On the adaptive de-
tetcion of blood vessels in retinal images. IEEE Trans-
actions on Biomedical Engineering, 53(2):341–343.
Wyszecki, G. W. and Stiles, S. W. (1982). Color science:
Concepts and methods, quantitative data and formu-
las. New York, Wiley.
Zhou, L., Rzeszotarsk, M. S., Singerman, L. J., and Chokr-
eff, J. M. (1994). The detection and quantification of
retinopathy using digital angiograms. IEEE Transac-
tions on Medical Imaging, 13.
AN EFFICIENT METHOD FOR VESSEL WIDTH MEASUREMENT ON COLOR RETINAL IMAGES
185