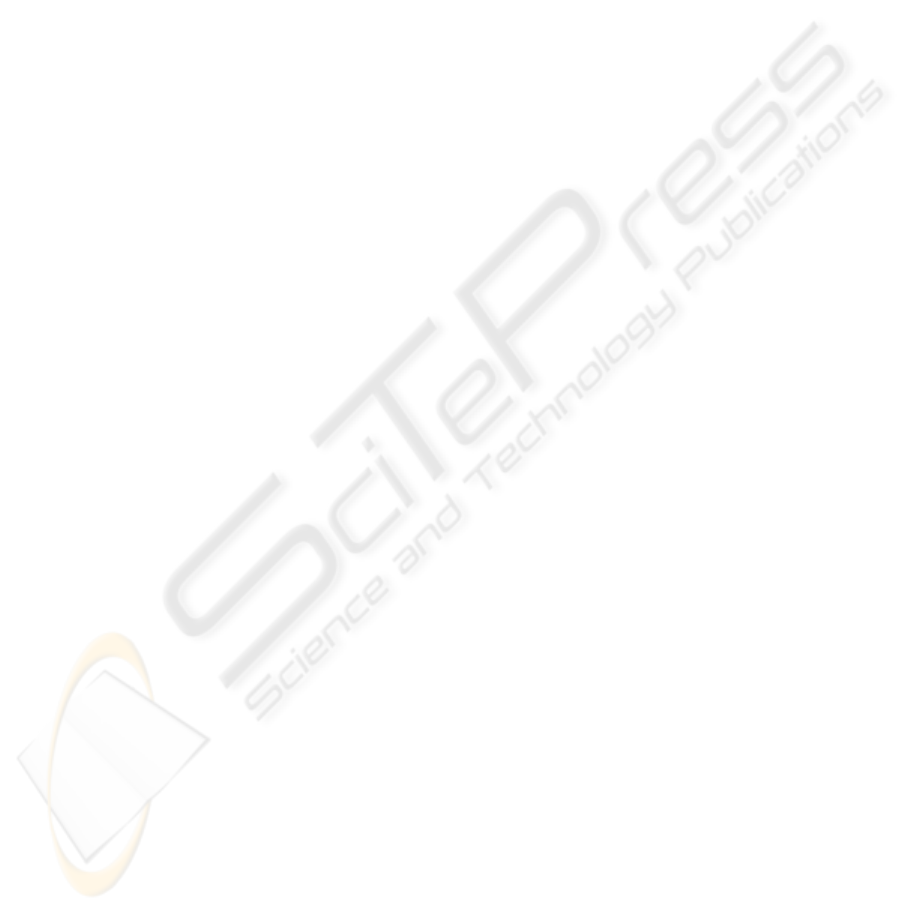
spike detectors based on WT reported in the literature
obtained comparable results with a sensitivity and a
positive predictivity of around 99.8% (Martinez et al.,
2004; Li et al., 1995; Chen et al., 1997). Our algo-
rithm achieves comparable performances without the
need for a more advanced post-processing stage such
as those used in these articles.
6 CONCLUSIONS
In this paper, a supervised learning algorithm for
the automatic detection of R peaks in ECG is in-
troduced. It uses the multiscale feature of the con-
tinuous wavelet transform (CWT) to emphasize the
QRS complex over high P or T waves, noise, base-
line drift and artifacts. The CWT keeps the important
time aspect of the non-stationary ECG signal. More-
over, very efficient implementations of the CWT exist
and a low computational complexity is required, al-
lowing real-time analysis. This algorithm learns and
propagates the annotations provided by a physician
on a small annotated segment. For this purpose, the
method selects the best subset of wavelet scales on a
representative training set by a stepwise forward pro-
cedure. The forward procedure allows to select scales
that are not necessarily consecutive and it does not
a priori restrict the range of computed scales on an
empirical basis. It allows a complete different set
of scales to be selected for each ECG signal, based
on its characteristics. The selected scales are then
used on the original long-term ECG signal recording
and a hard thresholding rule is applied on the deriva-
tive of the wavelet coefficients to label the R spikes.
The method is robust and does not require any pre-
processing stage. The selection procedure can be gen-
eralized in order to detect other ECG features such as
the P and T wave.
Experiments on the public annotated MIT-BIH
database lead to a sensitivity of 99.7% and a posi-
tive predictivity of 99.7% without the need of an ad-
vanced post-processing stage on the detected peaks.
To our knowledge, only three R spike detectors based
on WT reported in the literature obtained comparable
results, while requiring a more complex post process-
ing stage.
Further works will include: (1) The development
of a more advanced thresholding rule that takes the
peaks detected so far into account; (2) the use of
a more advanced post-processing stage to eliminate
wrong detections; (3) the design of an automatic se-
lection of the best mother wavelet by the same learn-
ing methodology; (4) the generalization of the method
for the detection of other ECG features such as P or T
wave.
ACKNOWLEDGEMENTS
This work was partly supported by the Bel-
gian “R
´
egion Wallonne” ADVENS convention 4994
project and by the Belgian “R
´
egion de Bruxelles-
Capitale” BEATS project.
REFERENCES
Acharya, U. R., Joseph, K. P., Kannathal, N., Lim, C., and
Suri, J. (2006). Heart rate variability: a review. Med-
ical and Biological Engineering and Computing, Nov
17.
Addison, P. D. (2005). Wavelet transform and the ecg: a
review. Physiological Measurement, 26:155–199.
Algra, A. and Zeelenberg, H. (1987). An algorithm for com-
puter measurement of qt intervals in the 24 h ecg. Pro-
ceedings of the IEEE Computer Society Press, page
1179.
Chen, S., Chen, H., and Chan, H. (1997). Dsp implementa-
tion of wavelet transform for real time ecg waveforms
detection and heart rate analysis. Computer Methods
and program in Biomedicine, 55(1):35–44.
Chen, S., Chen, H., and Chan, H. (2006). A real-time qrs
detection method based on moving-averaging incor-
porating with wavelet denoising. Comput Methods
Programs Biomed., 82(3):187–95.
Chen, Y., Yan, Z., and He, W. (2005). Detection of qrs-wave
in electrocardiogram: Based on kalman and wavelets.
Conf Proc IEEE Eng Med Biol Soc., 3:2758–60.
Christov, I. I. (2004). Real time electrocardiogram qrs de-
tection using combined adaptive threshold. BioMedi-
cal Engineering OnLine, 3(1):28.
Clavier, L., Boucher, J.-M., Lepage, R., Blanc, J.-J., and
Cornily, J.-C. (2002). Automatic p-wave analysis of
patients prone to atrial fibrillation. Med Biol Eng
Comp, 40:6371.
Daubechies, I. (1992). Ten Lectures on Wavelets (C B M S
- N S F Regional Conference Series in Applied Math-
ematics). Soc for Industrial & Applied Math.
Dobbs, S., Schmitt, N., and Ozemek, H. (1984). Qrs detec-
tion by template matching using real-time correlation
on a microcomputer. Journal of clinical engineering,
9(3):197–212.
Fard, P. J. M., Moradi, M., and Tajvidi, M. (2007). A novel
approach in r peak detection using hybrid complex
wavelet (hcw). International Journal of Cardiology,
In press(Available online 27 March 2007).
Goldberger, A., Amaral, L., Glass, L., Hausdorff, J., Ivanov,
P., Mark, R., Mietus, J., Moody, G., Peng, C.-K.,
and Stanley, H. (2000). PhysioBank, PhysioToolkit,
and PhysioNet: Components of a new research re-
source for complex physiologic signals. Circulation,
101(23):e215–e220.
BIOSIGNALS 2008 - International Conference on Bio-inspired Systems and Signal Processing
144