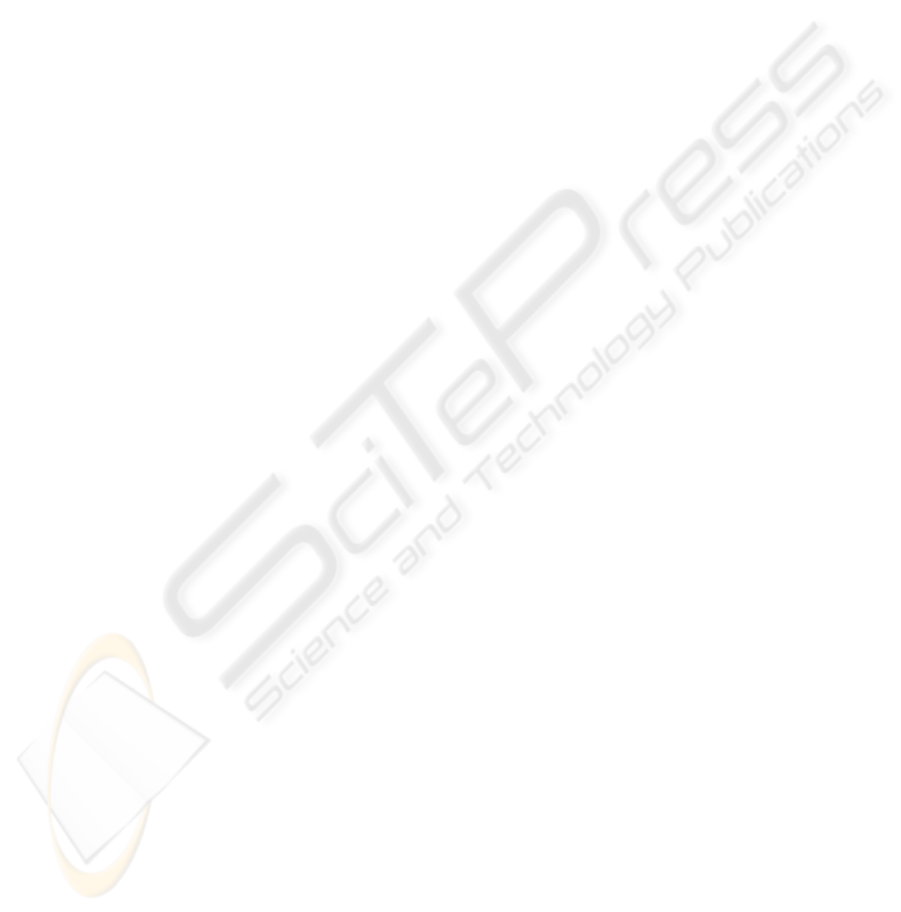
IMPROVING AN AUTOMATIC ARRHYTHMIAS RECOGNISER
BASED IN ECG SIGNALS
Jorge Corsino, Carlos M. Travieso, Jesús B. Alonso and Miguel A. Ferrer
Technological Centre for Innovation in Communications (CeTIC), University of Las Palmas de Gran Canaria
Campus de Tafira, s/n, 35017, Las Palmas de Gran Canaria, Spain
Keywords: Automatic recognition of arrhythmias, electrocardiography, neural network, principal component analysis,
wavelet transform.
Abstract: In the present work, we have developed and improved a tool for the automatic arrhythmias detection, based
on neural network with the “more-voted” algorithm. Arrhythmia Database MIT has been used in the work in
order to detect eight different states, seven are pathologies and one is normal. The unions of different blocks
and its optimization have found an improvement of success rates. In particular, we have used wavelet
transform in order to characterize the patron wave of electrocardiogram (ECG), and principal components
analysis in order to improve the discrimination of the coefficients. Finally, a neural network with more-
voted method has been applied.
1 INTRODUCTION
In Europe, cardiovascular diseases are one of most
important causes of death, with a great repercussion
in health assistance budget. For instance, to obtain
an early exact cardiovascular diagnosis is one of the
most important missions for the physicians. The
electrocardiogram is the graphic description of the
heart electric activity registered from the body
surface and is a basic element in the diagnosis of
different heart diseases.
The objective of this study is to make deeper in
the extraction of characteristics and the later
automatic classification of heart pathologies,
analyzing every aspect that takes parting.
To carry on with this objective, we have
developed Matlab software (Matlab, 2006), clear
and easy, where users have three options to practise
with all tools at their hands: making a pre-processing
with wavelet transform and in order to play with the
developed filing.
Wavelet transform (Romero-Legarreta, 2005) is
a mathematics technique that has gained importance
in the last years in all kind of applications related
with non-stationary signal process.
Although the decomposition in well defined
blocks in time and frequency, wavelet transform can
characterise the local sign regularities. This skill
allows distinguishing electrocardiogram waves
(ECG) from noise and other artefacts.
In this paper, we establish the use of
approximated wavelet coefficients taken out from
the ECG signal in order to classify eight types of
beat: normal pulse (N), extra-systole (L), premature
ventricular contraction (R), premature auricular
contraction (/), blockade left branch (A), blockade
right branch paced beat (V), fusion of normal and
paced beat (f) and fusion of normal and premature
ventricular contraction (F).
The use of principal component analysis (PCA)
(Bianchi, 2006) on the wavelet coefficients has
improved their discrimination. Finally, we have used
an automatic classification based on artificial neural
networks (NN) (Bishop, 1995), (Juang, 1992). An
improvement have been applied to NN, we have
implemented the “more voted” method, obtaining
better success rates.
2 WAVELET TRANSFORM:
FEATURE EXTRACTION
The ECG features are extracted through a pre-
processing stage in which the Wavelet transform is
applied to original ECG signal.
The Discrete Wavelet Transform (DWT) is
defined as follows:
453
Corsino J., M. Travieso C., B. Alonso J. and A. Ferrer M. (2008).
IMPROVING AN AUTOMATIC ARRHYTHMIAS RECOGNISER BASED IN ECG SIGNALS.
In Proceedings of the First International Conference on Bio-inspired Systems and Signal Processing, pages 453-457
DOI: 10.5220/0001066604530457
Copyright
c
SciTePress