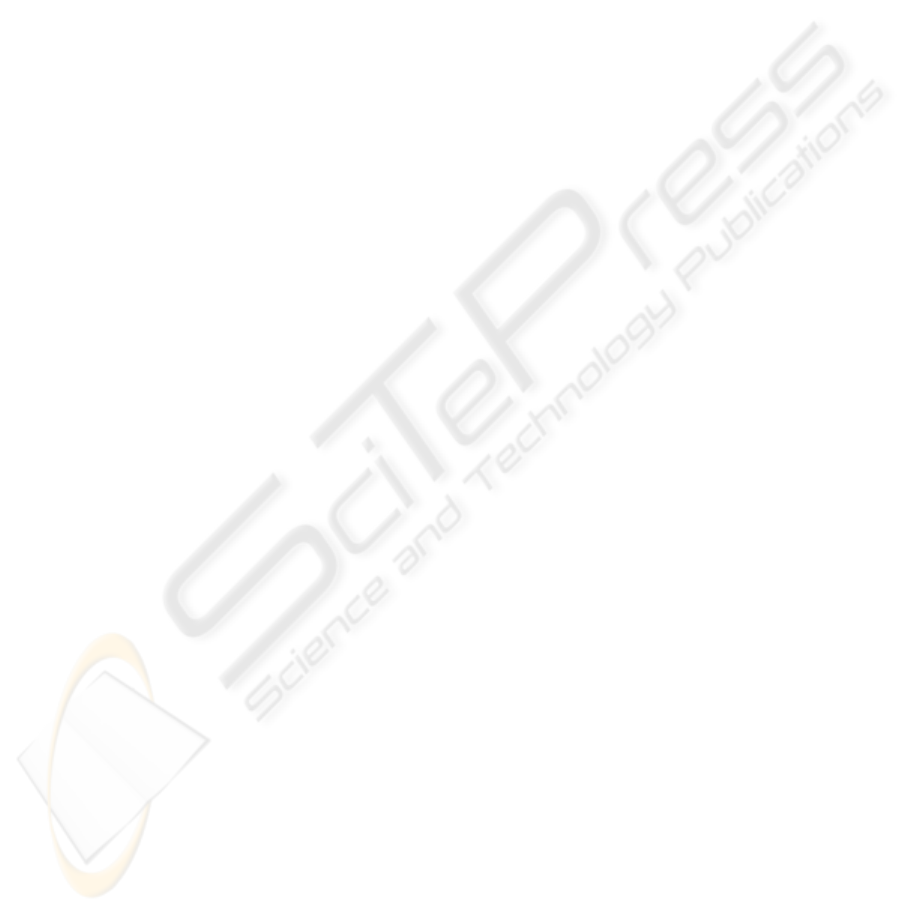
conclude that SampEn does not provide a good
discrimination.
From Fig. 2 is can be observed that while Sample
Entropy and Lempel-Ziv complexity values decrease
as a seizure is occurring, Shannon Entropy and
Multi-Scale Entropy increase as a seizure is taking
place. Similar behavior of entropy measures were
reported in (Costa et al., 2005) for ECG analysis and
(Ferenets et al., 2006) for EEG analysis. Ferenets et
al explain that ShEnt “is indifferent to the time order
of the signal”, while SampEnt and LZ are dependent
on the order of signal thus this might explain the
behavior mentioned above.
In a recently reported EEG based detection
method (Greene, 2006) six features were extracted,
one being Spectral Entropy. The patient specific
results reported in (Greene, 2006) showed that the
best performing feature was line length, while
Spectral Entropy and Non-linear Energy were
second best performing features. Therefore, it would
be beneficial to investigate if adding Spectral
Entropy to the list of features extracted in this study
will improve the overall performance of the
detection method.
In this study, the total amount of data employed
was 10.13 hours. In order to attain a clinically
relevant performance estimate for the method
proposed, a much larger data set would be required.
Using the features, with the parameter values chosen
from this study, on a new larger dataset containing
multi-channel continuously recorded EEG, would
further validate the effectiveness of these measures
in neonatal seizure detection.
9 CONCLUSIONS
The conclusion drawn from this study is that out of
the four entropy/complexity measures investigated.
Shannon entropy provides the best discrimination
between seizure and non-seizure EEG in the
neonate.
ACKNOWLEDGEMENTS
B. R. Greene was supported by Science Foundation
Ireland (SFI/05/PICA/1836).
REFERENCES
Aboy, M., Hornero, R., Abasolo, D., Alvarez, D., 2006.
Interpretation of the Lempel-Ziv Complexity measure
in the context of biomedical signal analysis. IEEE
Transactions on Biomedical Engineering, 53(11), p.
2282-2288.
Costa, M., Goldberger, A. L., Peng, C. K., 2005.
Multiscale Entropy Analysis of Biological Signals.
Physical Review, E;71:021906.
De Weered, A. W., Despland, P. A., Plouin, P., 1999.
Neonatal EEG. The International Federation of
Clinical Neurophysiology. Electroencephalography
Clinical Neurophysiology, 52, p. 149-157.
Ferenets, R., Lipping, T., Anier, A., Jantii, V., Melto, S.,
Hovilehto, S., 2006. Comparison of entropy and
complexity measures for the assessment of depth of
sedation, IEEE Transactions on Biomedical
Engineering, 53(6). p. 1067-1077, JUNE.
Goldberger, A. L., Amaral, L.A N., Glass, L., Hausdorff,
J. M., Ivanov, Mark, P. C. R. G., Mietus, J. E.,
Moody, G. B., Peng, C., Stanley, H. E., 2000.
PhysioBank, PhysioToolkit, and PhysioNet,
Components of a New Research Resource for
Complex Physiologic Signals. Circulation
101(23),e215-e220.
Gotman, J., Flanagan, D., Zhang, J., Rosenblatt, B., 1997.
Automatic seizure detection in newborns: methods and
initial evaluation. Electroencephalography and
clinical Neurophysiology, 103, p. 356-362.
Greene, B., Boylan, G., Reilly, R., de Chazal, P.,
Connolly, S., 2007. Combination of EEG and ECG for
improved automatic neonatal seizure detection,
Clinical Neurophysiology. 118, 1348-1359.
Greene, B. R., 2006, Quantification and classification of
Electrophysiological Markers of Seizure in the
Neonate. PhD thesis, University College Dublin,
National University of Ireland.
Lempel, A., Ziv, J., 1976. On the complexity of finite
sequences. IEEE Transactions on Information
Theory, IT-28(1), p. 75-81.
Richman, J. S., Moorman, J. R., 2000. Physiological time-
series analysis using approximate entropy and sample
entropy. American Journal of Physiology - Heart
Circulatory Physiology, 278(6). H2039-H2049.
Shannon, C. E., A mathematical theory of communication.
1948. Bell System Technical Journal, 27. p. 379-
423.
Volpe, J. J., 2001. Neurology of the newborn. 4
th
ed.
Philadelphia PA.: Saunders.
Zweig, M., Campbell, G., 1993. Receiver-Operating
Characteristic (ROC) plots: a fundamental evaluation
tool in clinical medicine. Clinical Chemistry, 39(4), p.
561-577.
BIOSIGNALS 2008 - International Conference on Bio-inspired Systems and Signal Processing
22