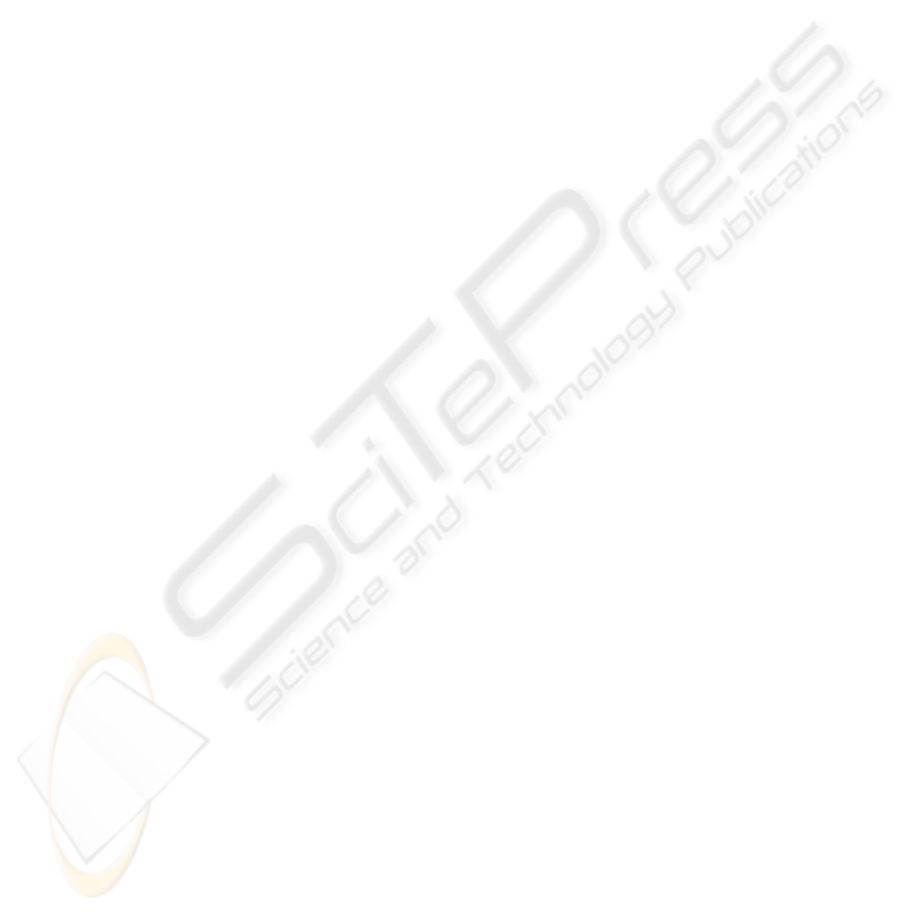
duces the initial set of features to a subset of features
which is unique for each user. Application of KNN
classifier to the selected set of features for the respec-
tive users decides the authenticity of the user identity
claim.
The results show that the proposed technique
could be a competitive biometric technique which
minimizes the rate at which an imposter bypasses the
authentication system. Apart from that, as mentioned
earlier, this technique does not require any additional
hardware. The existent hardware namely keyboard is
sufficient for this technique which makes it inexpen-
sive.
The open password approach followed enabled a
more complete study given that we had access to more
impostor data, and validated the possibility of using a
known sentence.
REFERENCES
Bartlow, N. and Cukic, B. (2006). Evaluating the reliabil-
ity of credential hardening through keystroke dynam-
ics. In ISSRE ’06: Proceedings of the 17th Interna-
tional Symposium on Software Reliability Engineer-
ing, pages 117–126, Washington DC.
Bergadano, F., Gunetti, D., and Picardi, C. (2002). User au-
thentication through keystroke dynamics. ACM Trans.
Inf. Syst. Secur., 5(4):367–397.
de Magalhaes, S. T., Revett, K., and Santos, H. M. D.
(2005). Password secured sites: Stepping forward
with keystroke dynamics. In NWESP ’05: Proceed-
ings of the International Conference on Next Gener-
ation Web Services Practices, page 293, Washington,
DC, USA. IEEE Computer Society.
Duda, R. O., Hart, P. E., and Stork, D. G. (2000). Pattern
Classification. Wiley-Interscience Publication.
Friant, D. (2006). Keystroke dynamics format for data in-
terchange. Technical Report INCITS M1/06-0268,
InterNational Committee for Information Technology
Standards.
Fukunaga, K. (1990). Introduction to statistical pattern
recognition. Academic Press Professional, Inc., San
Diego, CA, USA.
Gamboa, H. and Ferreira, V. (2003). Widam - web in-
teraction display and monitoring. In 5th Interna-
tional Conference on Enterprise Information Systems,
ICEIS’2003, pages 21–27, Anger, France. INSTICC
Press.
Gamboa, H., Fred, A., and Jain, A. (2007). Webbiometrics:
User verification via web interaction. In Biometrics
Symposium, BCC, Baltimore, USA.
Hocquet, S., Ramel, J.-Y., and Cardot, H. (2005). Fusion
of methods for keystroke dynamic authentication. au-
toid, 0:224–229.
Jain, A., Ross, A., and Prabhakar, S. (2004). An introduc-
tion to biometric recognition. Circuits and Systems for
Video Technology, IEEE Transactions on, 14(1):4– 20.
Jain, A. K. and Ross, A. (2002). Learning user-specific pa-
rameters in a multibiometric system. In Proc. of Inter-
national Conference on Image Processing (ICIP).
Jiang, C.-H., Shieh, S., and Liu, J.-C. (2007). Keystroke
statistical learning model for web authentication. In
ASIACCS ’07: Proceedings of the 2nd ACM sympo-
sium on Information, computer and communications
security, pages 359–361, New York, NY, USA. ACM
Press.
Modi, S. K. (2005). Keystroke dynamics verfication using
spontaneous password. Master’s thesis, Purdue Uni-
versity.
Monrose, F. and Rubin, A. D. (2000). Keystroke dynamics
as a biometric for authentication. Future Generation
Computer Systems, 16(4).
Revett, K., de Magalh˜aes, S. T., and Santos, H. M. D.
(2006). Enhancing login security through the use of
keystroke input dynamics. In Advances in Biomet-
rics, International Conference, ICB 2006, Hong Kong,
China, January 5-7, 2006, Proceedings, pages 661–
667.
Shepherd, S. J. (1995). Continuous authentication by anal-
ysis of keyboard typing characteristics. In European
Convention on Security and Detection (ECOS 95),
pages 111–114.
Siedlencki, W. and Sklansky, J. (1993). On automatic fea-
ture selection. In Chen, C. H., Pau, L. F., and Wang,
P. S. P., editors, Handbook of Pattern Recognition and
Computer Vision. World Scientific.
Silva, H. (2007). Feature selection in pattern recognition
systems. Master’s thesis, UNIVERSIDADE T´ecnica
de Lisboa, Instituto Superior T´ecnico.
BIOSIGNALS 2008 - International Conference on Bio-inspired Systems and Signal Processing
550