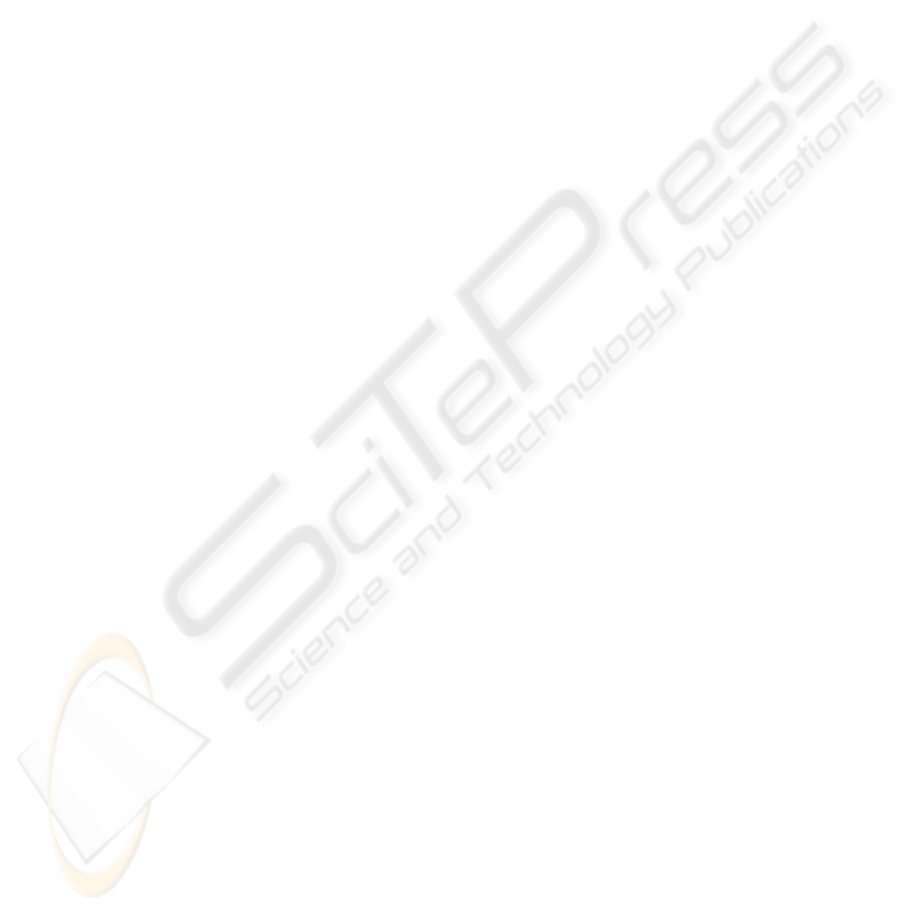
ing, synaptic strength, and postsynaptic cell type. The
Journal of Neuroscience, 18(24):10464–10472.
Connors, B. and Gutnick, M. (1990). Intrinsic firing pat-
terns of diverse neocortical neurons. Trends in Neuro-
sciences, 13:99–104.
Destexhe, A., Mainen, Z., and Sejnowski, T. J. (1994). An
efficient method for computing synaptic conductances
based on a kinetic model of receptor binding. Neural
Computation, 6:10–14.
Feldman, D. E. (2000). Timing-based LTP and LTD at ver-
tical inputs to layer II/III pyramidal cells in rat barrel
cortex. Neuron, 27:45–56.
Froemke, R. C. and Dan, Y. (2002). Spike-timing-
dependent plasticity modification induced by natural
spike trains. Nature, 416:433–438.
Froemke, R. C., Poo, M., and Dan, Y. (2005). Spike-
timing-dependent plasticity depends on dendritic lo-
cation. Nature, 434:221–225.
Hebb, D. O. (1949). The Organization of Behaviour. John
Wiley & Sons.
Hines, M. L. and Carnevale, N. T. (1997). The neuron sim-
ulation environment. Neural Computation, 9:1179–
1209.
Hodgkin, A. L. and Huxley, A. F. (1952). A quantitative
description of membrane current and its application to
conduction and excitation in nerve. Journal of Physi-
ology, 117:500–544.
Kepecs, A., van Rossum, M. C. W., Song, S., and Tegner,
J. (2002). Spike timing dependent plasticity: common
themes and divergent vistas. Biological Cybernetics,
87:446–458.
Le Masson, G., Renaud-Le Masson, S., Debay, D., and Bal,
T. (2002). Feedback inhibition controls spike transfer
in hybrid thalamic circuits. Nature, 417:854–858.
Lewis, N., Bornat, Y., Alvado, L., Lopez, C., Daouzli, A.,
Levi, T., Tomas, J., Saghi, S., and Renaud, S. (2006).
Pax : un outil logiciel / mat´eriel d’investigation pour
les neurosciences computationnelles. In NeuroComp,
pages 171–174.
Magee, J. C. and Johnston, D. (1997). A synaptically con-
trolled, associative signal for hebbian plasticity in hip-
pocampal neurons. Science, 275:209–213.
Markram, H., Lubke, J., Frotscher, M., and Sackmann,
B. (1997). Regulation of synaptic efficacy by co-
incidence of postsynaptic APs and EPSPs. Science,
275:213–215.
Renaud, S., Tomas, J., Bornat, Y., Daouzli, A., and Saighi,
S. (2007). Neuromimetic ICs with analog cores: an
alternative for simulating spiking neural networks. In
InternationaI Symposium on Circuits And Systems,
pages 3355–3358. IEEE.
Roberts, P. D. and Bell, C. C. (2002). Spike timing depen-
dent synaptic plasticity in biological systems. Biolog-
ical Cybernetics, 87:392–403.
Rumsey, C. C. and Abbott, L. F. (2003). Equalization of
synaptic efficacy by activity- and timing-dependent
synaptic plasticity. The Journal of Neurophysiology,
91:2273–2280.
Singer, W. and Gray, C. M. (1995). Visual feature integra-
tion and the temporal correlation hypothesis. Annual
Review of Neuroscience, 18:555–586.
Song, S. and Abbott, L. (2001). Cortical development and
remapping through spike timing-dependent plasticity.
Neuron, 32:339–350.
Song, S., Miller, K., and Abbott, L. (2000). Competi-
tive hebbian learning through spike-timing-dependent
synaptic plasticity. Nature Neuroscience, 3:919–926.
van Rossum, M. C. W., Bi, G.-Q., and Turrigiano., G.
(2000). Stable hebbian learning from spike timing-
dependent plasticity. The Journal of Neuroscience,
20:8812–8821.
van Rossum, M. C. W. and Turrigiano, G. (2001). Cor-
relation based learning from spike timing dependent
plasticity. Neurocomputing, 38-40:409–415.
Zou, Q. (2006). Computational models of spike timing
dependent plasticity: synapses, neurons and circuits.
PhD thesis, Universit´e Paris VI.
Zou, Q., Bornat, Y., Sa¨ıghi, S., Tomas, J., Renaud, S., and
Destexhe, A. (2006a). Analog-digital simulations of
full-conductance-based networks of spiking neurons
with spike timing dependent plasticity. Network: com-
putation in neural systems, 17:211–233.
Zou, Q., Bornat, Y., Tomas, J., Renaud, S., and Destexhe,
A. (2006b). Real-time simulations of networks of
hodgkin-huxley neurons using analog circuits. Neu-
rocomputing, 69:1137–1140.
Zou, Q. and Destexhe, A. (2007). Kinetic models of spike-
timing dependent plasticity and their functional con-
sequences in detecting correlations. Biol. Cybern.,
97(1):81–97.
WEIGHTS CONVERGENCE AND SPIKES CORRELATION IN AN ADAPTIVE NEURAL NETWORK
IMPLEMENTED ON VLSI
291