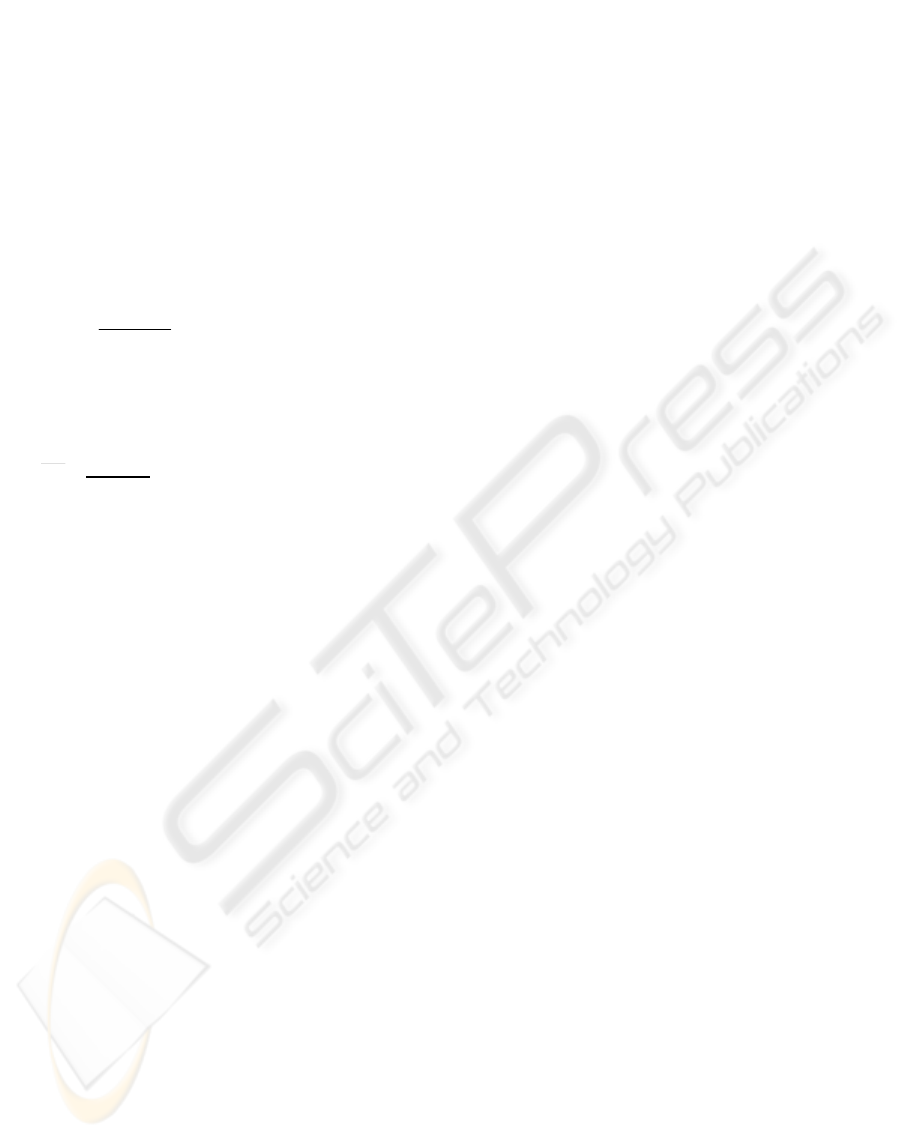
14
104,102106,406
−−
×× +v=t
a1p1
(s)
(1)
0,0680,967
a2p2
v=t (s)
(2)
Using these equations we can obtain estimates of
the loft time (t
pi
) from the variables measured from
the accelerometer signal (v
ai
).
The loft time relative error associated with each
of the algorithms i was determined for each jump j
(δ
εij
), taking as “real” loft times the values measured
from the force platform signal(t
pj
).
(
)
1,2 1,... 60
pp
jij
ε
ij
p
j
tt
δ =,i=;j=,
t
−
(3)
The accuracy of the algorithms was assessed by
determining the corresponding average loft time
relative errors:
δ
εi
=
∑
δ
εij
n
,i= 1,2 j=1,..., 60
(4)
The results led to relative errors of 7,0% for the
first algorithm and 2,9% for the second algorithm
Taking as reference the mean loft time determined
for the set of 60 jumps with the regression equations
(1) and (2) these relative errors correspond to 32 ms
and 13 ms, respectively.
Both algorithms are also affected by a common
base error of 0.1% which is characteristic of the
acquisition unit and inversely proportional to its
sampling rate.
Usually, when the force platform is used to
determine the loft time an associated error of 0,5% is
introduced because the algorithm is susceptible of
the parameters chosen by the user as the initial and
final points of the flight stage. In contrast, the
algorithms we propose are automatic.
5 CONCLUSIONS
The time interval between the minimum acceleration
value of the flying stage and the maximum
acceleration value of the landing stage is the best of
the two devised measures, showing a good
correlation with the real loft times (r=0.933 and
δ
ε
.=2,9%).
Although associated with errors, these
preliminary results indicate that these algorithms are
good alternative methods for the computation of loft
time, taking advantage of the use of an
accelerometer instead of a force platform, which is
more expensive and less portable.
In addition to the flight time other parameters
used to assess the performance of the jump can be
found on the acceleration signal, such as the height
of the jump. Furthermore, information on the
dynamic behaviour of the jumper, namely during the
flight stage can also be obtained from the
acceleration signal, which is impossible to study
with only the vertical force signal.
In the future, we plan to study the load
distribution between inferior members during the
take-off and landing stages by combining
acceleration and force analysis and study the on-
flight behaviour of the jumper.
REFERENCES
Dowling J., Vamos, L., 1993, J., Identification of Kinetic
and Temporal Factors Related to Vertical Jump
Performance, Applied Biomechanics, 9.
Linthorne, N. P., 2001, Analysis of standing vertical jumps
using a force platform, Am. Journal of Phys., 69 (11).
Hassan R., Begg R. K., Khandoker A. H., Stokes R., 2006,
Automated Recognition of Human Movement in Stress
Situations, Proceedings of the 2
nd
Internationsl
Workshop on Biosignal Procesing and Classification –
BPC 2006, INSTICC Press.
Silva H., Gamboa H., Viegas V., Fred A., 2005, Wireless
Physiologic Data Acquisition Platform., 2005.
Proceedings of the 5
th
Conference on
Telecomunications Confele.
http://www.plux.info
http://www.amtiweb.com/bio/force_platforms.htm
Proakis J. G., Manolakis D., 1995, Digital Signal
Processing: Principles, Algorithms and Applications,
Pearson US Import & PHIPEs, 3
rd
International
Edition.
BIOSIGNALS 2008 - International Conference on Bio-inspired Systems and Signal Processing
396