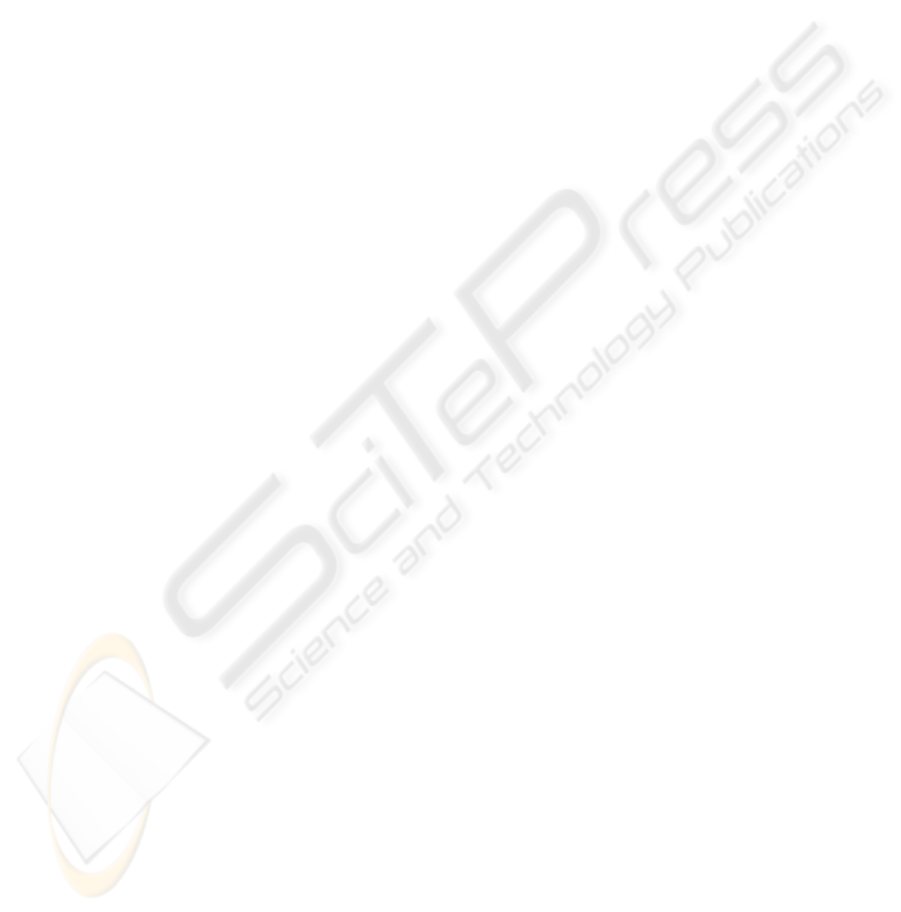
cope with the intrinsic subject variability.
Figure 2 shows the normalized power spectrum
of the channel Fp1 before and after artifact removal
when the first six components were removed. The
harmonic components disappeared. Moreover, re-
moving more than the first five components does not
significantly change the power spectrum of the data.
We can infer that the first six componentswere artifact
related, whereas the remaining sources were EEG-
related.
Therefore, the results confirm the presence of arti-
factual sources that share the same topographies over
time.
4 CONCLUSIONS
We demonstrated that CCA can be a valuable tool
in removing the BCG artifact from simultaneous
EEG/fMRI recording.
We believe that CCA is able to take into account
the physiology of the artifact. The identification of
sources whose topographies do not change over time
allows the use of both spatial and temporal informa-
tion during the identification of the artifact. The use
of a moving window also allows the topographies to
adapt to the physiological variation of the blood flow.
This makes CCA an extension with respect to those
methods, like ICA or PCA, in which only the spa-
tial information is considered. Moreover CCA is less
sensitive than ICA to the window length (Hyvarinen
et al., 2001), allowing the use of a time window that
matches the artifact characteristics.
Further research has to be done in order to auto-
matically detect the BCGa on the EEG data and au-
tomatically identify the number of components to re-
move, in such a way that an optimal reconstruction is
achieved in each window. In order to assess the relia-
bility of the procedure, the application of the method
to a larger database of human recording is also neces-
sary. Moreover a simulation study is needed in order
to test the performances of the algorithm with respect
to noise and artifact characteristics.
REFERENCES
Allen, P. J., Polizzi, G., Krakow, K., Fish, D. R., and
Lemieux, L. (1998). Identification of EEG events in
the MR scanner: the problem of pulse artifact and a
method for its subtraction. Neuroimage, 8:229–239.
Ben´ar, C., Aghakhani, Y., Wang, Y., Izenber, A., Al-Asmi,
A., Dubeau, F., and Gotman, J. (2003). Quality of
EEG in simoultaneous EEG-fMRI for epilepsy. Clin
Neurophysiol, 114:569–580.
Golub, G. and Van Loan, C. (1996). Matrix Computations.
The Johns Hopkins University Press, Baltimore, 3rd
edition.
Hotelling, H. (1936). Relations between two sets of vari-
ates. Biometrika, 28(3/4):321–377.
Hyvarinen, A., Karhunen, J., and Erkki Oja, E. (2001). In-
dependent Component Analysis. Wiley, John & Sons,
Incorporated.
Niazy, R., Beckmann, C., Iannetti, G., Brady, J., and Smith,
S. (2005). Removal of fMRI environment artifacts
from EEG data using optimal basis sets. NeuroImage,
28:720–737.
Srivastava, G., Crottaz-Herbette, S., Lau, K., Glover, G.,
and Menon, V. (2005). ICA-based procedures for re-
moving ballistocardiogram artifacts from EEG data
acquired in the MRI scanner. NeuroImage, 24:50–60.
BIOSIGNALS 2008 - International Conference on Bio-inspired Systems and Signal Processing
16