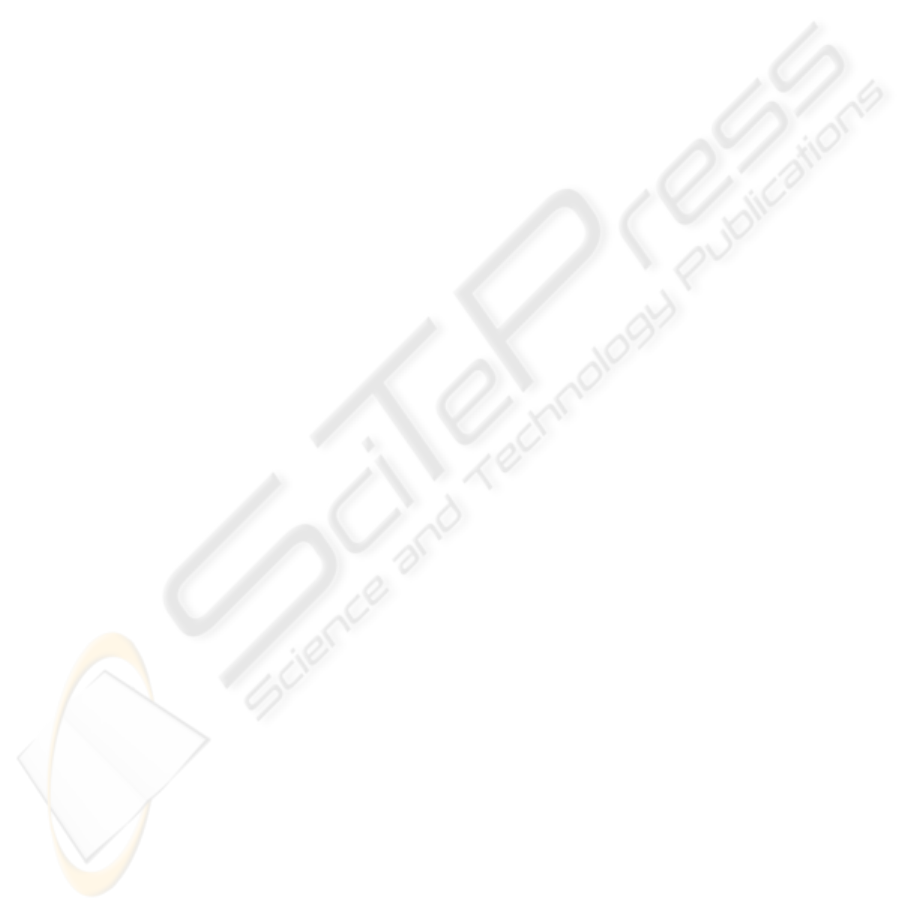
the novel used diffusion filter approaches could out-
perform the known algorithms with better and more
stable recognition results.
We showed also that the algorithms which are
closest to the visual perception could return the best
results.
Based on that first evaluation results further inves-
tigation in diffusion filters for illumination normaliza-
tion is definitely reasonable. Especially the diffusion
tensor methods offer a lot of opportunities to improve
the recognition results.
ACKNOWLEDGEMENTS
Parts of the presented research were realized within an
ongoing partnership with the MAGIX AG. The pub-
lication was supported by grant No. 01MQ07017 of
the German THESEUS program.
REFERENCES
Adini, Y., Moses, Y., and Ullman, S. (1997). Face recog-
nition: The problem of compensating for changes in
illumination direction. IEEE Transactions on Pattern
Analysis and Machine Intelligence, 19(7):721–732.
Belhumeur, P. N., Hespanha, J. P., and J.Kriegman, D.
(1997). Eigenfaces vs. fisherfaces: Recognition us-
ing class specific linear projection. IEEE Transac-
tions on Pattern Analysis and Machine Intelligence,
19(7):711–720.
Cohen, M. A. and Grossberg, S. (1984). Neural dynamics
of brightness perception: Features, boundaries, diffu-
sion, and resonance. Perception and Psychophysics,
36(5):428–456.
Georghiades, A. S., Belhumeur, P. N., and Kriegman, D. J.
(2001). From few to many: Illumination cone models
for face recognition under variable lighting and pose.
IEEE Transactions on Pattern Analysis and Machine
Intelligence, 23(6):643–660.
Gross, R. and Brajovic, V. (2003). An image preprocess-
ing algorithm for illumination invariant face recog-
nition. 4th International Conference on Audio- and
Video-Based Biometric Person Authentication, pages
10–18.
Jobson, D. J. and Woodell, G. A. (1995). Properties
of a center/surround retinex: Part 2 - surround de-
sign. Technical report, NASA Technical Memoran-
dum 110188.
Koenderink, J. (1984). The structure of images. Biological
cybernetics, pages 363–370.
Land, E. H. (1977). The retinex theory of color vision.
Scientific American, 237(6):108–120, 122–123, 126,
128.
Perona, P. and Malik, J. (1990). Scale-space and edge de-
tection using anisotropic diffusion. IEEE Transac-
tions on Pattern Analysis and Machine Intelligence,
12(7):629–639.
Rahman, Z., Jobson, D. J., and Woodell, G. A. (1996).
Multi-scale retinex for color image enhancement. In-
ternational Conference on Image Processing.
Turk, M. A. and Pentland, A. P. (1991). Face recognition
using eigenfaces. IEEE Proceedings of Computer Vi-
sion and Pattern Recognition, pages 586–591.
van den Boomgaard, R. (2004). Geometry driven diffusion.
Lecture Notes at University of Amsterdam.
Villegas-Santamaria, M. and Paredes-Palacios, R. (2005).
Comparison of illumination normalization for face
recognition. Third COST 275 Workshop Biometrics
on the Internet, pages 27–30.
Wang, H., Li, S. Z., and Wang, Y. (2004). Face recognition
under varying lighting conditions using self quotient
image. Sixth IEEE International Conference on Auto-
matic Face and Gesture Recognition, pages 819–824.
Weickert, J. (1998). Anisotropic Diffusion in Image Pro-
cessing. Teubner-Verlag, Stuttgart.
Westin, C.-F., Maier, S., Mamata, H., Nabavi, A., Jolesz, F.,
and Kikinis, R. (2002). Processing and visualization
for diffusion tensor mri. In Medical Image Analysis,
Volume 6, Number 2, pages 93–108.
Witkin, A. P. (1983). Scale space filtering. Proceedings In-
ternational Joint Conference on Artificial Intelligence,
pages 1019–1023.
DIFFUSION FILTERING FOR ILLUMINATION INVARIANT FACE RECOGNITION - Illumination Approximation
with Diffusion Filters within Retinex Context
247