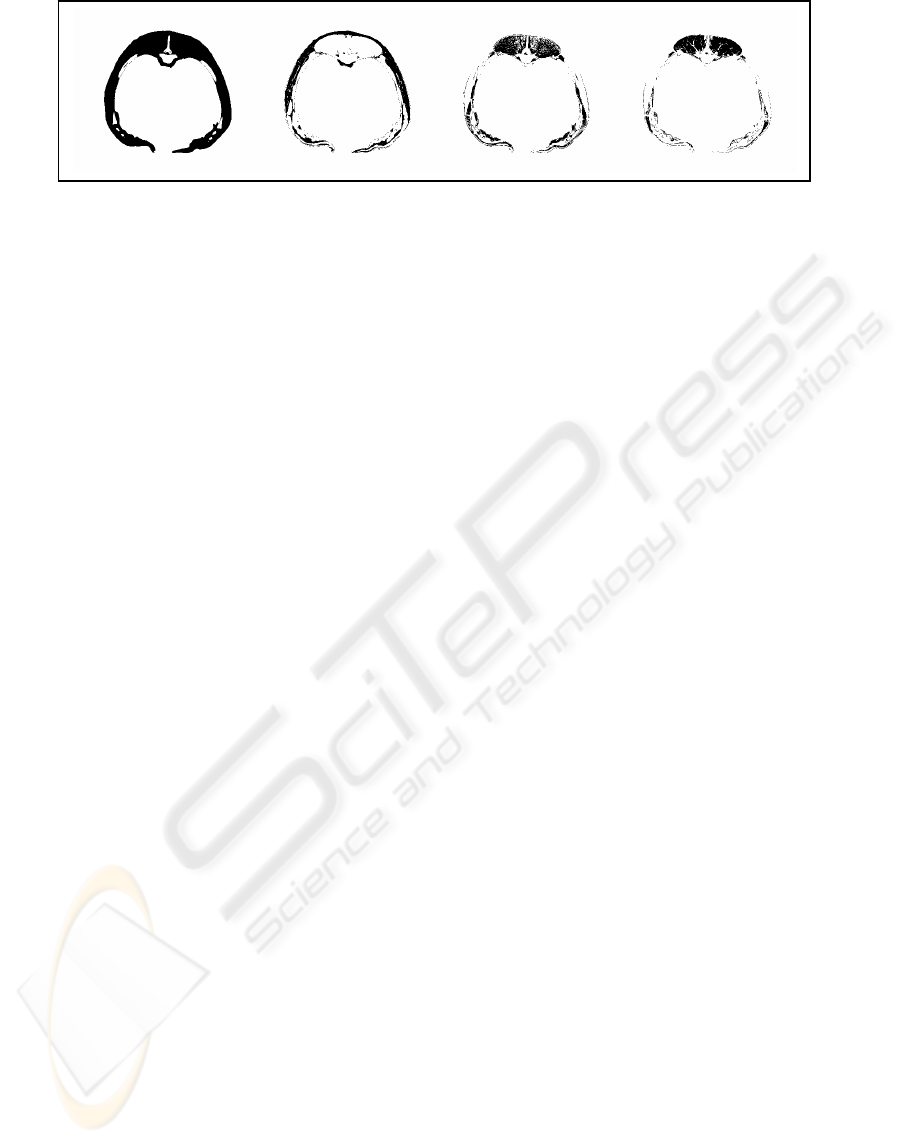
Figure 8; PARAFAC CT value frequency loadings > 0.02 applied on CT image from belly. From left, soft tissue HU= [-
200, 200], # 1, # 2, # 3.
represents component 1, the 3
rd
component 2 and 4
th
(right) component 3. When inspecting the images
visually, # 1 represent fat tissue, # 2 muscle tissue
with marbling fat and the 3
rd
lean muscle tissue.
PARAFAC yields a consistent decomposition of the
3D frequency distribution of the CT images, and
selected 3 unique soft tissue components
representing fat, and two types of muscle tissue.
4 CONCLUSIONS
This paper presents modelling and decomposition of
multi-way array CT image data, using PARAFAC as
a non-supervised classification tool for different
lamb carcass soft tissues. Multi-way modelling
applying PARAFAC did yield sensible interpretation
of the 3D CT value frequency distribution. Three
components or classes of soft tissues were extracted
from the model; fat, marbled and lean muscle.
ACKNOWLEDGEMENTS
This study was sponsored by grant 162188 of the
Research Council of Norway, as part of a Ph.D.
study program. I am grateful for the assistance from
Prof. Rasmus Bro at the Faculty of Life Sciences,
University of Copenhagen.
REFERENCES
Andersen, C. M. & Bro, R. 2003, "Practical aspects of
PARAFAC modeling of fluorescence excitation-
emission data", Journal of Chemometrics, vol. 17, no.
4, pp. 200-215.
Berg, E. P., Neary, M. K., Forrest, J. C., Thomas, D. L., &
Kauffman, R. G. 1997, "Evaluation of Electronic
Technology to Assess Lamb Carcass Composition",
Journal of Animal Science, vol. 75, no. 1997, pp.
2433-2444.
Bro, R. 1997, "PARAFAC. Tutorial and applications",
Chemometrics and Intelligent Laboratory Systems,
vol. 38, no. 2, pp. 149-171.
Bruwer, G. G., Naude, R. T., Dutoit, M. M., Cloete, A., &
Vosloo, W. A. 1987, "An Evaluation of the Lamb and
Mutton Carcass Grading System in the Republic of
South-Africa .2. the Use of Fat Measurements As
Predictors of Carcass Composition", South African
Journal of Animal Science-Suid-Afrikaanse Tydskrif
Vir Veekunde, vol. 17, no. 2, pp. 85-89.
Chandraratne, M. R., Kulasiri, D., Frampton, C.,
Samarasinghe, S., & Bickerstaffe, R. 2006, "Prediction
of lamb carcass grades using features extracted from
lamb chop images", Journal of Food Engineering, vol.
74, no. 1, pp. 116-124.
Chandraratne, M. R., Kulasiri, D., & Samarasinghe, S.
2007, "Classification of lamb carcass using machine
vision: Comparison of statistical and neural network
analyses", Journal of Food Engineering, vol. 82, no. 1,
pp. 26-34.
Cunha, B. C. N., Belk, K. E., Scanga, J. A., LeValley, S.
B., Tatum, J. D., & Smith, G. C. 2004, "Development
and validation of equations utilizing lamb vision
system output to predict lamb carcass fabrication
yields", Journal of Animal Science, vol. 82, no. 7, pp.
2069-2076.
Dobrowolski, A., Romvári, R., Allen, P., Branscheid, W.,
& Horn, P. 2004, "Schlachtkörperwertbestimmung
beim Schwein", Fleischwirtschaft, vol. 3, no. 2004,
pp. 109-112.
Engel, B., Buist, W. G., Walstra, P., Olsen, E. V., &
Daumas, G. 2003, "Accuracy of prediction of
percentage lean meat and authorization of carcass
measurement instruments: adverse effects of incorrect
sampling of carcasses in pig classification", Animal
Science, vol. 76, no. 2003, pp. 199-209.
Huang, J., Wium, H., Qvist, K. B., & Esbensen, K. H.
2003, "Multi-way methods in image analysis-
relationships and applications", Chemometrics and
Intelligent Laboratory Systems, vol. 66, no. 2, pp. 141-158.
Johansen, J., Egelandsdal, B., Røe, M., Kvaal, K., &
Aastveit, A. H. 2006, "Comparison of different
calibration models for prediction of lamb carcass
composition using Computerized Tomography (CT)
imaging", Chemometrics and Intelligent Laboratory
Systems, vol. Submitted.
Jones, S. D. M., Jeremiah, L. E., Tong, A. K. W.,
Robertson, W. M., & Gibson, L. L. 1992, "Estimation
PARAFAC CLASSIFICATION OF LAMB CARCASS SOFT TISSUES IN COMPUTER TOMOGRAPHY (CT) IMAGE
STACKS
247