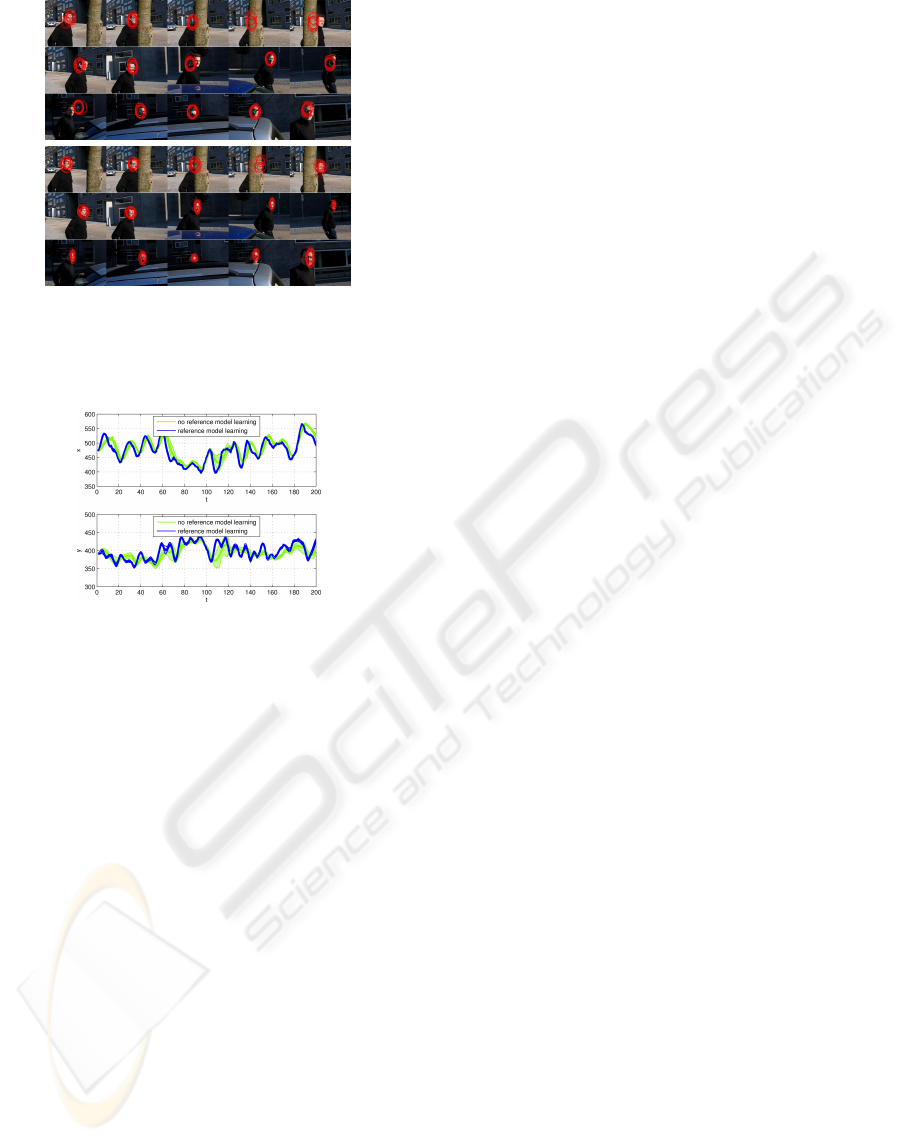
(a)
(b)
Figure 4: Outdoor tracking. Frames: 7, 11, 12, 13, 14, 20,
42, 63, 80, 107, 136, 146, 158, 165, 192 (the frame number
is assigned from left to right and top to bottom). (a) Track-
ing without appearance model adaptation. (b) Tracking with
on-line appearance model learning.
Figure 5: Averaged trajectory with standard deviation in x
and y of outdoor sequence (over 10 runs).
the standard deviation over 10 different tracking runs
performed for the outdoor scene. In the case of ap-
pearance model learning, we can observe in the video
sequences that the tracking of the face gives highly
similar trajectories. The standard deviation is small
and approximately constant over time. However, if
no learning of the reference model is performed the
standard deviation is large in certain time segments.
This leads to the conclusion that model adaptation re-
sults in a more robust tracking.
4 CONCLUSIONS
We propose a robust visual tracking algorithm for
multiple objects (faces of people) in a meeting sce-
nario based on low-level features as skin-color, tar-
get motion, and target size. Based on these features
automatic initialization and termination of objects is
performed. For tracking a sampling importance re-
sampling particle filter has been used to propagate
sample distributions over time. Furthermore, we use
on-line learning of the target models to handle the ap-
pearance variability of the objects. Numerous exper-
iments on meeting data show the capabilities of the
tracking approach. The participants were successfully
tracked over long image sequences. Partial occlusions
are handled by the algorithm. Additionally, we em-
pirically show that the adaptation of the appearance
model during tracking of an outdoor scene results in
a more robust tracking.
REFERENCES
Arulampalam, S., Maskell, S., Gordon, N., and Clapp, T.
(2002). A tutorial on particle filters for on-line on-
linear/non-gaussian Bayesian tracking. IEEE Trans-
actions on Signal Processing, 50(2):174–188.
Cai, Y., de Freitas, N., and Little, J. (2006). Robust visual
tracking for multiple targets. In European Conference
on Computer Vision (ECCV).
Cover, T. and Thomas, J. (1991). Elements of information
theory. John Wiley & Sons.
Dempster, A., Laird, N., and Rubin, D. (1977). Maxi-
mum likelihood estimation from incomplete data via
the EM algorithm. Journal of the Royal Statistic Soci-
ety, 30(B):1–38.
Dockstader, S. and Tekalp, A. (2000). Tracking multiple ob-
jects in the presence of articulated and occluded mo-
tion. In Workshop on Human Motion, pages 88–98.
Doucet, A. (1998). On sequential Monte Carlo sampling
methods for Bayesian filtering. Technical Report
CUED/F-INFENG/TR. 310, Cambridge University,
Dept. of Eng.
Duda, R., Hart, P., and Stork, D. (2000). Pattern classifica-
tion. John Wiley & Sons.
Haykin, S. (2001). Kalman filtering and neural networks.
John Wiley & Sons.
Hue, C., Le Cadre, J.-P., and P´erez, P. (2002). Tracking mul-
tiple objects with particle filtering. IEEE Transactions
on Aerospace and Electronic Systems, 38(3):791–812.
Isard, M. and Blake, A. (1998). Condensation - conditional
density propagation for visual tracking. International
Journal of Computer Vision, 29(1):5–28.
Jepson, A., D.J., F., and El-Maraghi, T. (2003). Robust
online appearance models for visual tracking. IEEE
Trans. on Pattern Analysis and Machine Intelligence,
25(10):1296–1311.
Lim, J., Ross, D., Lin, R.-S., and Yang, M.-H. (2005). In-
cremental learning for visual tracking. In Advances in
Neural Information Processing Systems 17.
Lin, J. (1991). Divergence measures based on the Shannon
entropy. IEEE Trans. on Inf. Theory, 37(1):145–151.
Nummiaro, K., Koller-Meier, E., and Van Gool, L. (2003).
An adaptive color-based particle filter. Image Vision
Computing, 21(1):99–110.
Okuma, K., Taleghani, A., de Freitas, N., Little, J., and
Lowe, D. (2004). A boosted particle filter: Multitar-
get detection and tracking. In European Conference
on Computer Vision (ECCV).
P´erez, P., Hue, C., Vermaak, J., and Gangnet, M. (2002).
Color-based probabilistic tracking. In European Con-
ference on Computer Vision (ECCV).
Sonka, M., Hlavac, V., and Boyle, R. (1999). Image
processing, analysis, and machine vision. Interna-
tional Thomson Publishing Inc.
Vermaak, J., Doucet, A., and P´erez, P. (2003). Maintaining
multi-modality through mixture tracking. In Interna-
tional Conference on Computer Vision (ICCV), pages
1110–1116.
VISAPP 2008 - International Conference on Computer Vision Theory and Applications
468