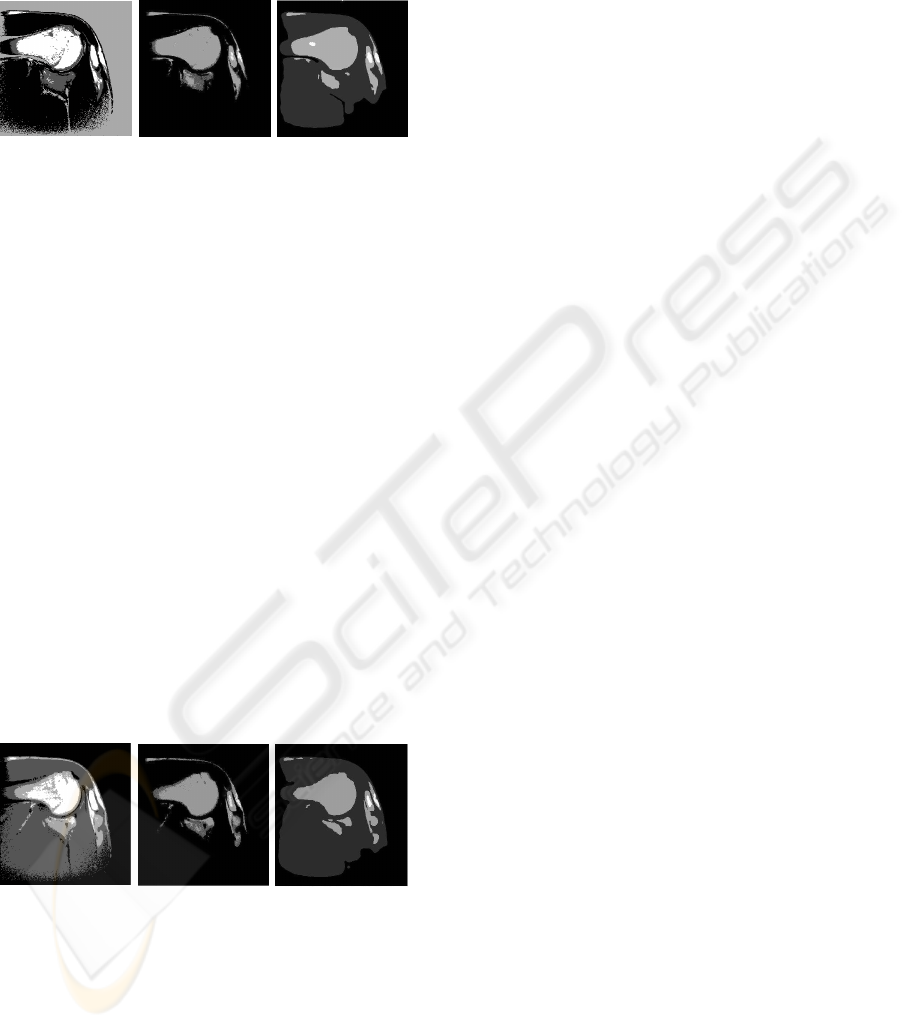
suggests convergence to the same limit solution. This
is indeed confirmed when the differences images are
computed and classical methods (first row) are com-
pared.
Figure 5: Slice 1. Segmentation image for two phases (4
classes) a) k-means b) Density mixture c) Active contours
without edges algorithms.
As aspected, some more differences between the
quality reconstruction of the different methods can
be appreciated in the multiphase (four classes) clas-
sification problem. In Figures 5-6 we report the re-
sults obtained with the classical (k-means) algorithm
(left), the bayesian mixture model (center) and the
Chan-Vese model (right). The greater tubercle and
the head of the humerus are properly classified and
shaped with our methods (center and right) while the
classical k-means fails in both aspects (and in both
slices, see Figures 5-6, on the left, where the bone
is under-estimated and muscle is wrongly detected).
Articular cartilage has been detected in (center and
right) but not in (left). Muscle is properly classified
with the Chan-Vese model (right) and the classical
method (left) but no classification has been done in
the bayesian approach where the background is as-
signed to the same class. At the same time the head of
the scapula has been properly classified in (right) but
not in (center) where the shape, nevertheless is cor-
rectly obtained. Notice also that the acromial process
has been characterized by the two methods.
Figure 6: Slice 2. Segmentation image for two phases (4
classes) a) k-means b) Density mixture c) Active contours
without edges algorithms.
4 CONCLUSIONS
We considered the problem of automatic segmenta-
tion of 2D images using an hybrid, statistical and
geometrical model based on Chan-Vese work. This
method provides correct classification of bony struc-
tures but soft tissues are not yet properly classified.
This is also manifested in the bayesian approach. The
differences between the results obtained with the two
methods suggest the conclusion that hybrid methods
can give better results as far as the right statistics are
included in the model and this will be the aim of our
future work.
ACKNOWLEDGEMENTS
This work was partially granted by ”Research on
Molecular and Multimodality Medical Imaging Net-
work” of the Carlos III Health Institute. Finally the
authors of the present work would like to thank Ru-
ber International Hospital and the neuroradiologist
Dr. Juan Linera.
REFERENCES
Biviji, A., Paiement, G., Davidsion, A., and Steinbach, L.
(2002). Musculoeskeletal manifestations of the hu-
man immunodeficiency virus infection. Of the Ameri-
can Academy of Orthopedic Surgeons, pages 10:312–
20.
Brinkmann, B. and Manduca, A. (1998). Optimized homo-
morphic unsharp masking for mr grayscale inhomo-
geneity correction. In IEEE transactions on medical
imaging, p. 62-66, volume 17.
Chan, T. F. and Vese, L. A. (2001). Active contours without
edges. ieee transactions on image processing. In IEEE
Transactions on Image Processing, volume 10.
Ehman, R., Megibow, A., MacCauley, T., Bluemke, D., and
Steinbach, L. (2001). Musculoskeletal imaging. In the
24th Annual Course of the Society of Computed Body
Tomography and Magnetic Resonance, Miami.
Johnson, R. and Steinbach LS, e. (2003). Essentials of mus-
culoskeletal imaging. american academy of orthope-
dic surgeons. Chicago.
Kass, M., WitKin, A., and Terzopoulos, D. (1987.). Snakes:
Active contour models. In Intl. J. Comput. Vision,
1:321-331.
Mignotte, M., Meunier, J., Soucy, J. P., and Janicki, C.
(2001). classification of brain spect images using 3d
markov random field and density mixture estimations.
5th world multi-conference on systemics, cybernetics
and informatics. In Concepts and Applications of Sys-
temics and Informatics, volume 10, pages 239–244,
Orlando.
Mumford, D. and Shah, J. (1989). Optimal approximation
by piecewise smooth functions and associated varia-
tional problems. In Communications on Pure Applied
Mathematics, p. 577-685, volume 42.
MRI SHOULDER COMPLEX SEGMENTATION AND CLASSIFICATION
17