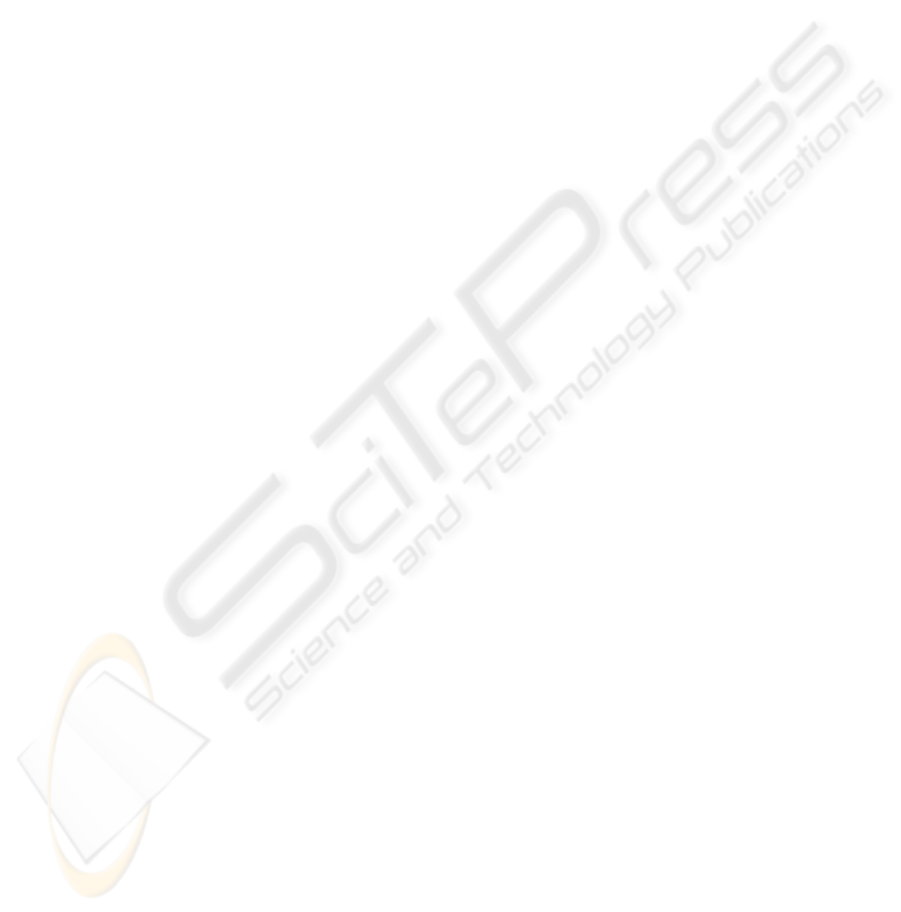
the two previous experiments (global and trait by trait
performances) with very small variances across the 20
samples (≈ 10
−2
for sensitivity and ≈ 10
−3
for speci-
ficity). Thus, the method appears to be stable with
respect to the number of items tested.
5 CONCLUSIONS
We presented a method for the identification of p-
values in omic studies. This approach is based on a
meta-analysis and has two main advantages. On one
side it is computationally efficient, and can thus be
used in interpreted languages such as R and Matlab
that offer rich libraries of functions for omic analyses.
On the other side it is based on the identification of
a p-value rather than FDR, and can thus take advan-
tage of nominal threshold for significance, allowing
for an easier automation of filtering steps in analyses
based on statistical tests. Conversely to the permuta-
tion technique, that remains a computationally inten-
sive but very robust reference method, our approach,
globally, appears to be more specific but less sensi-
tive. This improved specificity can be extremely ad-
vantageous in the practice of Systems Biology, since
novel compact functional subunits can emerge or re-
main uncovered and require longer and costly exper-
imental investigations to be extracted, depending on
the noise they appear to be identified with. Applica-
tion to real data needs to be provided and this repre-
sents our current research activity. For these reasons
we believe the definition of alternative and comple-
mentary method is appropriate.
ACKNOWLEDGEMENTS
The authors would like to thank Diego di Bernardo
and Mukesh Bansal for constructive discussion.
REFERENCES
Bansal, M., Belcastro, V., Ambesi-Impiombato, A., and
di Bernardo, D. (2007). How to infer gene networks
from expression profiles. Mol Syst Biol, 3.
Benjamini, Y. and Hochberg, Y. (1995). Controlling the
false discovery rate: a practical and powerful ap-
proach to multiple testing. J.R. Stat. Soc. B, 57:289–
300.
Cheng, C., Pounds, S., Boyett, J., Pei, D., Kuo, M., and
Roussel, M. F. (2004). Statistical significance thresh-
old criteria for analysis of microarray gene expression
data. Stat Appl Genet Mol Biol, 3:Article36.
Gentleman, R., Carey, V., Huber, W., Irizarry, R., and Du-
doit, S. (2005). Bioinformatics and Computational Bi-
ology Solutions Using R and Bioconductor. Springer.
Lapointe, J., Li, C., Higgins, J. P., van de Rijn, M., Bair,
E., Montgomery, K., Ferrari, M., Egevad, L., Rayford,
W., Bergerheim, U., Ekman, P., DeMarzo, A. M., Tib-
shirani, R., Botstein, D., Brown, P. O., Brooks, J. D.,
and Pollack, J. R. (2004). Gene expression profiling
identifies clinically relevant subtypes of prostate can-
cer. Proc. Natl. Acad. Sci., 101(3):811–816.
L.B.Hedges and I.Olkin ((1985)). Statistical Methods in
Meta-Analysis. Academic Press, New York.
Liang, Y., Diehn, M., Watson, N., Bollen, A. W., Aldape,
K. D., Nicholas, M. K., Lamborn, K. R., Berger,
M. S., Botstein, D., Brown, P. O., and Israel, M. A.
(2005). Gene expression profiling reveals molecularly
and clinically distinct subtypes of glioblastoma multi-
forme. Proc. Natl. Acad. Sci., 102(16):5814–5819.
Nardini, C., Benini, L., and Micheli, G. D. (2006). Circuits
and systems for high-throughput biology. Circuits and
Systems Magazine, IEEE, 6(3):10–20.
Pan, K.-H., Lih, C.-J., and Cohen, S. N. (2005). Effects
of threshold choice on biological conclusions reached
during analysis of gene expression by DNA microar-
rays. Proc. Natl. Acad. Sci., 102(25):8961–8965.
Ramaswamy, S., Ross, K. N., Lander, E. S., and Golub,
T. R. (2003). A molecular signature of metastasis in
primary solid tumors. Nat. Genet., 33(1):49–54.
Rossi, S., Masotti, D., Nardini, C., Bonora, E., Romeo, G.,
Macii, E., Benini, L., and Volinia, S. (2006). TOM:
a web-based integrated approach for efficient identifi-
cation of candidate disease genes. Nucleic Acids Res.,
34(doi:10.1093/nar/gkl340):W285–W292.
R.R.Sokal and F.J.Rohlf (2003). Biometry. Freeman, New
York.
Segal, E., Sirlin, C. B., Ooi, C., Adler, A. S., Gollub,
J., Chen, X., Chan, B. K., Matcuk, G. R., Barry,
C. T., Chang, H. Y., and Kuo, M. D. (2007). De-
coding global gene expression programs in liver can-
cer by noninvasive imaging. Nature Biotechnology,
25(6):675–680.
Storey, J. D. and Tibshirani, R. (2003). Statistical signifi-
cance for genomewide studies. PNAS, 10(16):9440–
9445.
Tiffin, N., Adie, E., Turner, F., Brunner, H., van Driel nd
M. Oti, M. A., Lopez-Bigas, N., Ouzunis, C., Perez-
Iratxeta, C., Andrade-Navarro, M. A., Adeyemo,
A., Patti, M. E., Semple, C. A. M., and Hide,
W. (2006). Computational disease gene identifica-
tion: a concert of methods prioritizes type 2 dia-
betes and obesity candidate genes. Nucleic Acids Res.,
34(doi:10.1093/nar/gkl381).
Tusher, V. G., Tibshirani, R., and Chu, G. (2001). Sig-
nificance analysis of microarrays applied to the ion-
izing radiation response. Proc. Natl. Acad. Sci.,
98(9):5116–5121.
Yang, J. J. and Yang, M. C. (2006). An improved procedure
for gene selection from microarray experiments using
false discovery rate criterion. BMC Bioinformatics,
7:15.
STATISTICAL SIGNIFICANCE IN OMIC DATA ANALYSES - Alternative/Complementary Method for Efficient
Automatic Identification of Statistically Significant Tests in High Throughput Biological Studies
63