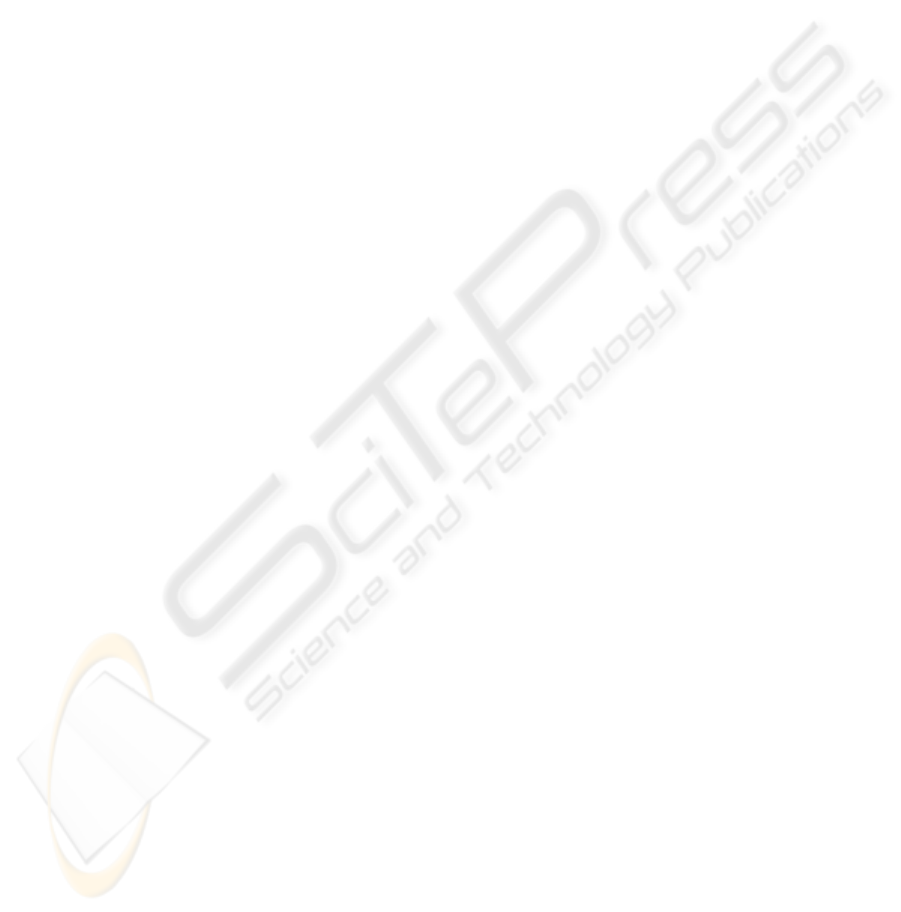
The time series novelty detection technique pre-
sented is general purpose and can be used for screen-
ing other anomalies. It has already been used to detect
ECG arrhythmias (Lemos et al., 2007).
The novelty detection technique proposed in this
work can be integrated on a physiological remote
monitoring system in order to reduce the amount of
data transmitted. Those systems are used to monitor
chronic patients biomedical signals ( ECG, breathing
frequency, temperature ). At patient’s location, some
sensors are used to read biomedical signals. These
signals are sent through a network to a remote station
where the data is stored and analysed by specialists
(Lin et al., 2004). The novelty detection technique
proposed can be used to filter the data that needs to be
sent to the remote station, where only data classified
as novelty is sent.
REFERENCES
AASM (1999). Sleep-related breathing disorders in adults:
recommendations for syndrome definition and mea-
surement techniques in clinical research. Sleep,
22:667–689. American Academy of Sleep Medicine
Task Force.
Box, G. E. P. and Jenkins, G. (1990). Time Series Analysis,
Forecasting and Control. Holden-Day, Incorporated.
Davis, M. and Vinter, R. (1985). Stochastic Modelling and
Control. Chapman Hall.
Goldberger, A. L., Amaral, L. A. N., Glass, L., Hausdorff,
J. M., Ivanov, P. C., Mark, R. G., Mietus, J. E., Moody,
G. B., Peng, C.-K., and Stanley, H. E. (2000). Phys-
ioBank, PhysioToolkit, and PhysioNet: Components
of a new research resource for complex physiologic
signals. Circulation, 101(23):e215–e220.
Guilleminault, C., Connolly, S., Winkle, R., Melvin, K., and
Tilkian, A. (1984). Cyclical variation of the heart rate
in sleep apnoea syndrome. mechanisms, and useful-
ness of 24 h electrocardiography as a screening tech-
nique. Lancet, 1:126–131.
Kay, S. M. (1993). Fundamentals of statistical signal pro-
cessing: detection theory. Prentice-Hall, Inc., Upper
Saddle River, NJ, USA.
Lemos, A. P., Tierra-Criollo, C. J., and Caminhas, W. M.
(2007). Ecg anomalies identification using a time se-
ries novelty detection technique. In IV Latin American
Congress on Biomedical Engineering.
Lin, Y.-H., Jan, I.-C., Ko, P., Chen, Y.-Y., Wong, J.-M., and
Jan, G.-J. (2004). A wireless pda-based physiological
monitoring system for patient transport. IEEE Trans-
actions on Information Technology in Biomedicine,
8(4):439–447.
Mietus, J. E., Peng, Ivanov, P., and Goldberger, A.
(2003). Hilbert transform based sleep apnea detec-
tion using a single lead electrocardiogram (apdet).
http://www.physionet.org/physiotools/apdet/.
Mietus, J. E., Peng, C. K., Ivanov, P. C., and Goldberger,
A. L. (2000). Detection of obstructive sleep apnea
from cardiac interbeat interval time series. Computers
in Cardiology, 27:753–756.
Penzel, T., McNames, J., de Chazal, P., Raymond, B., Mur-
ray, A., and Moody, G. (2002). Systematic compari-
son of different algorithms for apnoea detection based
on electrocardiogram recordings. Medical & Biologi-
cal Engineering & Computing, 40:402–407.
Schwarz, G. (1978). Estimating the dimension of a model.
The Annals of Statistics, 6:461–464.
Young, T., Palta, M., Dempsey, J., Skatrud, J., Weber,
S., and Badr, S. (1993). The occurence of sleep-
disordered breathing among middle-aged adults. New
England Journal of Medicine, 328:1230–1235.
SCREENING OF OBSTRUCTIVE SLEEP APNEA BY RR INTERVAL TIME SERIES USING A TIME SERIES
NOVELTY DETECTION TECHNIQUE
575