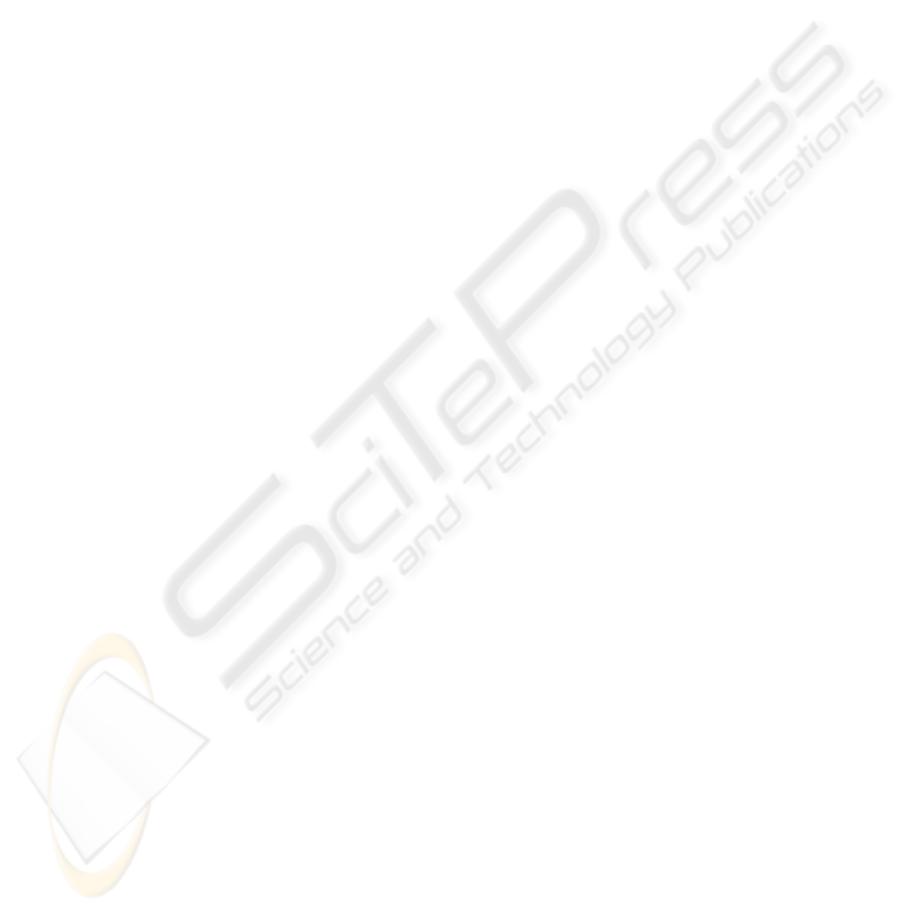
for motion texture detection as well. Moreover, the
reference classes can be learned from a single instan-
taneous motion map allowing, eventually, to define an
adaptive scheme for recognition and classification.
Future prospects are based on considering other
dissimilarity measures between statistical models,
combining the classification and detection approach
with existing motion or dynamic texture segmentation
methods and considering the introduction of contex-
tual information through discriminative models, pos-
sibly in the form of Conditional Markov Random
Fields (CMRF).
REFERENCES
Besag, J. (1974). Spatial interaction and the statistical anal-
ysis of lattice systems. Journal of the Royal Statistical
Society. Series B, 36:192–236.
Bouthemy, P., Hardouin, C., Piriou, G., and Yao, J. (2006).
Mixed-state auto-models and motion texture model-
ing. Journal of Mathematical Imaging and Vision,
25:387–402.
Cernuschi-Frias, B. (2007). Mixed-states markov random
fields with symbolic labels and multidimensional real
values. Rapport de Recherche INRIA.
Crivelli, T., Cernuschi, B., Bouthemy, P., and Yao, J. (2006).
Segmentation of motion textures using mixed-state
markov random fields. In Proceedings of SPIE, vol-
ume 6315, 63150J.
Doretto, G., Chiuso, A., Wu, Y., and Soatto, S. (2003).
Dynamic textures. Intl. Journal of Comp. Vision,
51(2):91–109.
Fablet, R. and Bouthemy, P. (2003). Motion recognition
using non-parametric image motion models estimated
from temporal and multiscale co-ocurrence statistics.
IEEE Trans. on Pattern Analysis and Machine Intelli-
gence, 25(12):1619–1624.
Fazekas, S. and Chetverikov, D. (2005). Normal versus
complete flow in dynamic texture recognition: a com-
parative study. In Texture 2005: 4th international
workshop on texture analysis and synthesis. ICCV’05,
Beijing, pages 37–42.
Geman, S. and Geman, D. (1984). Stochastic relaxation,
gibbs distributions, and the bayesian restoration of im-
ages. IEEE Transactions on Pattern Analysis and Ma-
chine Intelligence, 6:721–741.
Lu, Z., Xie, W., Pei, J., and Huang, J. (2005). Dynamic
texture recognition by spatio-temporal multiresolution
histograms. In IEEE Workshop on Motion and Video
Computing (WACV/MOTION), pages 241–246.
Peteri, R. and Chetverikov, D. (2005). Dynamic texture
recognition using normal flow and texture regularity.
In Proc. of IbPRIA, Estoril, pages 223–230.
Peteri, R., Huiskes, M., and Fazekas, S. (2005). Dyn-
tex: A comprehensive database of dynamic textures.
http://www.cwi.nl/projects/dyntex/index.html.
Saisan, P., Doretto, G., Wu, Y., and Soatto, S. (2001).
Dynamic texture recognition. In Proc. of the IEEE
Conf. on Computer Vision and Pattern Recognition.
CVPR’01, Hawaii, pages 58–63.
Salzenstein, F. and Pieczynski, W. (1997). Parameter es-
timation in hidden fuzzy markov random fields and
image segmentation. Graph. Models Image Process.,
59(4):205–220.
Vidal, R. and Ravichandran, A. (2005). Optical flow esti-
mation and segmentation of multiple moving dynamic
textures. In Proc. of CVPR’05, SanDiego, volume 2,
pages 516–521.
Yuan, L., Wen, F., Liu, C., and Shum, H. (2004). Synthesiz-
ing dynamic textures with closed-loop linear dynamic
systems. In Proc. of the 8th European Conf. on Com-
puter Vision, ECCV’04, Prague, volume LNCS 3022,
pages 603–616.
RECOGNITION OF DYNAMIC VIDEO CONTENTS BASED ON MOTION TEXTURE STATISTICAL MODELS
289