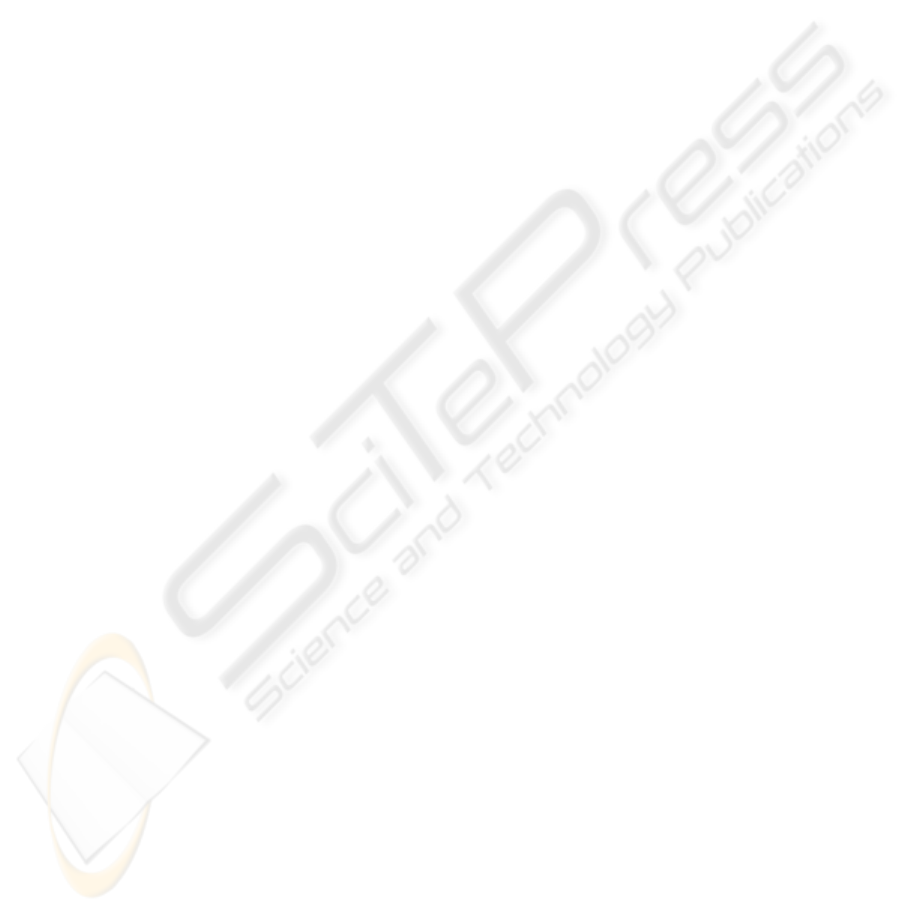
6 CONCLUSIONS
The proposed model combines information from mo-
tion streaks and image flow, forming the signals mag-
nitude of image flow, intensity of motion streaks, and
coherence of motion directions. These three signals
are combined for the final attention signal. An ROC
analysis for scenarios of video surveillance is con-
ducted and shows that three attentive signals and the
final signal are appropriate for the division between
attentive motion and background noise. Compared
with the method of (Tian and Hampapur, 2005) our
model has only one critical threshold and shows bet-
ter results for two analyzed scenes. Main challenges
solved by our model are the robust processing and
analysis of noisy background and locally incoherent
motions of walking persons.
Within our model motion streaks are used, which
provide information about orientation and speed for
object and self-motion. In addition to the approach
of (Majchrzak et al., 2000) our model provides a
dense field of orientations and speeds. Therefore, mo-
tion streaks could serve as a robustprior or bias for the
estimation of image flow and also for the estimation
of ego-motion. Future work will pursue incorpora-
tions of motion streaks into those estimation tasks.
ACKNOWLEDGEMENTS
This work is supported by the Graduate School
Mathematical Analysis of Evolution, Information and
Complexity.
REFERENCES
Barron, J., Fleet, D., and Beauchemin, S. (1994). Perfor-
mance of optical flow techniques. IJCV, pages 43–77.
Brown, L., Senior, A., Tian, Y.-L., Connell, J., Hampapur,
A., Shu, C.-F., Merkl, H., and Lu, M. (2005). Perfor-
mance evaluation of surveillance systems under vary-
ing conditions. IEEE Int’l Workshop on Performance
Evaluation of Tracking and Surveillance.
Daugman, J. (1988). Complete discrete 2-D Gabor trans-
forms by neural networks for image analysis and com-
pression. Trans. Acoustics, Speech, and Signal Proc.,
26(7):1169–1179.
Deneve, S., Latham, P., and Pouget, A. (1999). Reading
population codes: a neural implementation of ideal
observers. Nature Neuroscience, 2:740–745.
F¨orstner, W. (1986). A feature based correspondence algo-
rithm for image matching. ISP Comm. III, Rovaniemi
1986, International Archives of Photogrammetry,
pages 26–3/3.
Hubel, D. and Wiesel, T. (1968). Receptive fields and func-
tional architecture of monkey striate cortex. J. Phys-
iol., (195):215–243.
Lucas, B. and Kanade, T. (1981). An iterative image regis-
tration technique with an application to stereo vision.
Proc. DARPA Image Understanding Workshop, pages
121–130.
Majchrzak, D., Sarkar, S., Sheppard, B., and Murphy, R.
(2000). Motion detection from temporally integrated
images. In Proc. IEEE 15th ICPR, pages 836–839.
Marr, D. and Ullman, S. (1981). Direction selectivity and
its use in early visual processing. Proc. Royal Soc. of
London, B, 211:151–180.
Neumann, H. and Sepp, W. (1999). Recurrent V1-V2 in-
teraction in early visual boundary processing. Biol.
Cybernetics, 81:425–444.
Ren, Y., Chua, C.-S., and Ho, Y.-K. (2003). Motion detec-
tion with nonstationary background. Machine Vision
and Applications, 13:332–343.
Rothenstein, A. and Tsotsos, J. (2007). Attention links sens-
ing to recognition. Image and Vision Computing. (in
press).
Tian, Y.-L. and Hampapur, A. (2005). Robust salient mo-
tion detection with complex background for real-time
video surveillance. Proc. IEEE Workshop on Motion
and Video Computing, pages 30–35.
Tsotsos, J., Liu, Y., Martinze-Trujiloo, J., Pomplun, M.,
Simine, E., and Zhou, K. (2005). Attending to visual
motion. Computer Vision and Image Understanding,
100:3–40.
Weidenbacher, U., Bayerl, P., Neumann, H., and Flemming,
R. (2006). Sketching shiny surfaces: 3D shape extrac-
tion and depicting of specular surfaces. ACM Trans.
on Applied Perception, 3:262–285.
Wixson, L. and Hansen, M. (1999). Detecting salient mo-
tion by accumulating directionally-consistent flow. In
Proc. of the Seventh IEEE ICCV, pages 797–804.
Zhang, W., Fang, X., Yang, X., and Wu, Q. (2007). Spa-
tiotemporal gaussian mixture model to detect moving
objects in dynamic scenes. J. of Electronic Imaging,
16.
Zhou, Q. and Aggarwal, J. (2001). Tracking and classifying
moving objects from video. In IEEE Int. Workshop on
PETS.
VISAPP 2008 - International Conference on Computer Vision Theory and Applications
650