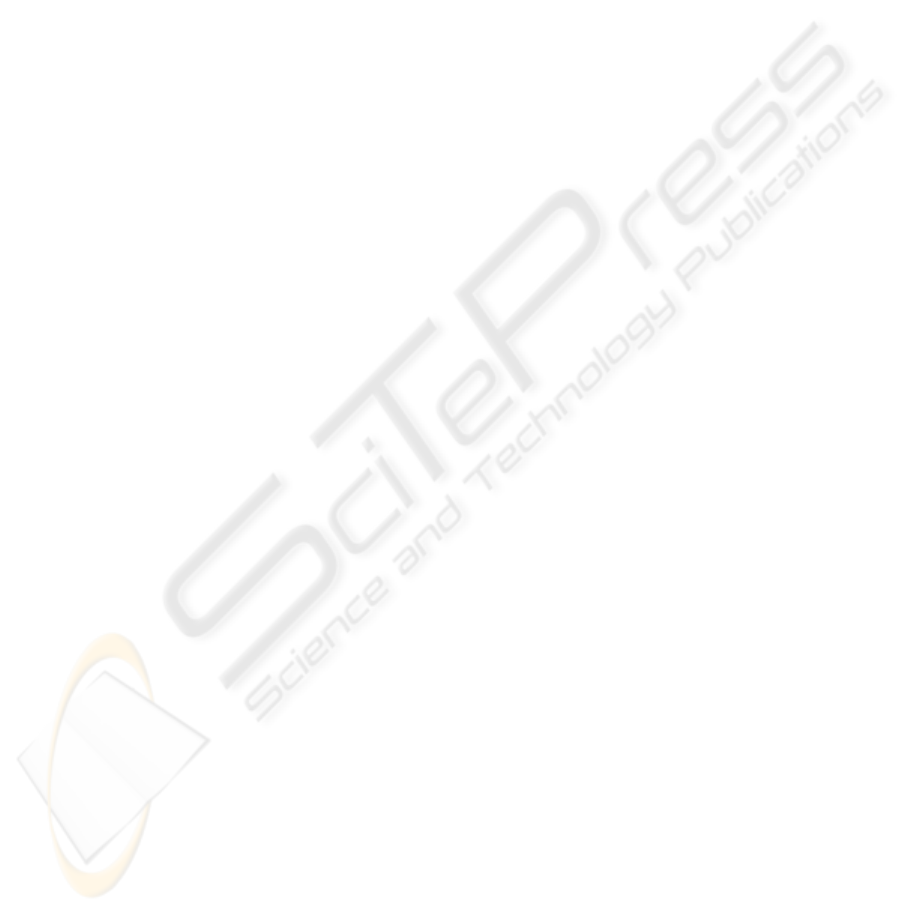
order to analyze the pathology dynamics and devel-
opment.
We described the original processing steps we
designed. Finally, we carried out an extensive ex-
perimental evaluation on a large set of heterogene-
ous images that demonstrated the high accuracy
achievable by the proposed technique (90% on aver-
age) compared to a more traditional approach based
on Support Vector Machines (SVM).
As future work, we will compare the proposed ap-
proach to artificial neural networks (ANN), and we
will eventually study the possibility of their integra-
tion.
ACKNOWLEDGEMENTS
We acknowledge the Dep. of Pathology of the
S.Luigi Hospital of Orbassano in Turin, Italy, for
providing IHC images and for the helpful and stimu-
lating discussions.
REFERENCES
Angelini, E, Campanini, R., Iampieri, E., Lanconelli, N.
Masotti, M., Roffilli, M., 2006. Testing the perform-
ances of different image representation for mass clas-
sification in digital mammograms. Int. J. Mod. Phys.
17(1):113-131.
Anguita, D., Boni, A., Ridella, S., Rivieccio F., Sterpi, D.,
2005. Theoretical and Practical Model Selection
Methods for Support Vector Classifiers. Springer,
Studies in Fuzziness and Soft Computing, Support
Vector Machines: Theory and Application.
Brey, E.M., Lalani, Z., Hohnston, C., Wong, M., McIntire,
L.V., Duke, P.J., Patrick, C.W., 2003. Automated se-
lection of DAB-labeled tissue for immunohistochemi-
cal quantification. In J. Histochem. Cytochem., 51(5),
pp.575-584.
Cai, C.Z., W.L.Wang, Y.Z: Chen, 2003. Int.J.Mod.Phys.
14:575.
Demandolx D, Davoust J. , 1997. Multiparameter image
cytometry: from confocal micrographs to subcellular
fluorograms. Bioimaging. 4:159-169.
Dybowski R., 2000. Neural computation in medicine:
perspectives and prospects. Proc. ANNIMAB-1. pp.
27-36.
E. Osuna, R. Freund, F. Girrosi, 1997. Training Support
Vector Machines: an Application to Face Detection.
IEEE Computer Society Conference on Computer Vi-
sion and Pattern Recognition (CVPR'97). pp. 130.
Ficarra, E., Macii, E., De Micheli, G., 2006. Computer-
aided evaluation of protein expression in pathological
tissue images. In Proc. of IEEE CBMS‘06., pp.413-
418.
Jain, A.K., Dubes, R.C., 1988. Algorithms for clustering
data, Prentice Hall.
Landini, G., 2007. Software, http://www.dentistry.bham.ac
.uk/landinig/software/software.html
Malpica N, de Solorzano CO, Vaquero JJ, Santos A,
Vallcorba I, Garcia-Sagredo JM, del Pozo F, 1997.
Applying watershed algorithms to the segmentation of
clustered nuclei. Cytometry. 28(4): 289-297.
Muller K.R:, S. Mika,G. Ratsch, K. Tsuda, 2001. IEEE
Trans. Neural Networks, 12:181.
Nattkemper, T.W., 2004. Automatic segmentation of digi-
tal micrographs: A survey. Medinfo, 11(Pt 2):847-51.
Nedzved A, Ablameyko S, Pitas I., 2000. Morphological
segmentation of histology cell images. ICPR. 1:500-3.
Platt, J., 1999. Fast training of support vector machines
using sequential minimal optimization. In Scholkopf,
B., Advances in kernel methods-support vector learn-
ing. MIT Press, Cambridge, MA, USA.
Rasband, W.S., ImageJ, U. S. National Institutes of
Health, Bethesda, Maryland, USA, rsb.info.nih.gov/ij/.
Ruifrok, A.C., Johnston, D.A., 2001. Quantification of
histochemical staining by color deconvolution. In
Anal.Quant.Cytol.Histol., 23(4), pp.291-299.
Ruifrok, A.C., Katz, R., Johnston, D., 2004. Comparison
of quantification of histochemical staining by Hue-
Saturation-Intensity (HSI) transformation and color
deconvolution. In Appl. Immunohisto. M. M., 11(1),
pp.85-91.
Sacha, J., K-means clustering, http://ij-plugins.sourceforge
.net/plugins/clustering/index.html.
Statnikov, A., Aliferis, C.F., Tsamardinos, I., Hardin, D.,
Levy, S., 2005. A comprehensive evaluation of multi-
category classification methods for microarray gene
expression cancer diagnosis. In Bioinformatics, 21(5),
pp.631-643.
Taneja, T.K., SK.Sharma Markers of small cell lung can-
cer. World Journal of Surgical Oncology, Vol(2):10.
Vapnik, V., 1998. Statistical learning theory, Wiley-
Interscience, New York, NY, USA.
Wang, L., 2004. Support vector machines: theory and
applications, Springer.
FULLY-AUTOMATED SEGMENTATION OF TUMOR AREAS IN TISSUE CONFOCAL IMAGES - Comparison
between a Custom Unsupervised and a Supervised SVM Approach
123