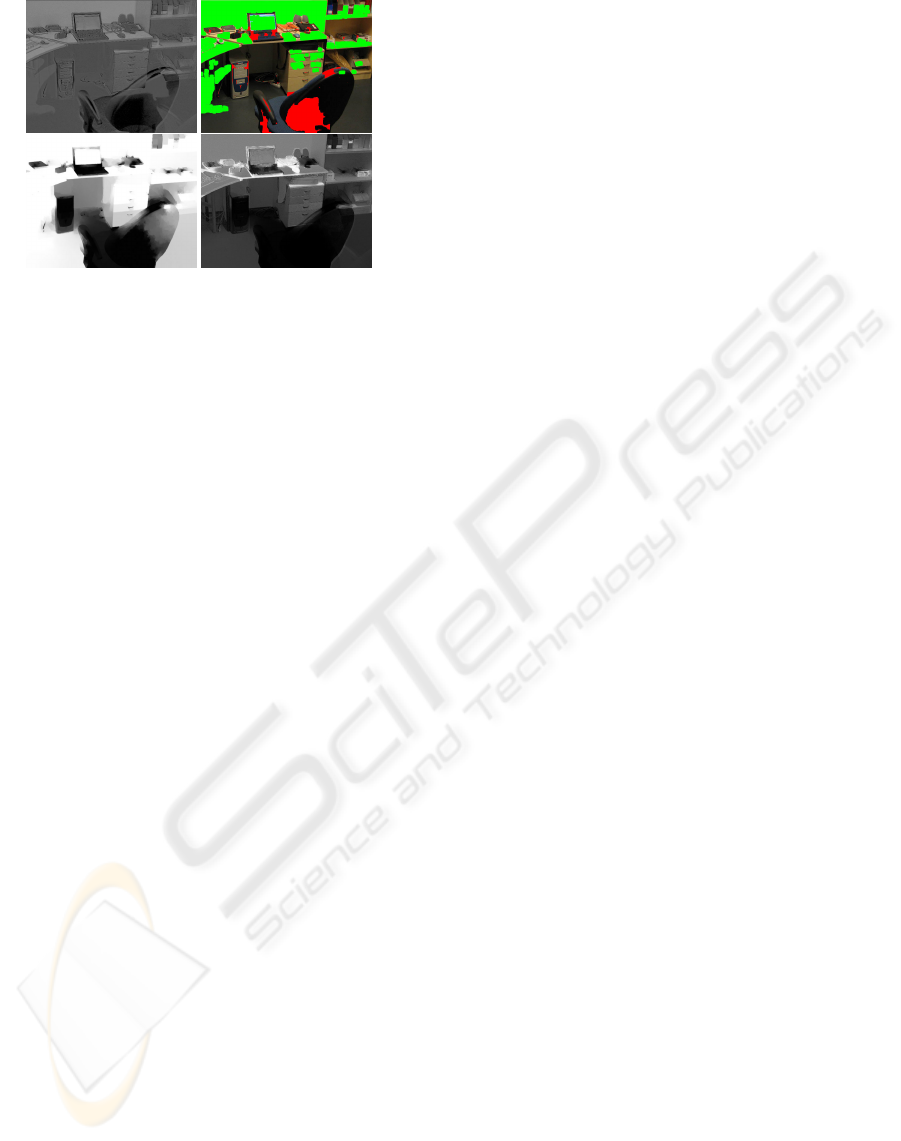
Figure 1: The main steps of the propagation process:
weights obtained from density estimation; low and high
weights segmented; propagated weights; final weights.
putation time, in case of big images, we perform the
propagation step on downsampled versions of the ex-
posures, and resize the results back to their original
dimensions.
4 RESULTS AND CONCLUSION
We compared our approach to the one described in
(Khan et al., 2006). Reinhard’s photographic opera-
tor was used to tonemap the generated HDR images
(Reinhard et al., 2002). Our approach does not require
any setting to be adjusted by the user. In all the exper-
iments shown we used only one global bandwidth ma-
trix that is reused at every iteration: the more general
approach described resulted in a significant increase
of computation time with little benefits. For Khan’s
method, we used a default identity bandwidth matrix,
and 3 × 3 × R neighborhoods. We included in Figure
2 some of the exposures used for generating the final
HDR images. Figure 3 shows the results of the exper-
iments. In the first scene, ghosting is localized and oc-
curs in regions that have high dynamic range; artifacts
are completely removed only with our algorithm. In
the second scene, the situation is similar but less expo-
sures were available. Density estimation alone could
not distinguish properly the background, while the
weight propagation helped to improve the results. Fi-
nally we considered a handheld set of exposures in-
tentionally left unaligned, and where chaotic move-
ment is present; this sequence does not hold the as-
sumption that the background is prevalently captured
and suffers from critical occlusion and parallax prob-
lems. In spite of this, our method proved a remarkable
robustness against feature misalignments. In all the
cases that have been considered, our approach showed
a significant improvement in reducing ghosting arti-
facts, and when the previously mentioned assumption
holds, ghosts can be completely eliminated even with
a single iteration.
REFERENCES
Bogoni, L. (2000). Extending dynamic range of
monochrome and color images through fusion. In In-
ternational Conference on Pattern Recognition, 2000,
vol. 3, pp. 7-12.
Debevec, P. and Malik, J. (1997). Recovering high dynamic
range radiance maps from photograph. In SIGGRAPH
97, August 1997.
Grosch, T. (2006). Fast and robust high dynamic range
image generation with camera and object movement.
In Vision, Modeling and Visualization (VMV), RWTH
Aachen, 22.11 - 24.11.2006.
Grossberg, M. D. and Nayar, S. K. (2003). Determining the
camera response from images: What is knowable? In
IEEE Transactions on Pattern Analysis and Machine
Intelligence, Vol.25, No.11, pp.1455-1467, Nov, 2003.
Jacobs, K., Ward, G., and Loscos, C. (2005). Automatic
hdri generation of dynamic environments. In ACM
SIGGRAPH 2005 Sketches.
Khan, E. A., Akyuz, A. O., and Reinhard, E. (2006). Ghost
removal in high dynamic range images. In IEEE In-
ternational Conference on Image Processing, Atlanta,
USA, August 2006.
Kim, S. J. and Pollefeys, M. (2004). Radiometric alignment
of image sequences. In IEEE Computer Society Con-
ference on Computer Vision and Pattern Recognition
(CVPR’04) - Volume 1, pp. 645-651.
Lischinski, D., Farbman, Z., Uyttendaele, M., and Szeliski,
R. (2006). Interactive local adjustment of tonal val-
ues. In ACM Transactions on Graphics, ACM SIG-
GRAPH 2006 Papers SIGGRAPH ’06, Volume 25 Is-
sue 3. ACM Press.
Raykar, V. C. and Duraiswami, R. (2006). Very fast opti-
mal bandwidth selection for univariate kernel density
estimation. In CS-TR-4774, Department of Computer
Science, University of Maryland, Collegepark.
Reinhard, E., Stark, M., Shirley, P., and Ferwerda, J. (2002).
Photographic tone reproduction for digital images. In
ACM Transactions on Graphics, 21(3), pp 267-276,
Proceedings of SIGGRAPH 2002.
Reinhard, E., Ward, G., Pattanaik, S., and Debevec, P.
(2005). High Dynamic Range Imaging: Acquisition,
Display and Image-Based-Lighting. Morgan Kauf-
mann.
Scott, D. W. (1992). Multivariate Density Estimation: The-
ory, Practice, and Visualization. John Wiley.
Silverman, B. W. (1986). Density Estimation for Statistics
and Data Analysis. Chapman and Hall.
Zhang, X., King, M. L., and Hyndman, R. J. (2004). Band-
width selection for multivariate kernel density estima-
tion using mcmc. In Monash Econometrics and Busi-
ness Statistics Working Papers 9/04.
VISAPP 2008 - International Conference on Computer Vision Theory and Applications
40