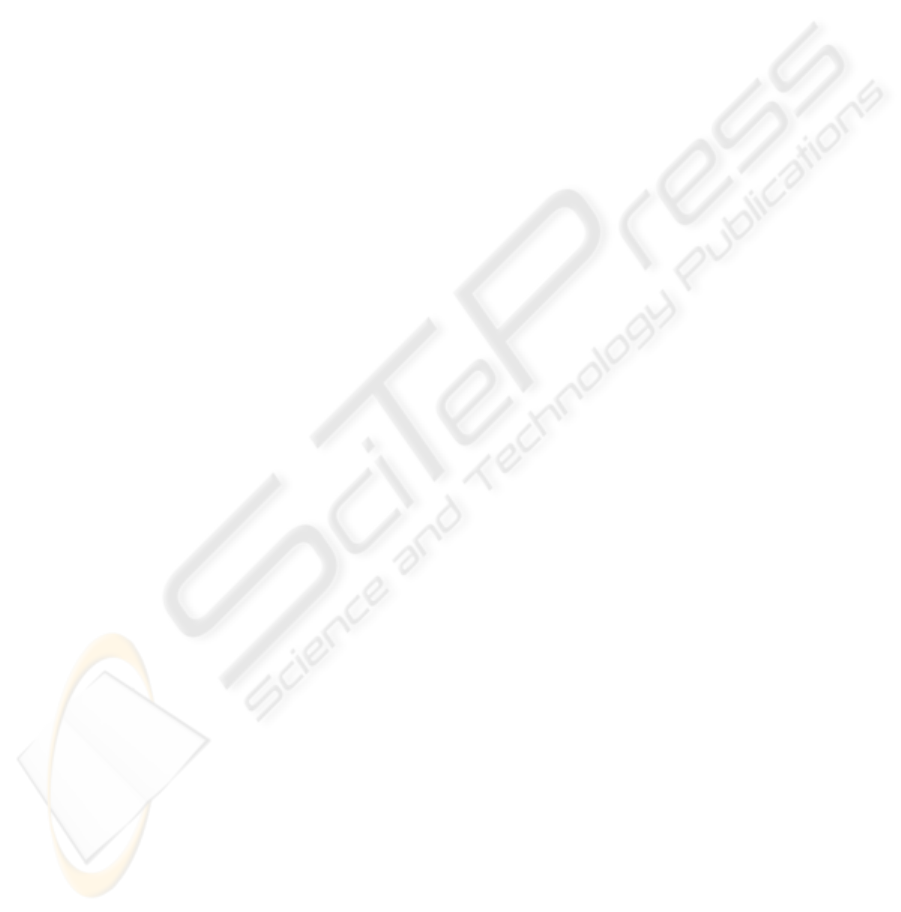
6 CONCLUSIONS AND FUTURE
WORK
Sleep problems belong to the most common serious
neurological disorders. Reliable and robust detection
of these disorders would improve the quality of life
of many people. The implemented methods allow
automatic classification of EEG signals. The
approach has been tested on real sleep EEG
recording for which the classification has been
known. We have focused on discovering the most
significant features which would be highly
correlated with classes of data. Our experiments
have been based on the selection of a single feature
to separate data belonging to two classes. There have
been many other features with good selection
results. The most frequent ones have been
autoregressive features representing the order of
used AR model and error of AR model. We have
determined some features and wavelet coefficients
which are best suited for classification of sleep EEG
data. The future work will be focused on
exploitation of other types of mother wavelets, using
higher level of wavelet coefficients as source of
features, and more sophisticated classifiers.
ACKNOWLEDGEMENTS
This work has been supported by the research
program “Information Society” under Grant No.
1ET101210512 “Intelligent methods for evaluation
of long-term EEG recordings“.
REFERENCES
Agarwal, R., Gotman, J., 2001. Computer-assisted sleep
staging, IEEE Trans. on Biomed. Engineering, 48
(12), pp. 1412-1423.
Bloch,
K.E., 1997. Polysomnography: a systematic review,
Technology and Health Care, 5, pp. 285-305.
Daubechies, I., 1992. Ten lectures on Wavelets, CBMS-
NSF, SIAM, 61, Philadelphia, Pennsylvania, USA.
Doman, J., Detka, C., Hoffman, T., Kesicki, D., Monahan,
J.P., Buysse, D.J., Reynolds III, C.F., Coble, P.A.,
Matzzie, J., Kupfer, D.J., 1995. Automating the sleep
laboratory: implementation and validation of digital
recording and analysis, Int. J. Biomed. Comput., 38,
pp. 277-290.
Frank, E., Hall, M., Trigg, L. Weka – Data Mining
Software in Java. Internet site address: http://
www.cs.waikato.ac.nz.
Gaillard, J.M., Tissot, R., 1973. Principles of automatic
analysis of sleep records with a hybrid system,
Comput. Biomed. Res., 6, pp. 1-13.
Gerla, V., Lhotská, L., Krajča, V., 2005. Utilization of
Time Dependence in EEG Signal Classification. The
3rd European Medical and Biological Engineering
Conference EMBEC´05 Prague.
Johnson, L., Lubin, A., Naitoh, P., Nute, C., Austin, M.,
1969. Spectral analysis of the EEG of dominant and
nondominant alpha subjects during waking and
sleeping, Electroencephalogr. Clin. Neurophysiol., 26,
pp. 361-370.
Kemp,
B., 1993. A proposal for computer-based
sleep/wake analysis, J. Sleep Res., 2, pp. 179-185.
Kemp, B., Zwinderman, A., Tuk, B., Kamphuisen, H.,
Oberyé, J., 2000. Analysis of a sleep-dependent
neuronal feedback loop: the slow-wave
microcontinuity of the EEG. IEEE-BME 47(9), pp.
1185-1194.
Kemp, B., 2007. Sleep Recordings and Hypnograms in
European Data Format. The Netherlands. Internet site
address: http://www.physionet.org/ physiobank/
database/sleep-edf.
Kim, Y., Kurachi, M., Horita, M., Matsuura, K.,
Kamikawa, Y., 1992. Agreement in visual scoring of
sleep stages among laboratories in Japan, J. Sleep Res.,
pp. 58-60.
Larsen, L.E., Walter,
D.O., 1970. On automatic methods
of sleep staging by EEG spectra, Electroencephalogr.
Clin. Neurophysiol., 28, pp. 459-467.
Mourtazaev, M., Kemp, B., Zwinderman, A.,
Kamphuisen, H., 1995. Age and gender affect
different characteristics of slow waves in the sleep
EEG. Sleep 18(7), pp. 557-564.
Quinlan, J.R., 1990. Decision trees and decision making.
IEEE Trans System, Man and Cybernetics, 20(2), pp.
339-346.
Rechtschaffen, A., Kales, A. (eds.), 1968. A manual of
standardized terminology, techniques and scoring
system for sleep stages of human subjects, Brain
Inform. Service/Brain Res. Inst., Univ. California, Los
Angeles.
Schaltenbrand,
N. et al., 1996. Sleep stage scoring using
the neural network model: Comparison between visual
and automatic analysis in normal subjects and patients,
Sleep, 19, pp. 26-35.
Smith, L.I., 2002. A tutorial on Principal Components
Analysis, University of Otago, New Zealand, 2002.
Stanus, E., Lacroix, B., Kerkhofs, M., Mendlewicz, J.,
1987. Automated sleep scoring: A comparative
reliability study of algorithms, Electroencephalogr.
Clin. Neurophysiol., 66, pp. 448-456.
Sweden, B., Kemp, B., Kamphuisen, H., Velde, E., 1990.
Alternative electrode placement in (automatic) sleep
scoring (Fpz-Cz / Pz-Oz versus C4-A1 / C3-A2). Sleep
13(3), pp.279-283.
Therrien, C.W., 1992. Discrete Random Signals and
Statistical Signal Processing. Englewood Cliffs (NJ):
Prentice Hall.
USING WAVELET TRANSFORM FOR FEATURE EXTRACTION FROM EEG SIGNAL
241