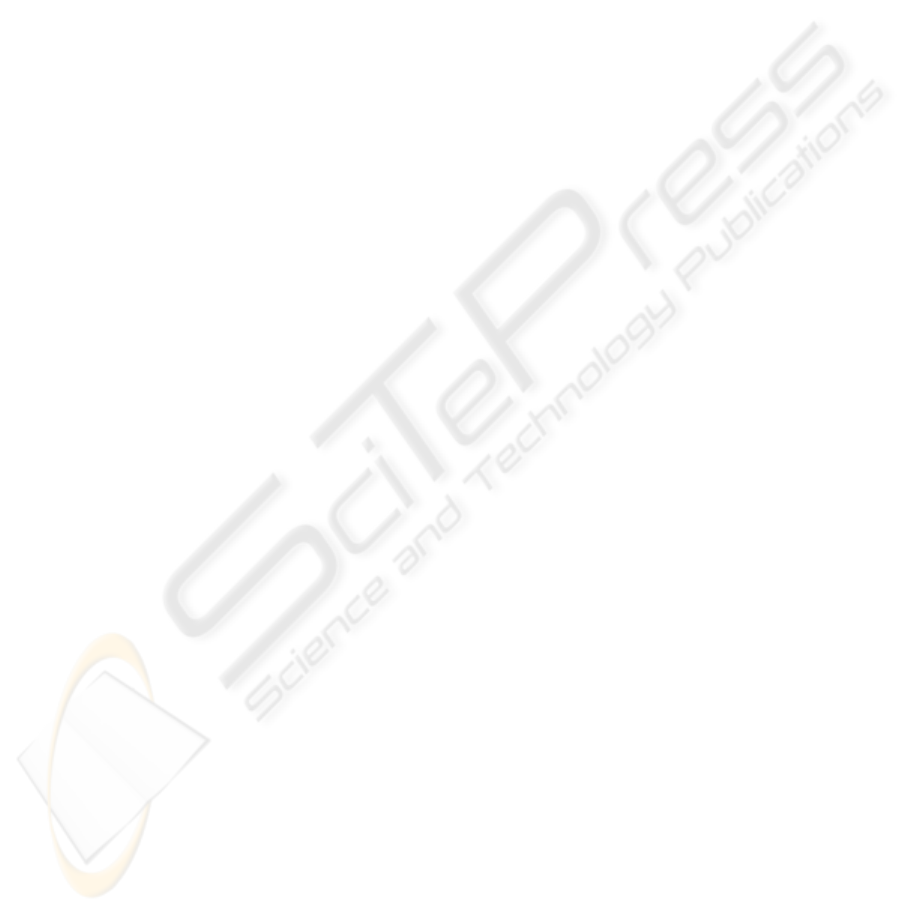
Further optimization can be made when the ratio
of F runs is much higher than G runs. Elements vis-
ited on the list that have reached a locally optimal po-
sition will remain in that position on the next run of
the speed function. Therefore they can be removed
from successive F runs on that pass.
As mentioned in their paper title, this method de-
parts almost entirely from the PDE based methods,
but maintains many of the advantages. Sub cell accu-
racy is not possible in this generic form, but it would
a simple addition to use this method as a prototype
generator for slower, but more accurate methods. The
inclusion of the φ embedding, albeit much simplified,
allows local topology to be determined at any point
to discrete-level accuracy. Thus, the Gaussian func-
tion has the information required to approximate the
’sharpness’ at a point, and to expand or contract as a
result (in a reasonable approximation to the effect of
curvature). The lack of the requirement to solve PDEs
holds several advantages. No timestep is required in
the update process - the algorithm is entirely discrete.
The method maintains the implicit ability of splits and
merges along the front, without relying on any gra-
dient calculation, eliminating the need for expensive
entropy-satisfying spatial derivative schemes.
Parameter selection for level set segmentation
generally requires at least some level of human in-
teraction. Specifically in the case of image segmen-
tation, initialization of a level set solver requires at
minimum seed locations, an ideal data value, and an
acceptable noise threshold to be preset. Also, differ-
ing data classes in the same problem space, for exam-
ple tissue types in medical scans, each require their
own level set surface with their own set of initializa-
tion parameters. Ideally the task of assigning seed
locations and calculating level set parameters would
be automated. These issues lend themselves well to
an agent based approach, which will be discussed in
the following section.
1.2 Multi-Agent Swarm Based
Algorithms
Agents are independent entities existing in some
environment - sensing, processing and modifying
the environment based on internal reasoning. For
multi-agent systems, the swarm intelligence and self-
organisation paradigms have become popularized in
many disciplines as an explanation for the apparent
mismatch in complexity of agent versus complexity
of task (Camazine et al., 2001), and as a unique engi-
neering metaphor (Bonabeau et al., 1999). Such sys-
tems of agents can focus on simple stimulus-response
functions using purely local stimuli with little or no
cognition or direct communication. Tasks are ac-
complished by exploiting the non-linearity inherent
in such a massively parallel system, rather than re-
lying on individual agent complexity. An agent mod-
ifying the environment at a particular location allows
another agent in that same location at a later time
to sense this new state and respond accordingly. In
this way the environment is used for indirect com-
munication, termed stigmergy. Stigmergy in bio-
logical systems is further enhanced by the complex-
ity of the environment, for example diffusion is uti-
lized in many biological processes to spread infor-
mation in the form of chemical gradients. Activa-
tion/attraction and inhibition/repulsion functions can
thus be designed to control agent interactions based
solely on local environmental state and any internal
state, relying on quantitative information reinforce-
ment and decay as well as qualitative signaling via the
environment to control the weighting between possi-
ble responses. A global-level task or structure may
then be many times more complex than an individual
can perceive or accomplish, yet through parallel ap-
plication of simple rules with indirect non-linear cou-
plings, we see the spontaneous emergence of a solu-
tion. Given this non-linearity, a tiny change in a pa-
rameter can result in a drastically different solution,
but (if the system is well formed) one that still con-
forms to a valid set of stable solutions - that is, the
system exhibits multistability. We have demonstrated
this engineering paradigm previously in modeling the
building behaviour of Macrotermes termites (Feltell
and Bai, 2004; Feltell et al., 2005). The use of an
attractive cement pheromone to coordinate soil clus-
tering behaviour has provided the inspiration for the
swarm-intelligent clustering mechanism used here.
Swarm based clustering algorithms have been de-
veloped to cluster sets of n-dimensional data, gen-
erally projected onto a 2D grid, by taking inspira-
tion from brood sorting and corpse clustering in ants
(Monmarche et al., 1999; Monmarche, 1999; Kanade
and Hall, 2003; Schockaert et al., 2004) as well as
building behaviour in termites (Vizine et al., 2005).
The approach does not need any prior knowledge of
the problem space or number of clusters, and as a
bonus gives a visual representation of the clusters on
the 2D grid.
Agent based approaches have also been devel-
oped to directly segment an image, again using in-
spiration from natural systems such as ant pheromone
trail networks (Ramos and Almeida, 2000), artificial
life (Liu et al., 1997; Liu and Tang, 1999; Bocchi
et al., 2005), social spiders (Bourjot et al., 2003), and
even termites, bloodhounds and children (Fledelius
and Mayoh, 2006). Here the agents interact directly
BIOSIGNALS 2008 - International Conference on Bio-inspired Systems and Signal Processing
212