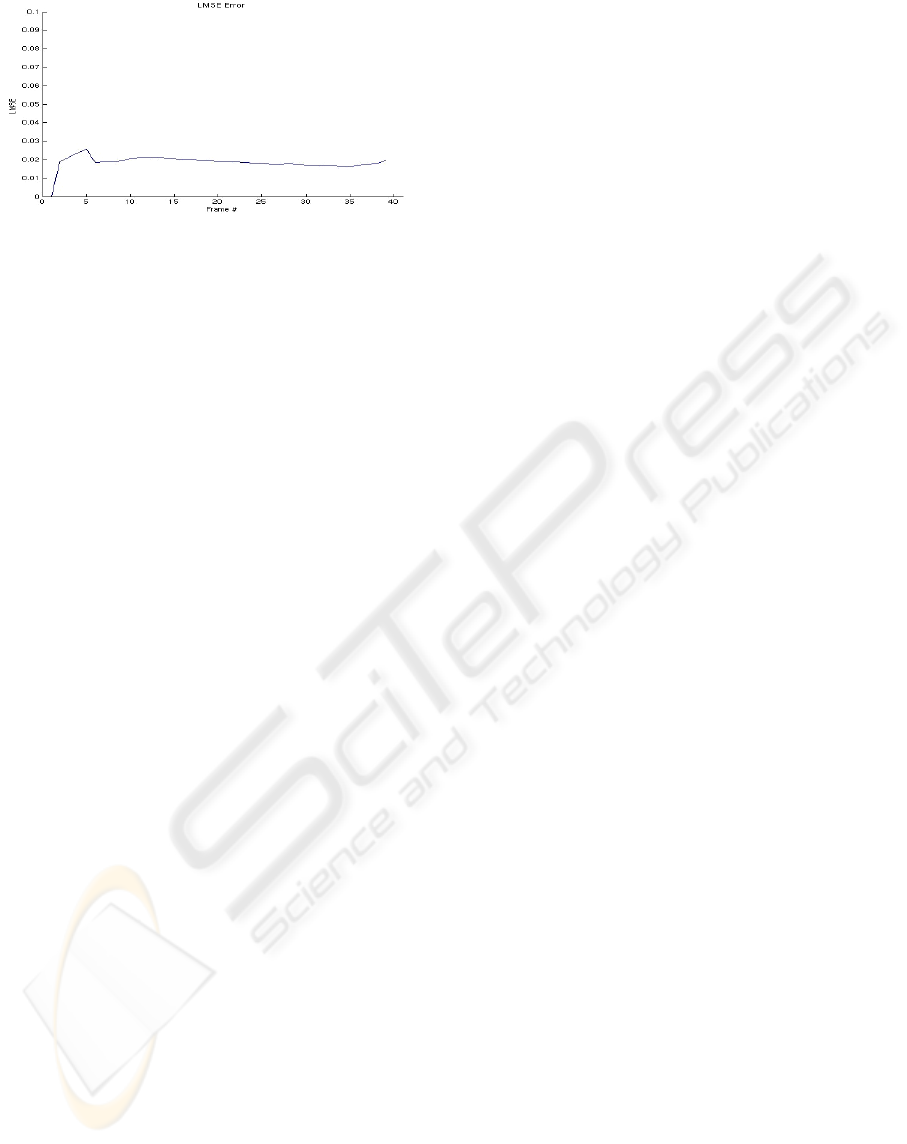
Figure 6: LMSE results for the Rheinhafen database.
4 CONCLUSIONS
This work has the objective of predicting mobile
objects in video scenes as the camera or sensory
device mounted on a platform remains stationary.
Unlike existing target detection and tracking
research, it makes use of Gabor filtering (and
boundary box method) to select the ROI and a
nonlinear extended Kalman filtering as a feedback
mechanism to accurately track the moving targets
and predict their locations ahead of time. The
reported experimental results demonstrate that the
nonlinear Kalman filtering based scene prediction
performs well and can accurately estimate the next
frames in images to a certain degree of accuracy.
The low LMSE error measurement of the nonlinear
filter prediction, on the average of about 2 to 3 %,
proves the reliability and robustness of this approach
to time-varying image data processing. The
presented results are reasonably low in error for low-
cost visible and IR camera applications [17, 21].
Potential areas for future research lie in devising an
ROI tracking mechanism in lieu of semantic
information and improvements to the Kalman
filtering algorithm to adjust itself for high-level
visual clues. The magnitude of the prediction error
involving initial frames indicates that further work is
needed for the performance improvement.
REFERENCES
Bramberger, M., et al. (2004) Real-time video analysis on
an embedded smart camera for traffic surveillance. In
Proc. of 10
th
IEEE RTAS Symp., pp. 174-181.
Celenk, M., et al., (2007a) A Kalman filtering approach to
3-D IR scene prediction using single-camera range
video,” in Proc. IEEE ICIP, San Antonio, TX.
Celenk, M.,et al. (2007b) Non-linear IR scene prediction
for range video surveillance. In 4
th
Joint IEEE Int.
Workshop on OTCBVS’0), Minneapolis, MN
Cheung, S.Y., et al., (2005) Traffic surveillance with
wireless magnetic sensors. In Proc. 12th ITS World
Congress, San Francisco, Nov. 2005.
Dorfmüller-Ulhaas, K. (2003) Robust optical user motion
tracking using a Kalman filter. Technical Report.
University of Augsburg, May 2003.
Hoover A., et al., (2003) Egomotion estimation of a range
camera using the space envelope. IEEET-SMCB,
33(4), pp. 717-721.
Huang, T. and Russell, S. (1998) Object identification: A
Bayesian analysis with application to traffic
surveillance. Artificial Intel., 103(1), pp. 77-93.
Institute for Algorithms and Cognitive Systems, Image
Sequence Server, Karlsruhe University,
<http://i21www.ira.uka.de/image_sequences >.
Irani, M., and Anandan P. (1998) A unified approach to
moving object detection in 2D and 3D scenes. IEEET-
PAMI, 20(6), pp. 577-589.
Julier, S. J. and Uhlmann, J. K. (1997) A new extension of
the Kalman filter to nonlinear systems. In Proc. of
AeroSense, Orlando, FL
Kim, J. and Woods, J.W. (1998) 3-D Kalman filter for
image motion estimation. IEEET-IP, 7(1), pp. 42-52.
Koller, D., et al,. (1994) Towards robust automatic traffic
scene analysis in real-time. In Proc. IAPR.
Macenko, M., et al., (2007) Lesion detection using Gabor-
based saliency field mapping. Medical Imaging 2007,
Proc. SPIE Vol. 6512, Feb. 17-24, San Diego, CA.
Maire, M. and Kamath, C. (2005) Tracking Vehicles in
Traffic Surveillance Video. Lawrence Livermore
National Lab., Technical Report UCRL-TR-214595.
Roumeliotis, S. I. and Bekey, G. A. (2000a) SEGMENTS:
A Layered, Dual-Kalman filter Algorithm for Indoor
Feature Extraction. Proc. IROS 2000, pp. 454-461.
Roumeliotis, S. I. and Bekey, G. A. (2000b) Bayesian
estimation and Kalman filtering: A unified framework
for Mobile Robot Localization. Proc. IEEE Conf.
Robotics and Autom., pp. 2985-2992.
Sawhney, H.S, et al., (2000) Independent motion detection
in 3D scenes. IEEET- PAMI, 22(10), pp. 1191-1199.
Sorenson, H. W. (1985) Kalman Filtering: theory and
application. IEEE Press, 1985.
Theodoridis, S. and Koutroumbas, K. (2006) Pattern
Recognition. 3
rd
ed., Academic Press.
Wang, Y., et al. (2006) Renaissance: A real-time freeway
network traffic surveillance tool. In IEEE ITSC '06.
VISAPP 2008 - International Conference on Computer Vision Theory and Applications
622