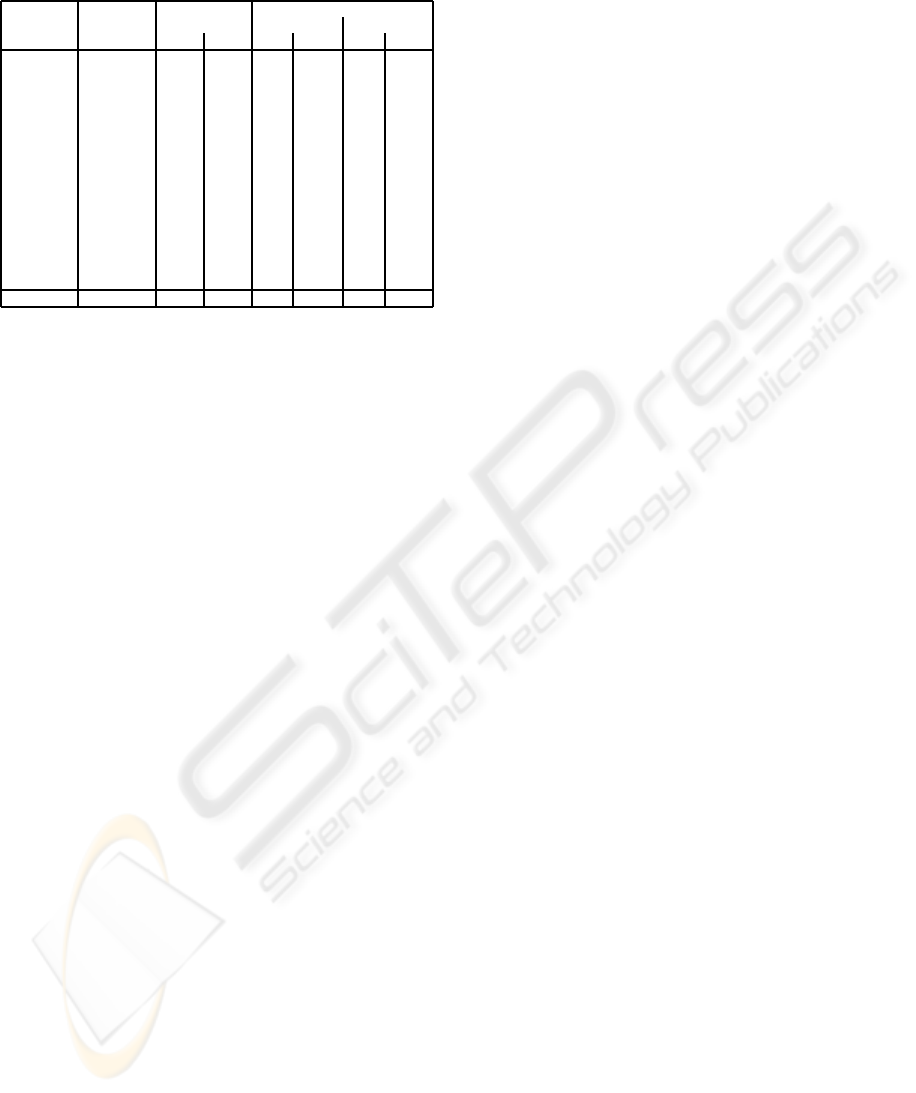
Table 1: Performance evaluations.
Collecting Selection Clustering
images Valid Images False negative False positive
Downloads N. % N. % N. %
Bowl 1596 140 9% 8 6% 25 18%
Candle 2032 139 7% 11 8% 32 23%
Chair 4862 602 12% 25 4% 40 7%
Desk 3185 344 11% 40 12% 55 16%
Door 3754 288 8% 00 3% 100 35%
Fork 2264 168 7% 12 7% 20 12%
Glass 4828 396 8% 250 63% 8 2%
Hammer 3561 422 12% 60 14% 55 13%
Knife 4506 551 12% 32 6% 66 12%
Lamp 4773 479 10% 30 6% 16 3%
Pen 3495 460 13% 28 6% 24 5%
Spoon 3699 150 4% 14 9% 22 15%
Sunglasses 962 190 20% 8 4% 25 13%
Torch 1585 112 7% 9 8% 36 32%
Watch 4050 510 13% 44 9% 45 9%
Total (%) 50324 5116 10% 590 12% 607 12%
is related to images of word chair using different hy-
ponyms from WordNet. In this case the list of first
five keywords used for word “chair” is: “armchair”,
“barber”, “longue”, “chaise”, “daybed”. The filtering
phase individuates 63 (of 602) images that have a de-
tectable shape, and 2 clusters are validated as source
of shape models: they have a sufficient number of im-
ages (≥ 10), and a mean error under a given thresh-
old. The effectiveness of the approach could be high-
lighted by some specific examples: in bottom part of
figure 2 we see two images of chairs grouped in the
same cluster that are impossible to correlate if we con-
sider texture, color, or other image features different
from shape; the upper part reports images of screw-
driver that demonstrate the independence from scale,
orientation, and mirroring.
Results of an extensive experiment are reported in
table 1, using 16 different words of common objects.
The table shows the number of images downloaded
for each word (column 2), the number of valid images
to create prototypes and percentage with respect to
downloaded images (column 3 and 4), and the perfor-
mance of clustering (last 4 columns): absolute num-
ber and percentage with respect to the number of valid
images of images erroneously excluded from relevant
clusters, and absolute number and percentage of im-
age of object wrongly included in some clusters. In
general results could be considered positive, even if
some words are intrinsically difficult to manage for
our aims: glass images report very different typolo-
gies of objects and in this case an interaction with user
could be necessary.
Future works will deal with integration in the
frameworkof other visual features (texture and color),
in order to have better results. Moreover, it is interest-
ing to explore the possibility to defines categories (or
typologies) of the same object using keywords, and to
find or define some simple relation among them based
on visual features.
ACKNOWLEDGEMENTS
The work of this paper was partially supported by
project POR Sicilia 2000-2006,1999/IT.16.1.PO.011/
3.13/7.2.4/342.
REFERENCES
Del Bimbo, A. and Pala, P. (1997). Visual image retrieval by
elastic matching of user sketches. IEEE Trans. on Pat-
tern Analysis and Machine Intelligence, vol. 19 (no.
2), pp. 121-132.
Fergus, R., Fei-Fei, L., Perona, P., and Zisserman, A.
(2005). Learning object categories from google’s im-
age search. ICCV, pages 1816–1823.
Jia, L. and Wang, J. Z. (2003). Automatic linguistic in-
dexing of pictures by a statistical modeling approach.
IEEE transaction on pattern analysis and machine in-
telligence, vol 25, no. 9.
Lee, D. J. Antani, S. and Long, L. R. (2003). Similarity
measurement using polygon curve representation and
fourier descriptors for shape-based vertebral image re-
trieval. Proceedings of IS&T/SPIE Medical Imaging
2003: Image Processing, vol. SPIE 5032, pp. 1283-
1291.
Oliver, A., Munoz, X., Batlle, J., Pacheco, L., and Freix-
enet, J. (2006). Improving clustering algorithms for
image segmentation using contour and region infor-
mation. Automation, Quality and Testing, Robotics,
2006 IEEE Intl. Conf., pages 315–320.
POW. Download page:. http://www.pa.icar.cnr.it/ in-
fantino/demo/.
Rivest, R. L. (1992). The md5 message digest algorithm.
In: Internet, RFC 1321.
Tieu, K. and Viola, P. (2004). Boosting image retrieval.
Intl. Journal of Computer Vision, pages vol. 56(1/2),
pp. 1736.
Wikipedia. Home page. http://wikipedia.org.
Wordnet. Home page. http://wordnet.princeton.edu/.
Zhang, D. and Lu, G. (2002). Shape-based image retrieval
using generic fourier descriptor. Signal Processing:
Image Communication, vol.17, no. 10, pp. 825-848.
Zinger, S., Millet, C., Mathieu, B., Grefenstette, G., Hede,
P., and Moellic, P. A. (2006). Clustering and seman-
tically filtering web images to create a large-scale im-
age ontology. Proc. Of IS-T/SPIE 18th Symposium
Electronic Imaging.
AUTOMATED OBJECT SHAPE MODELLING BY CLUSTERING OF WEB IMAGES
255