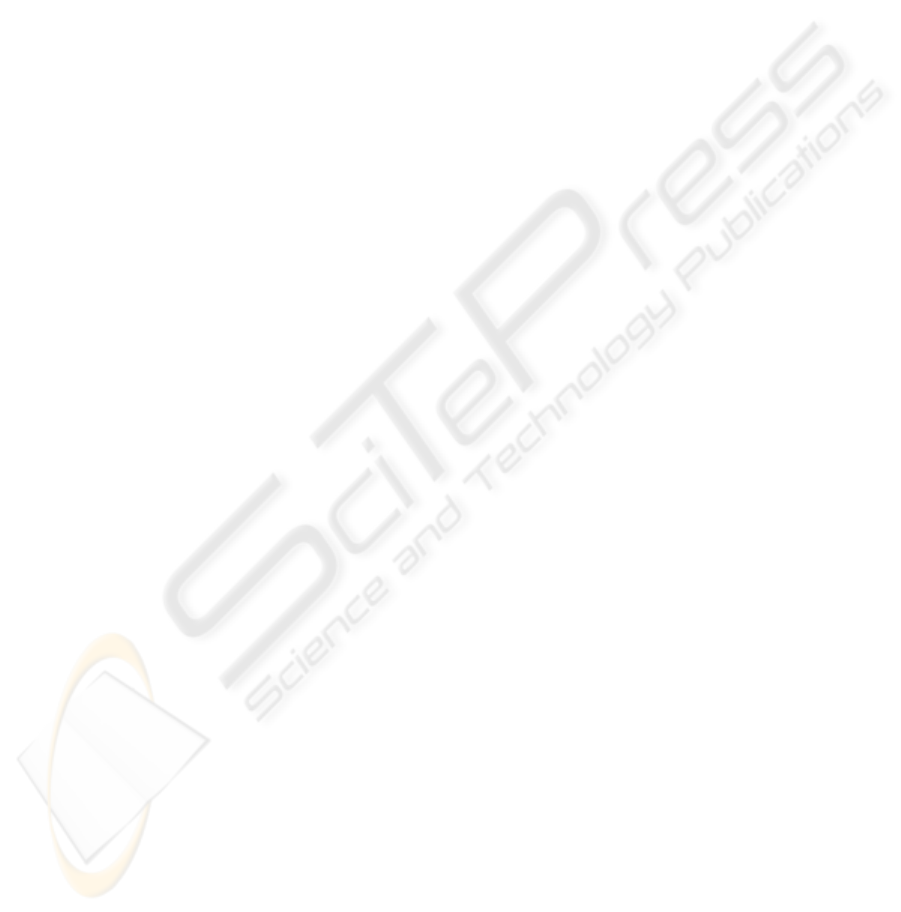
4 CONCLUSIONS
Results show that UV-VIS-SWNIR spectroscopy is a
feasable technology for plate count agar microorgan-
isms identifications. The robust mean scattering cor-
rection algorithm was able to efficiently remove the
growth media and colonies scattering artifacts, allow-
ing a better interpretation of the singular value de-
composition scores loading. In this exploratory ex-
periment, VIS-SWNIR wavelengths were able to pro-
duce better discriminations between microorganisms
than the UV-VIS region. Nevertheless, experimen-
tal methodology and signal processing improvements
proposed may increase the discrimination resolution,
making UV-VIS-SWNIR an attractive methodology
for rapid microorganisms identification in plate count
agar.
REFERENCES
Avantes (2007). Avaspec-2048-4-dt/-rm.
http://www.avantes.com.
Baig, S. and Rehman, F. (2006). Signal modeling using sin-
gular value decomposition. In Advances in Computer,
Information, and Systems Sciences, and Engineering.
Springer Netherlands.
Berger, A. and Zhu, Q. (2003). Identification of oral bacte-
ria by raman microspectroscopy. In Journal of Mod-
ern Optics 50(15-17): 2375-2380. Taylor and Francis
Group.
Bhatta, H., Goldys, E., and Learmonth, R. (2005). Rapid
identification of microorganisms by intrinsicfluores-
cence. In Imaging, Manipulation, and Analysis of
Biomolecules and Cells: Fundamentals and Applica-
tions III,. SPIE.
Difco, L. (2005). Difco manual : dehydrated culture media
and reagents for microbiology. - VII. Difco Laborato-
ries, Detroit, USA.
Dziuba, B., Babuchowski, A., Naleczb, D., and Niklewicz,
M. (2007). Identification of lactic acid bacteria using
ftir spectroscopy and cluster analysis. In International
Dairy Journal 17: 183189. Elsevier.
Gallagher, N. B., Blake, T., and Gassman, P. (2005). Ap-
plication of extended inverse scattering correction to
mid-infrared reflectance of soil. In Journal of Chemo-
metrics 19: 271-281.
Guibeta, F., Marieya, L., Pichonb, P., Traverta, J., Denisb,
C., and Amiela, C. Discrimination and classifica-
tion of enterococci by fourier transform infrared spec-
troscopy.
Hammes, G. G. (2005). Fundamentals of spectroscopy. In
Spectroscopy for the Biological Sciences. John Wiley
Sons, Inc.
Harz, M., Rosch, P., Peschke, K.-D., Ronneberger, O.,
Burkhardtb, H., and Popp, J. (2005). Micro-raman
spectroscopic identification of bacterial cells of the
genus staphylococcus and dependence on their cul-
tivation conditions. In Analyst 130: 15431550. The
Royal Society of Chemistry.
Jolliffe, I. (1986). Principal Component Analysis. Springer,
New York, USA.
Krzanowski, W. J. (1998). Principles of Multivariate Data
Analysis. Oxford University Press, Oxford, UK.
Manly, B. F. (1998). Randomization, Bootstrap and Monte
Carlo Methods in Biology. Chapman and Hall, Lon-
don, UK, 2nd edition.
Mariey, L., Signolle, J., Amiel, C., and Travert, J. (2001).
Discrimination, classification, identification of mi-
croorganisms using ftir spectroscopy and chemomet-
rics. In Vibrational Spectroscopy 26: 151-159. Else-
vier.
Martens, H., Nielsen, J. P., and Engelsen, S. B. (2003).
Light scattering and light absorbance separated by ex-
tended multiplicative signal correction. application to
near-infrared transmission analysis of powder mix-
tures. In Analytical Chemistry 75(9): 394-404. Amer-
ican Chemical Society.
Martens, H. and Stark, E. (1991). Extended multiplicative
signal correction and spectral interference subtraction:
new preprocessing methods for near infrared spec-
troscopy. In Journal of Pharmaceutical and Biomedi-
cal Analysis 9: 625-635. American Chemical Society.
Oberreuter, H., Mertens, F., Seiler, H., and Scherer, S.
(2000). Quantification of micro-organisms in binary
mixed populations by fourier transform infrared (ft-
ir) spectroscopy. In Letters in Applied Microbiology
2000, 30: 8589. The Society for Applied Microbiol-
ogy.
Oberreuter, H., Seiler, H., and Scherer, S. (2002). Iden-
tification of coryneform bacteria and related taxa by
fourier-transform infrared (ft-ir) spectroscopy. In In-
ternational Journal of Systematic and Evolutionary
Microbiology 52: 91100. IUMS.
Perkauparus, H., Grinter, H., and Therfall, T. (1994). Uv-Vis
spectroscopy and its applications. Springer-Verlag,
New York, USA.
R-Project (2006). R: A programming environment for data
analysis and graphics. URL: http://www.r-project.org.
Rch, P., Harz, M., Schmitt, M., and Popp, J. (2005). Raman
spectroscopic identification of single yeast cells. In J.
Raman Spectrosc 36: 377379. Wiley InterScience.
Riddle, J., Kabler, P., Kenner, B., Bordner, R., Rockwood,
S., and Stevenson, H. (1956). Bacterial Identification
by Infrared Spectrophotometry. Cincinnati, Ohio.
Winder, C., Carr, E., Goodacre, R., and Seviour, R. (2004).
The rapid identification of acinetobacter species using
fourier transform infrared spectroscopy. In Journal
of Applied Microbiology 96, 328339. The Society for
Applied Microbiology.
BIOSIGNALS 2008 - International Conference on Bio-inspired Systems and Signal Processing
32