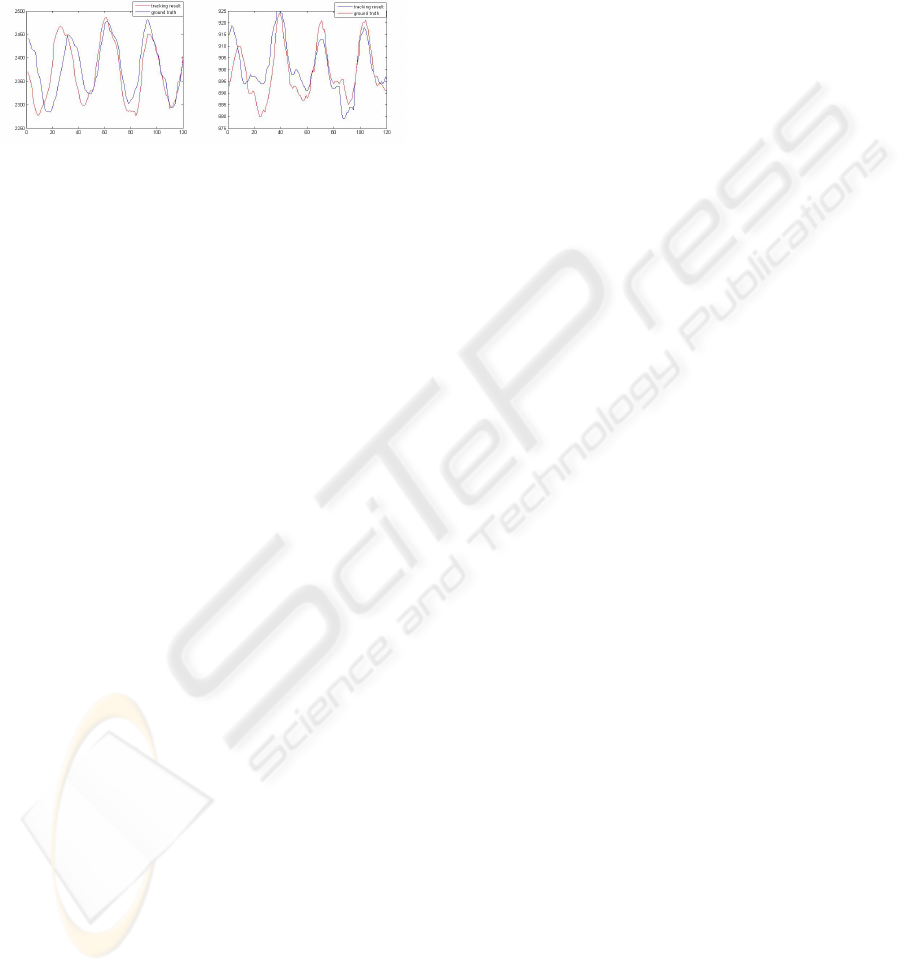
The comparisons between tracking results from
the first image series shown in figure 3 and ground
truth from 5DT Cyber Glove are shown in Figure 6.
The NBP in feasible configuration space based
articulated 3D tracker can achieve 2.5 frames/second
in average for the shown 320 × 240 image series on
a Pentium (R) D 3.4G desktop.
Figure 6: The comparison between our tracking results of
figure 3 and the ground truth. The abscissa represents
training data (frames), the ordinate represents phalange’s
angle. (left) distal phalanx of middle finger, (right) distal
phalanx of little finger.
5 CONCLUSIONS
Due to the high dimensionality of human hand
incurring complexity of hand tracking, graphical
models are widely used to decompose multivariate,
joint distributions into a set of local interactions. In
addition to the traditional physiological constraints
and temporal information, we also introduce
occlusion constraints of hand motion. The advantage
of this framework is that self occlusion could be
partially solved. Consequently, the hand tracking is
transformed into an inference of graphical model.
We utilize embedded sequential mode
propagation underlying NBP in a restrict hand
motion space obtained by CAMSHIFT to perform
hand tracking. It accelerates tracking procedure
remarkably. The experiment results show the
capability of the entire framework.
REFERENCES
Wu, Y., Lin, J., Huang, T. S., December 2005. Analyzing
and Capturing Articulated Hand Motion in Image
Sequences. In Vol. 27, No. 12, IEEE Transactions on
Pattern Analysis and Machine Intelligence.
Stenger, B., Wu, Y., Mendonca, P. R. S., 2001. Model-
based 3D tracking of an articulated hand. In pp. 310-
315, Vol. 2. Proc. IEEE Conf. Computer Vision and
Pattern Recognition.
Wu, Y., Lin, J. Y., Huang, T. S., 2001. Capturing natural
hand articulation. In pp. 426-432, Vol. 2. Proc. IEEE
Int. Conf. Computer Vision.
Isard, M., Blake, A, 1998. CONDENSATION —
conditional density propagation for visual tracking. In
pp. 5-28, Vol. 29. International Journal of Computer
Vision.
Sudderth, E. B., Mandel, M. I., Freeman, W. T., Willsky,
A. S., May 2004. Visual Hand Tracking Using
Nonparametric Belief Propagation. In MIT Laboratory
For Information & Decision Systems Technical
Report.
Lin, J. Y., Wu, Y., Huang, T. S., December 2002.
Capturing Human Hand Motion in Image Sequences.
In pp. 99-104. Proc. IEEE Workshop Motion and
Video Computing.
Chao, E., An, K., Cooney, W., Linscheid, R., 1989.
Biomechanics of the Hand: A Basic Research Study.
In Mayo Foundation, Minn.: World Scientific.
Tony, X. H., Ning, H. Z., Huang, T. S., 2006. Efficient
Nonparametric Belief Propagation with Application to
Articulated Body Tracking. In pp. 214-221, Vol. 1,
Proc IEEE Conf. Computer Vision and Pattern
Recognition.
Isard, M., 2003. PAMPAS: Real-Valued Graphical
Models for Computer Vision. In pp. 18-20, Vol. 1,
Proc IEEE Conf. Computer Vision and Pattern
Recognition.
Sigal, S., Bhatia, S., Roth, S., Black, M. J., Isard, M.,
2004. Tracking Loose-limbed People. In pp. 421-428,
Vol. 1, Proc IEEE Conf. Computer Vision and Pattern
Recognition.
Frey, B. J., Bhatia, S., MacKay, D. J. C., 1998. A
revolution: Belief propagation in graphs with cycles.
In pp. 479-485, Vol. 1, Neural Information Processing
Systems 10, MIT Press.
Wu, Y., Huang, T. S., May 2001. Hand modeling, analysis,
and recognition. In pp. 51-60, IEEE Signal Proc.
Mag..
Stenger, B. J., Mendonca, P. R. S., Cipolla, R., 2001.
Model–based 3D tracking of an articulated hand. In pp.
310-315
, Vol. 2, Proc IEEE Conf. Computer Vision
and Pattern Recognition.
3D ARTICULATED HAND TRACKING BY NONPARAMETRIC BELIEF PROPAGATION ON FEASIBLE
CONFIGURATION SPACE
513