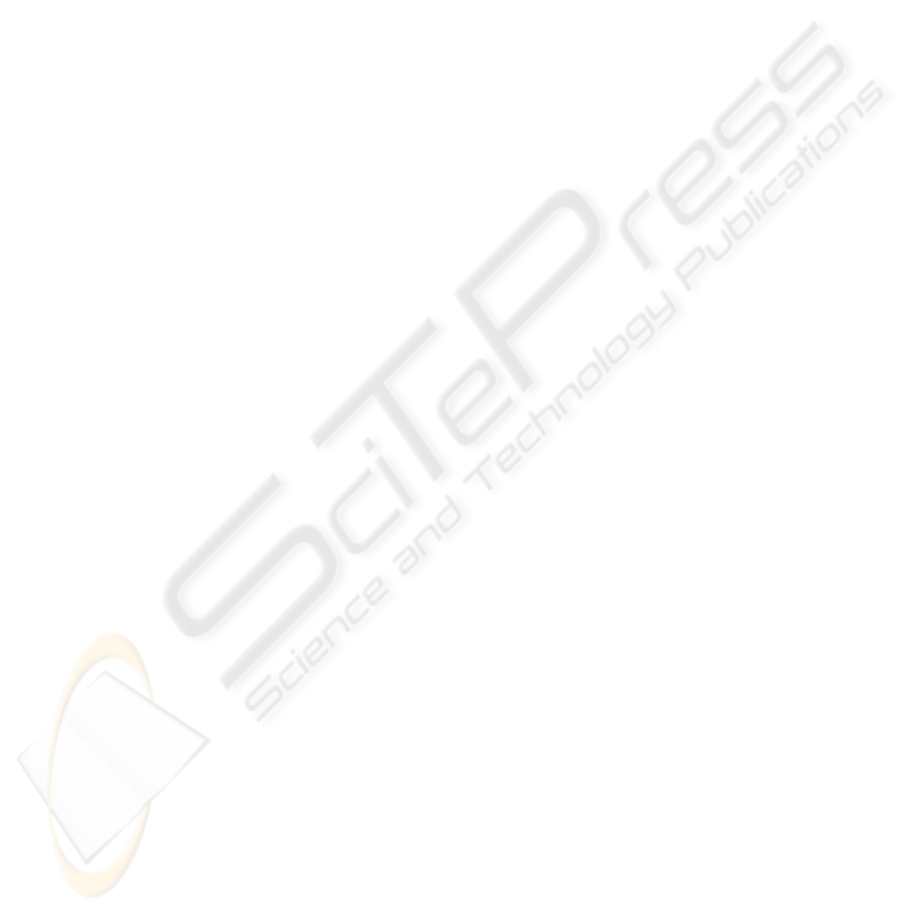
intervals between two spikes (Inter Spike Metric).
The movement cost q was set to 50 s
−1
– see (Vic-
tor and Purpura, 1997). A firing rate metric based on
the normalised mean squared error (NMSE) (Berry II
and Meister, 1998) was also applied. For that purpose,
the firing rates where estimated for both the real and
the estimated data, by convolving their PeriStimulus
Time Histogram (PSTH) (Berry II and Meister, 1998)
with a gaussian window of zero mean and 20ms of
standard deviation.
Cross-evaluation between the models’ responses
and the real ganglion cells responses using the de-
scribed error metrics are presented in Table 1. This
table shows the results when comparing the training
trial and the other trials. The presented error met-
rics confirm our analysis: the dynamic model is able
to capture the dynamics of real retina ganglion cells
which cannot be described by a simple set of a feed-
forward filter and a feedback filter. This can be seen
by noticing that the mean values for all error met-
rics are much lower for the dynamic model than for
the static model. The dynamic model also tends to
achieve a higher degree of variability than the static
model. However, this is due to the natural variability
of the real data.
In the presented work, the number of dynamic
components was fixed to five. However, for obtain-
ing a more general model, one could start by using a
larger number of dynamic components and then us-
ing feature selection – such as in (Tom´as and Sousa,
2007) – to remove all unnecessary components.
5 CONCLUSIONS
Many researchers tend to classify SLIF models and
Poisson-based models into different groups. How-
ever, as show in this paper, the former can be trans-
lated into the latest. One of the most important fea-
tures of the SLIF model is the presence of feed-
back mechanisms. Given that this property helps to
increase the spiking precision, we present a model
whose coefficients are dynamic in time. Moreover we
present a method to estimate its coefficients: it uses
eigen-analysis to estimate the initial parameters and a
gradient ascent technique for tuning the model. Pre-
sented results show that the proposed model is able to
achieve better results than the simpler static models.
REFERENCES
Akc¸ay, H. and Ninness, B. (1999). Orthonormal basis func-
tions for modelling continuous-time systems. Signal
Processing, 77(1):261–274.
Baccus, S. and Meister, M. (2002). Fast and Slow Contrast
Adaptation in Retinal Circuitry. Neuron, 36(5):909–
919.
Berry II, M. J. and Meister, M. (1998). Refractoriness and
neural precision. The Journal of Neuroscience.
Bialek, W. and van Steveninck, R. (2005). Features and
dimensions: Motion estimation in fly vision. Arxiv
preprint q-bio.NC/0505003.
Capela, S., Tom´as, P., and Sousa, L. (2007). Stochas-
tic integrate-and-fire model for the retina. In
15th European Signal Processing Conference (EU-
SIPCO’2007).
Chichilnisky, E. J. (2001). A simple white noise analysis of
neuronal light responses. Network: Computation in
Neural Systems, 12(2):199–213.
Gerstner, W., Jolivet, R., Brette, R., Clopath, C., Rauch, A.,
and Luscher, H. (2006). Predicting Neuronal Activ-
ity with Simple Models of the Threshold Type. Time
(msec), 100:50.
Keat, J., Reinagel, P., Reid, R. C., and Meister, M. (2001).
Predicting every spike: A model for the responses of
visual neurons. Neuron, 30:803–817.
Paninski, L. (2006). The most likely voltage path and
large deviations approximations for integrate-and-fire
neurons. Journal of Computational Neuroscience,
21(1):71–87.
Pillow, J. and Simoncelli, E. (2003). Biases in white noise
analysis due to non-Poisson spike generation. Neuro-
computing, 52(54):109–115.
Schwartz, O., Chichilnisky, E. J., and Simoncelli, E. P.
(2002). Characterizing neural gain control using
spike-triggered covariance. In Dietterich, T. G.,
Becker, S., and Ghahramani, Z., editors, Advances in
Neural Information Processing Systems, volume 14,
pages 269–276, Cambridge, MA. MIT Press.
Simoncelli, E. P., Paninski, L., Pillow, J., and Schwartz, O.
(2004). Characterization of Neural Responses with
Stochastic Stimuli, chapter 23, pages 327–338. MIT
Press.
Tom´as, P. and Sousa, L. (2007). Feature selection for the
stochastic integrate and fire model. In 2007 IEEE In-
ternational Symposium on Intelligent Signal Process-
ing (WISP’2007).
Victor, J. D. and Purpura, K. P. (1997). Metric-space analy-
sis of spike trains: theory, algorithms and application.
Computation Neural Systems, 8:127–164.
TOWARDS A UNIFIED MODEL FOR THE RETINA - Static vs Dynamic Integrate and Fire Models
533