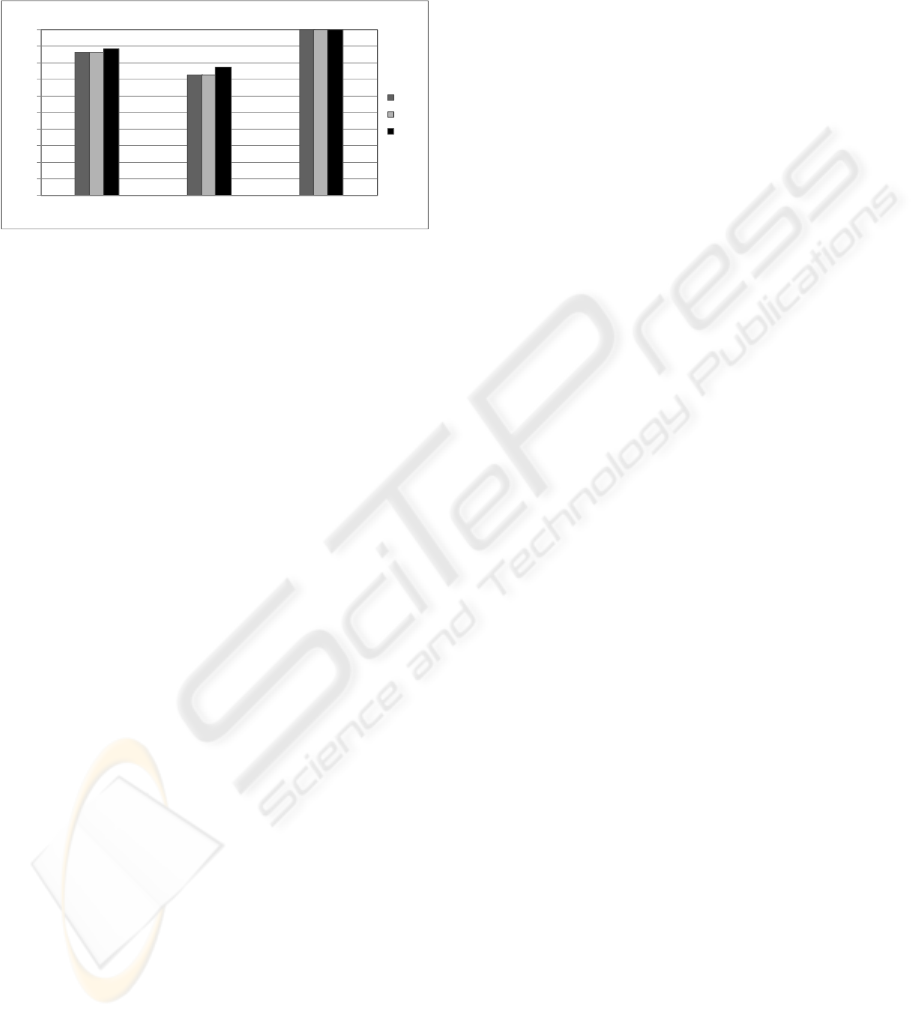
The other pathologies are not considered here. The
ability of FFT-MFCC in modeling the irregular
vibration of the vocal folds provoked by the
pathology is shown in the results. Good results are
also obtained to LPC and cepstral analysis.
0
10
20
30
40
50
60
70
80
90
100
E ( % ) SE( %) SP ( %)
LPC
CEP
MEL
Figure 6: A comparison of the performance evaluation of
LPC, cepstral and mel-cepstral analysis to the cases of
vocal fold edema and normal voices.
5 CONCLUSIONS
The changes on LPC, cepstral and mel-cepstral
coefficients describe the abnormal behaviour of the
vocal folds movements caused by the pathologies.
The efficiency in characterizing pathological voices
using short-time cepstral analysis is well described
by results.
It is noted that mel-cepstral coefficients are very
good to detect the presence of pathology. They
provide a good separation of normal and
pathological voices. However, this method is not
efficient in discriminating distinct pathologies. The
differences among pathologies which belong to
similar class of diseases are not evident. LPC and
cepstral methods seem to be better in representing
the pathologies specificities.
In order to improve the performance of the
classification process, two aspects are suggested: 2)
the use of non-linear analysis to improve the
acoustic modeling of non-linear characteristics
inherent to speech signal, and 2) the employment of
other classifiers based on Artifitial Neural Networks
or Hideen Markov Models, for example.
REFERENCES
Bou-Ghazale, S.E., Hansen, J.H.L., 2000. A Comparative
Study of Traditional and Newly Proposed Features for
Recognition of Speech Under Stress. IEEE
Transactions on Speech & Audio Processing. Vol. 8,
no. 4, pp. 429-442, July.
Davis, S. B, 1979. Acoustic Characteristics of Normal and
Pathological Voices. Speech and Language: Advances
in Basic Research and Practice. Vol. 1, pp. 271–335.
Dibazar, A. A., Berger, T.W., and Narayanan, S. S., 2006.
Pathological Voice Assessment. Proceedings of the
28th IEEE EMBS Annual International Conference.
New York, USA, Aug. 30-Sept. 3.
Furui, S., 1981. Cepstral Analysis Technique for
Automatic Speaker Verification. IEEE Transactions
on Acoustics, Speech and Signal Processing. Vol. 29,
No. 2, pp 254-272, April.
Gavidia-Ceballos, Liliana and Hansen, John H. L., 1996.
Direct Speech Feature Estimation Using an Interactive
EM Algorithm for Vocal Fold Pathology Detection.
IEEE Trans. on Biomedical Engineering. Vol. 43, No.
4, April.
Godino-Llorente, J. I., Gomes-Vilda, P. and Blanco-
Velasco M., 2006. Dimensionality Reduction of a
Pathological Voice Quality Assessment System Based
on Gaussian Mixture Models and Short-Term Cepstral
Parameters. IEEE Transactions on Biomedical
Engineering. Vol. 53, No. 10, pp. 1943-1953, October,
Kay Elemetrics Corp. Disordered Voice Database, 1994.
Model 4337, 03 Ed.
Linde, Y., Buzo, A., and Gray, R. M., 1980. An Algorithm
for Vector Quantizer Design, IEEE Transaction on
Communications. Vol. COM-28, N0.I, pages 84-95,
January.
Marinaki, M., Contropoulos, C., Pitas, I., and Maglaveras,
N., 2004. Automatic Detection of Vocal Fold
Paralysis and Edema, Proc. of 8th Conf. Spoken
Language Processing (Interspeech 2004). Jeju, Korea,
October.
Murphy, Peter J. and Akande, Olatunji O., 2007. Noise
Estimation in Voice Signals Using Short-term
Cepstral, Journal of the Acoustical Society of America.
pp. 1679-1690, Vol. 121, No. 3, March.
O’Shaughnessy, Douglas, 2000. Speech Communications:
Human and Machine. 2nd Edition, NY, IEEE Press.
Parsa, Vijay and Jamieson, Donald G., 2001. Acoustic
Discrimination of Pathological Voice: Sustained
Vowels versus Continuous Speech. Journal of Speech,
Language, and Hearing Research. Vol. 44, pp 327–
339, April.
Quek, F., M. Harper, Haciahmetoglou, Y., Chen, L. and
Raming, L. O., 2002. Speech pauses and gestural
holds in Parkinson´s disease. Proceedings of
International Conference on Spoken Language
Processing. pp. 2485-2488.
Rabiner L. R. and Schafer R. W., 1978. Digital Processing
of Speech Signals. New Jersey: Prentice-Hall.
Shama, K., Krishna, A. and Cholayya, N. U., 2007. Study
of Harmonics-to-Noise Ratio and Critical-Band
Energy Spectrum of Speech as Acoustic Indicators of
Laryngeal and Voice Pathology. EURASIP Journal on
Advances in Signal Processing. Vol. 2007.
Umapathy, K., Krishnan, S., Parsa, V., and Jamieson D.,
2005. G. Discrimination of Pathological Voices Using
a Time-Frequency Approach.
IEEE Transactions on
Biomedical Engineering. Vol. 52, No. 3, March.
SHORT-TERM CEPSTRAL ANALYSIS APPLIED TO VOCAL FOLD EDEMA DETECTION
115