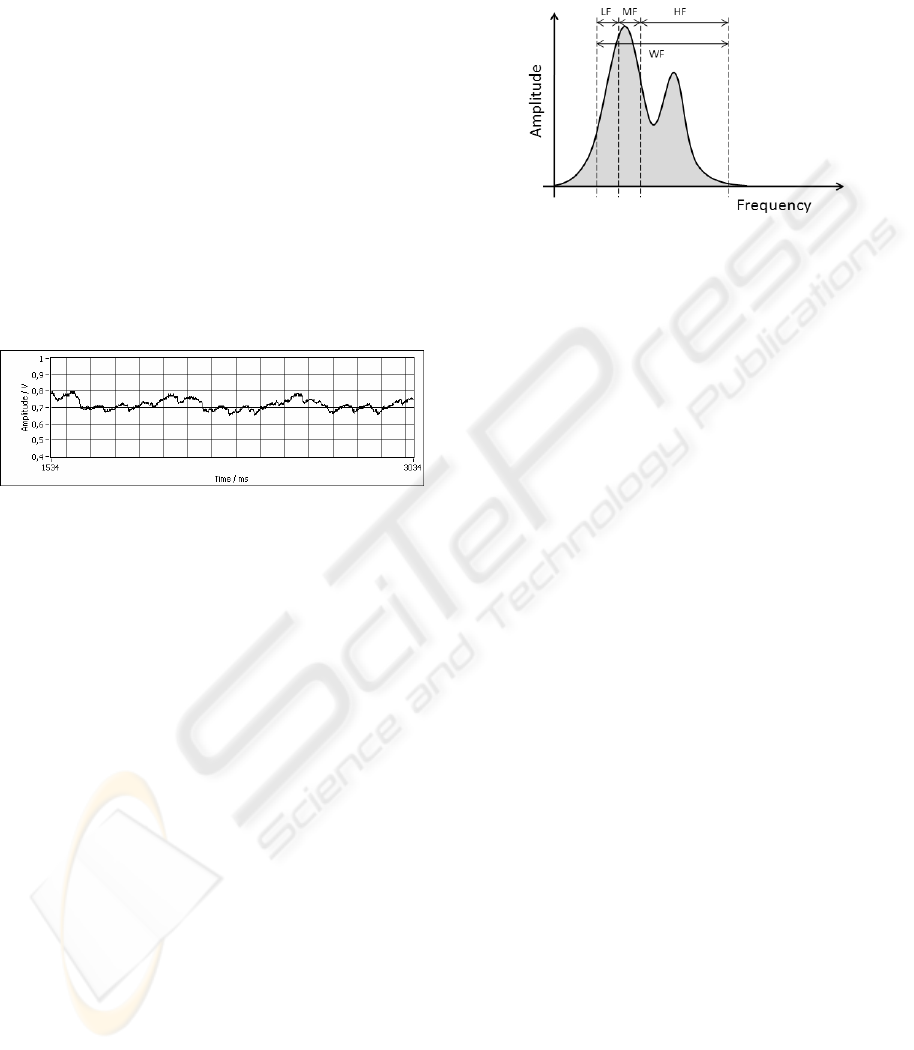
distance to any organic object in- or decreases. This
change in resonance frequency is reflected in a
change of voltage. This principle is used in homes in
the form of touchless switches. However, since the
changes in the tissue surface caused by pulse beat
and respiration are very small, the use of classical
LC oscillators is - apart from a few exceptions - not
possible. Therefore a new nonlinear oscillator has
been developed. With its help it is possible to
measure changes in submillimeter range. Because of
this high sensitivity even little muscle contractions
will be detected by the sensor. As some of these
minor contractions do belong to neither pulse nor
respiration they have to be eliminated. It is
necessary to filter and process the signal to eliminate
these artifacts. An example for the measured signal
is shown in Fig. 2. For more technical information
please refer to (Jaeger, 2007).
Figure 2: Signal measured by the sensor.
2.3 Algorithms
First the signal is band-filtered with hard coded cut-
off frequencies covering the whole spectrum of
possible pulse and respiration frequencies. In the
next step a low resolution FFT of this filtered signal
is performed. In extreme cases the respiration
frequency can be higher than the pulse frequency.
Due to this there are four possible frequency bands
(Fig.3):
- the whole frequency band from the lowest
possible frequency of the respiration to the
highest possible frequency of the pulse (WF)
- the lower frequency band of respiration
from the lowest possible frequency of the
respiration to the lowest possible frequency of
the pulse (LF),
- the upper frequency band of pulse, from
the highest possible frequency of the respiration
to the highest possible frequency of the pulse
(HF)
- and the overlapping band from the lowest
possible frequency of the pulse to the highest
possible frequency of the respiration (MF).
By calculating relations between the different
amplitudes, the frequency parts with high intensity
can be discerned. Normally there will be only two
frequency parts with high intensity representing
pulse and respiration.
Figure 3: Spectrum of the filtered signal with four
frequency bands.
If there are more than two such frequency parts,
there has to be a measurement error. If no error is
detected the frequency parts defined above can be
analyzed further. If there is a frequency part of high
intensity in the lower frequency band of respiration,
there may be only one such frequency part in the
overlapping band - the one of the pulse. If such a
frequency part is additionally detected in the upper
frequency band of the pulse there cannot be any in
the overlapping band. As soon as these conditions
are met, the frequencies for pulse and respiration are
approximately determined. With the detected
frequencies it is possible to define narrow cut-off
frequencies for pulse and respiration. With these
cut-off frequencies the input signal is filtered again,
once for pulse and once for respiration.
After this step there are two rather clear signals
which are relatively free of errors.
With these
filtered signals it is possible to determine more exact
frequencies for pulse and respiration. Therefore the
filtering is designed to adapt which means that the
cut-off frequencies of the fine filters are always
readjusted using the frequencies determined by the
fine filtered signals. Additionally the approximated
frequencies of pulse and respiration gained from the
roughly filtered input signal are used to validate the
adjustment of the cut-off frequencies for the fine
filters. The determination of the frequency of the
fine filtered signal works as follows: On the one
hand zero-crossings are counted using a hysteresis,
on the other hand a high resolution FFT for the
filtered signals is processed. Both results undergo a
plausibility check.
The frequency determination by counting zero-
crossings is done by using hysteresis. A zero-
crossing is only interpreted as such if the signal does
not only drop below a lower hysteresis border but
BIOSIGNALS 2008 - International Conference on Bio-inspired Systems and Signal Processing
260