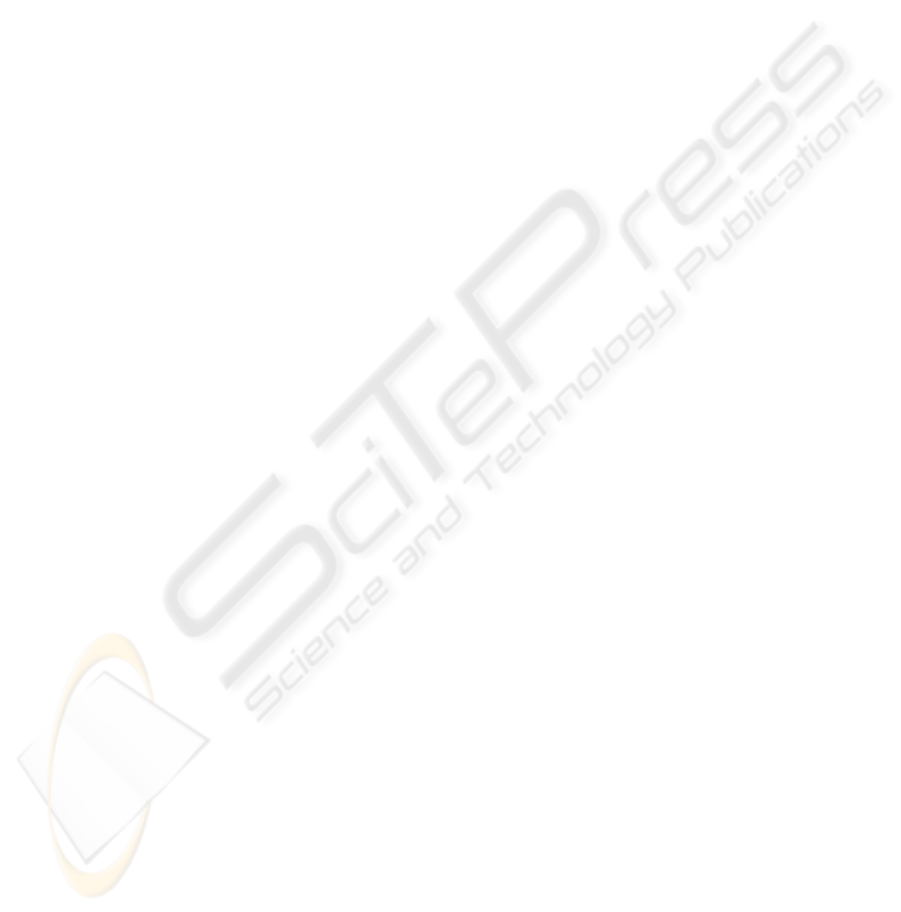
ject H, Isomap appears to recover sources whose acti-
vation better matches the reference function. For sub-
ject K, ICA alone appears to outperform the manifold
learning methods. For subjects S,T, and W, manifold
learning appears to generate better source separation.
5 DISCUSSION
In our method, we motivate manifold learning as a
pre-processing step to convolutive source separation
by appealing to the need for dimensionality reduc-
tion. The idea in using manifold learning to reduce di-
mensionality is that we can automatically identify the
voxels in the ROI that contain the most information
about the activation sequence of the area. Further-
more, the frequency space representation of voxels
results in much higher dimensionality; therefore, re-
ducing the dimensionality is critical to feasible com-
ponent analysis. The computational cost of filtering
unneeded dimensions at component analysis time is
far greater than at manifold learning time.
An additional side effect of manifold learning is
that we not only find features representing the acti-
vation in an area, but we also space the data along
these features so that we implicitly perform whiten-
ing of the data. In the normal use of time domain ICA
one explicitly performs PCA as a first step in order to
whiten the data. In the time domain this decorrelates
the data, making the source separation task return bet-
ter results.
We have shown improvement by using manifold
learning as a preprocessing step to complex source
separation. One benefit of this method is that the
reduced dimensionality representation requires less
computation by complex ICA. Furthermore, little
prior information is needed to define the ROI. These
results suggest that a more tightly integrated approach
would lead to better separation performance.
REFERENCES
Anemuller, J., Sejnowski, T., and Makeig, S. (2003). Com-
plex independent component analysis of frequency-
domain electroencephalographic data. Neural Net-
works, 16:1311–1323.
Belkin, M. and Niyogi, P. (2003). Laplacian eigenmaps
for dimensionality reduction and data representation.
Neural Computation, 15:1373–1396.
Bingham, E. and Hyvarinen, A. (2000). A fast fixed-
point algorithm for independent component analysis
of complex valued signals. International Journal of
Neural Systems, 10(1):1–8.
Coifman, R. R. and Lafon, S. (2006). Diffusion maps. Ap-
plied and Computational Harmonic Analysis, 21:5–
30.
Dogil, G., Ackerman, H., Grodd, W., Haider, H., Kamp,
H., Mayer, J., Reicker, A., and Wildgruber, D. (2002).
The speaking brain: a tutorial introduction to fmri ex-
periments in the production of speech, prosody, and
syntax. Journal of Neurolinguistics, 15(1):59–90.
Friston, K. (2003). Experimental design and statistical para-
metric mapping. In et al., F., editor, Human brain
function. Academic Press, 2nd edition.
Hurd, M. (2000). Functional neuroimaging motor study.
Josephs, O., Turner, R., and Friston, K. (1997). Event-
related fmri. Human Brain Mapping, 5(4):243–248.
McKeown, M. J., Makeig, S., Brown, G. G., Jung, T. P.,
Kindermann, S. S., Bell, A. J., and Sejnowski, T. J.
(1998). Analysis of fmri data by blind separation into
independent spatial components. Human Brain Map-
ping, 6:160–188.
Pederson, M. S., Larsen, J., Kjerns, U., and Parra, L. C.
(2007). Springer handbook on speech processing and
speech communication, chapter A survey on convolu-
tive blind source separation methods. Springer Press.
Postle, B. R., Berger, J. S., Taich, A. M., and D’Esposito,
M. (2000). Activity in human frontal cortex associated
with spatial working memory and saccadic behavior.
Journal of Cognitive Neuroscience, 12 Supp. 2:2–14.
Roweis, S. and Ghahramani, Z. (1999). A unifying re-
view of linear gaussian models. Neural Computation,
11:305–345.
Roweis, S. and Saul, L. (2000). Nonlinear dimensional-
ity reduction by locally linear embedding. Science,
290(5500):2323–2326.
Shen, X. and Meyer, F. G. (2006). Nonlinear dimension
reduction and activation detection for fmri dataset. In
IEEE, editor, Proceedings of 2006 conference on com-
puter vision and pattern recognition workshop. IEEE.
Tenenbaum, J., de Silva, V., and Langford, J. (2000). A
global geometric framework for nonlinear dimension-
ality reduction. Science, 290(5500):2319–2323.
DIMENSIONALITY REDUCTION FOR IMPROVED SOURCE SEPARATION IN FMRI DATA
313