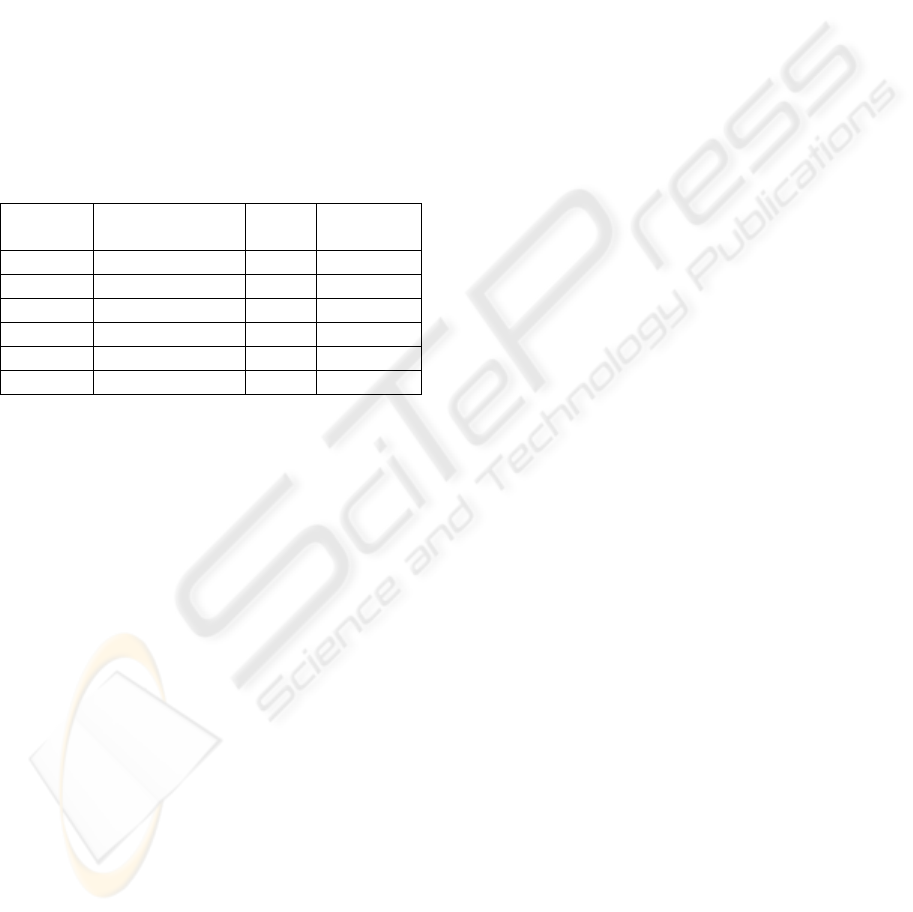
the “texture mosaic problem” proposed in
(Grossberg and Williamson, 1999). Our mosaic
includes 9 textural areas versus the 5 textural areas
from (Grossberg and Williamson, 1999). As
explained before, with the third image from each
texture class, we built a 210x210 pixels multitex test
image (see Figure 4 row 3-right) in order to evaluate
the frontier precision between textures in the
prediction of our architecture. Both the training and
the testing was performed with two different levels
of vigilance, ρ=0.95 and ρ=0.98 for training and
ρ=0.9 and ρ=0.97, respectively for testing. The
results are shown in Table 5. Those results show a
better class rate in all resolutions and vigilance
levels than those obtained in (Grossberg and
Williamson, 1999) as our worst result (95.89%)
beats the best result (95.7%) shown in this work.
Table 5: Multitex prediction statistics.
Resolution Train vigilance
parameter
Samples
/class
Class rate
(%)
8x8 0.95 300
95.89
16x16 0.95 125
96.67
32x32 0.95 40
97.30
8x8 0.98 300
99.75
16x16 0.98 125
99.48
32x32 0.98 40
99.18
The images of the predictions can be seen in
Figure 5 where only those corresponding to a
vigilance parameter value of ρ=0.95 are shown.
Each prediction class is depicted with a level of
grey, from black to white. Those images reveal two
remarkable points. First, the best prediction for the
interior points shows up for a 32x32 resolution.
However, it is the 8x8 resolution the one which
accurately resolve texture transitions.
The main differences between our architecture
and the one shown in (Grossberg and Williamson,
1999) are basically the inclusion of colour
information (the two output signals coming from the
chromatic channels in the diffusion stage) and the
use of one additional receptive field in the pattern’s
textural components. Our architecture also includes
in the patterns the processing of the symetric
receptive field with central excitation simple cells.
REFERENCES
Carpenter, G.A., Grossberg, S., Markuzon, N. and
Reynolds, J.H. (1992). Fuzzy ARTMAP : A Neural
Network Architecture for Incremental Supervised
Learning of Analog Multidimensional Maps. Neural
Networks, 3, 5, 698 -713.
Daugman, J.G. (1980) Two-dimensional spectral analisis
of cortical receptive field profiles. Vision Research,
20, 847-856.
Gonzalez, R.C. and Woods R.E. (2002), Digital Image
Provessing, 2/E, Prentice Hall.
Grossberg, S. (1984). Outline of a theory of brightness,
colour, and form perception. In E. Degreef and J. van
Buggenhault (Eds.) Trends in mathematical
psychology. Amsterdam: North Holland.
Grossberg, S. and Hong, S. (2006). A Neural Model of
Surface Perception: Lightness, Anchoring, and Filling-
In. Spatial Vision, 19, 2, 263-321.
Grossberg, S. and Williamson, J.R. (1999). A self-
organizing neural system for leaning to recognize
textured scenes, Vision Research, 39, pp. 1385-1406.
Grossberg, S., and Mingolla, E. (1988). Neural dynamics
of perceptual grouping: textures, boundaries, and
emergent segmentations. In Grossberg, S. (Ed.): The
adaptive brain II. Chap. 3. Amsterdam, North Holland,
1988.
Grossberg, S., Mingolla, E. and Williamson, J. (1995).
Synthethic aperture radar processing by a multiple
scale neural system for boundary and surface
representation. Neural Networks, 8, 1005-1028.
Hubel D.H. (1995) Eye, Brain and Vision. Scientific
American Library, No. 22., WH Freeman, NY. p. 70.
Hubel, D.H., and Livingstone, M.S. (1990). Color and
contrast sensitivity in lateral geniculate body and
Primary Visual Cortex of the Macaque Monkey. The
Journal of Neuroscience, 10, 7, 2223-2237.
Intel Corporation (2006). Open Source Computer Vision
Library. http://www.intel.com/technology/computing/
opencv/
Julesz, B., and Bergen, R. (1987), Textons, The
Fundamental Elements in Preattentive Vision and
Perception of Textures. In Fischer and Firschen (eds.).
Readings in Computer Vision, 243-256, 1987.
Mingolla, E., Ross, W. and Grossberg, S. (1999) A neural
network for enhancing boundaries and surfaces in
synthetic aperture radar images. Neural Networks, 12,
499-511, 1999.
Pollen, D.A. and Ronner, S.F. (1983) Visual cortical
neurons as localized spatial frequency filters. IEEE
Transactions on Systems, Man, and Cybernetics,
SMC-13, No 15, 907-916.
BIOSIGNALS 2008 - International Conference on Bio-inspired Systems and Signal Processing
184