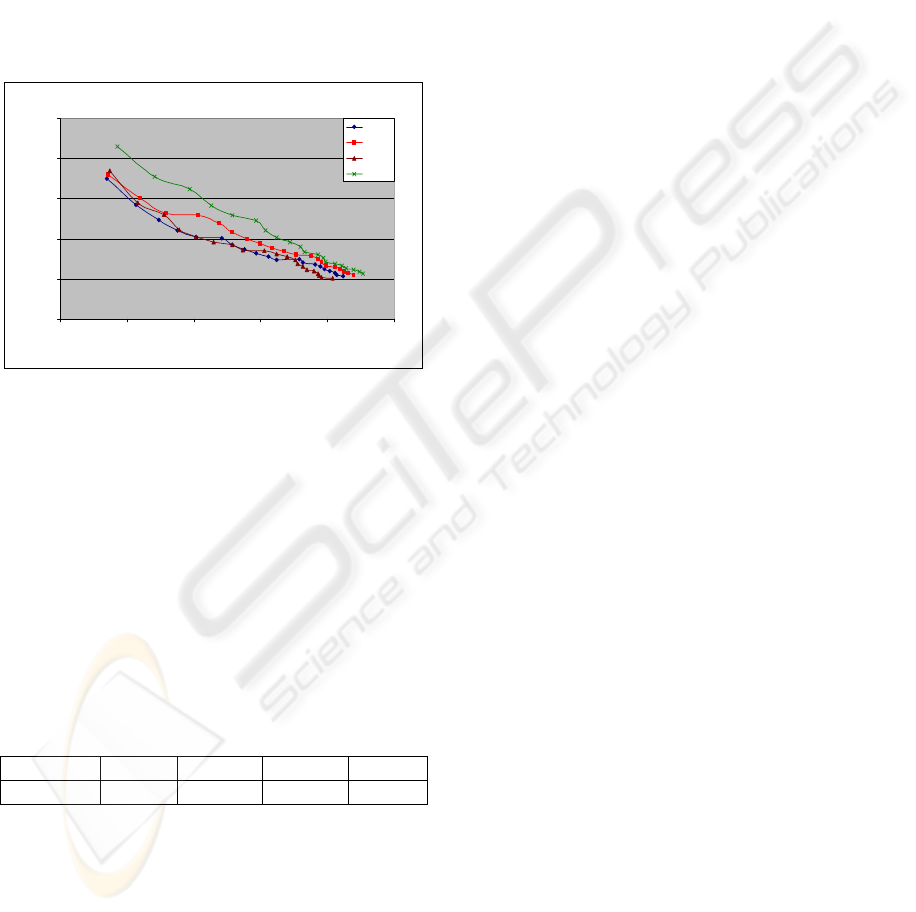
Figure 3 shows the precision versus recall plot
for comparing the performance of methods. From
the plot, it is noted that our method (SNH)
outperformed the other three methods with higher
precision for the same recall rate. For top rank
match, SNH obtains the highest precision rate of
0.86 followed by EOAC, EPNH and EDH with 0.74,
0.72 and 0.70 respectively.
For ANMRR evaluation, we exploit the top 5
retrieved images for a query images and calculate
the NMRR. Then, we calculate the ANMRR for all
50 query images. The result obtained is shown in
table 1.
Precision v s. Recall
0%
20%
40%
60%
80%
100%
0% 20% 40% 60% 80% 100%
Recall (% )
Precision (%)
EDH
EPNH
EOAC
SNH
Figure 3: Performance comparison between SNH (our
method), EDH, EPNH, and EOAC.
The experimental results have indicated that our
method is capable of obtaining an acceptable
performance in terms of ANMRR. SNH has the
lowest ANMRR followed by EPNH. Lower value of
ANMRR shows that our method has better result
than the other three methods. The results also show
that, for EOAC and EDH, their performance is at
approximately the same level. These results have
indicated that our method is efficient and capable of
producing a good performance.
Table 1: Comparison of ANMRR for SNH, EPNH, EOAC
and EDH.
SNH EPNH EOAC EDH
ANMRR 0.4500 0.4935 0.5630 0.5625
4 CONCLUSIONS
Our study has shown that integrating spatial
neighborhood information into a histogram can
increase the retrieval system performance. The
separate use of the edge and LBP information
produces good retrieval result. In our work, we have
shown that combining both properties can further
improve a system’s performance. For images of
man-made structure such as buildings, SNH
produces better results when compared to other
similar methods.
Although our method is simple and
straightforward, the experimental results have shown
that it is capable of improving the retrieval precision.
For future work, further tests with large-scale image
database are expected. We also plan to integrate
other features to the histogram in order to improve
its efficiency.
REFERENCES
Chalechale, A & Mertins, A 2002, ‘Semantic Evaluation
and Efficiency Comparison of the Edge Pixel
Neighboring Histogram in Image Retrieval’,
Proceedings of the First Workshop on the Internet,
Telecommunication and Signal Processing, vol. 1, pp.
179–184.
Iqbal, Q & Aggarwal, J.K. 1999, ‘Applying perceptual
grouping to content-based image retrieval: Building
Images’, Proceedings of the IEEE International
Conference of Computer Vision and Pattern
Recognition, pp. 42-48.
Li, Y & Shapiro, LG 2002, ‘Consistent line clusters for
building recognition in CBIR’, Proceedings of the 16
th
International Conference of Pattern Recognition,
vol.3, pp. 952-956.
Manjunath, BS, Salembier, P & Sikora, T 2002,
‘Introduction to MPEG-7’, John Wiley & Sons Ltd.
Mahmoudi, F, Shahbenzadeh, J, Eftekhari-Moghadam, A-
M & Soltanian-Zadeh, H 2003, ‘A new non-
segmentation shape-based image indexing method’,
Proceedings of the IEEE International Conference on
Acoustics, Speech, and Signal Processing, vol.3, pp.
III-17-20
Ojala, T, Pretikainen, M & Harwood, D 1999, ‘A
Comparative Study of Texture Measures with
Classification Based on Feature Distribution’, Pattern
Recognition, vol. 29(1), pp 51-59.
Vailaya, A, Jain, A & Zhang, HJ, 1998, ‘On Image
Classification: City vs. Landscape’, Proceedings of the
IEEE Workshop on Content-Based Access of Image
and Video Libraries, pp. 3 -8.
Veltkamp, RC 2001, ‘Shape Matching: Similarity
Measures and Algorithms’, Proceedings of Shape
Modelling International, pp 188-197.
Zhang, W & Kosecka, J 2005, ‘Localization Based on
Building Recognition’, Proceedings of the 2005 IEEE
Computer Society Conference on Computer Vision
and Pattern Recognition, vol. 3, p. 21.
SPATIAL NEIGHBORING HISTOGRAM FOR SHAPE-BASED IMAGE RETRIEVAL
259