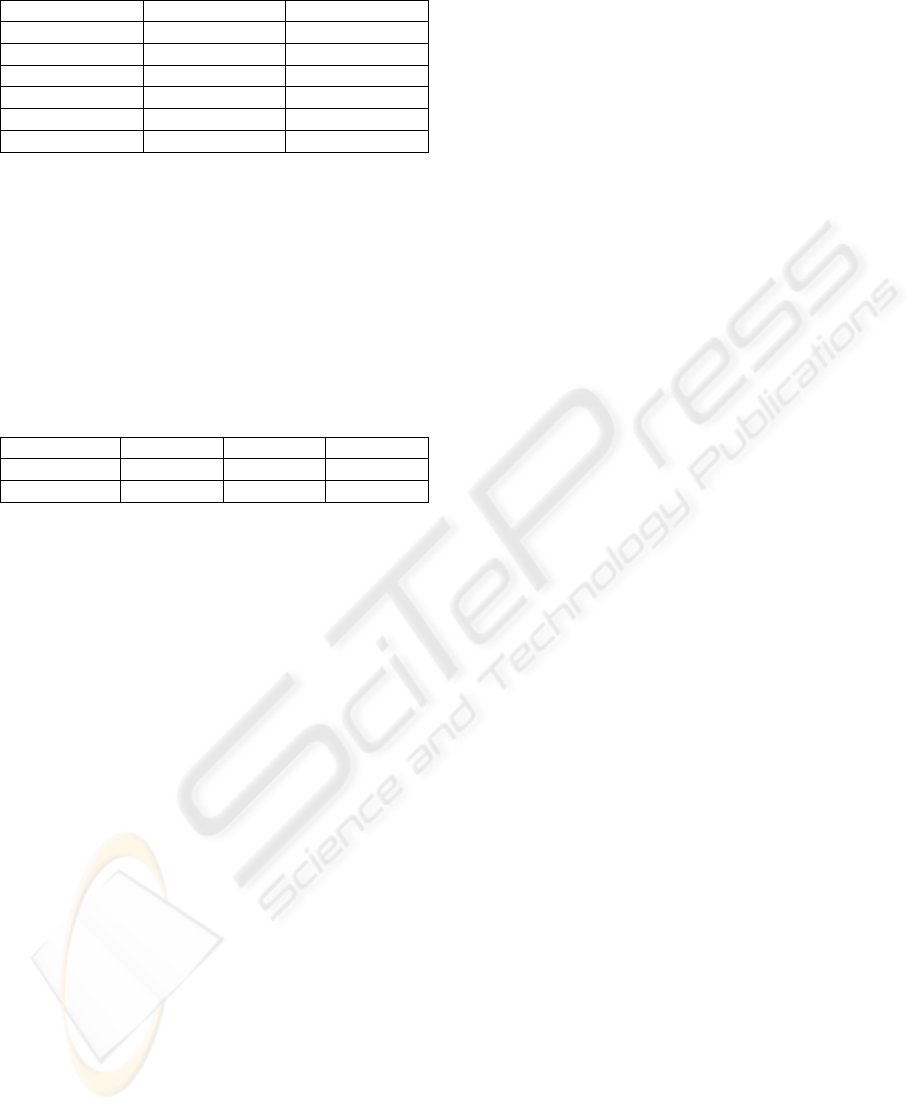
Table 3: Average precision for top 20 and top 50 images.
Iteration times P20 P50
#0 0.4982 0.3878
#1 0.6026 0.4570
#2 0.6446 0.4853
#3 0.6694 0.5013
#4 0.6894 0.5107
#5 0.7028 0.5192
Rao et al. proposed a querying by example
method based on Fuzzy SVM and performed
experiment on 2,000 Corel images (Rao et al., 2006).
Top 20 images were labelled each time. We did
similar QBE experiment on 5,000 Corel images.
Table 4 shows the experiment results between two
methods at three iteration steps (#1, #5, and #10),
Rao et al.’s method is denoted as Fuzzy SVM.
Table 4: Average precision for top 20 images in two
methods at three iteration steps.
P20 #1 #5 #10
Fuzzy SVM About 0.53 About 0.74 About 0.77
MMP 0.5730 0.6681 0.7172
5 CONCLUSIONS
In this paper, we have proposed a new relevance
feedback method based on max-min posterior
pseudo-probabilities framework for learning pattern
classification. We assume that the feature vectors
extracted from the relevant images be of the
distribution of Gaussian mixture model. The
corresponding posterior pseudo-probability function
is used to determine whether the image is relevant to
the user intention. In each feedback process, those
images relevant to the user intention are returned as
the retrieval results and then labeled as true or false
by the user. According to labeled retrieval results,
MMP training criterion is used to update the
parameter set of posterior pseudo-probability
function and subsequent retrieval results. We
conducted concept retrieval and example retrieval
experiments of relevance feedback on Corel
database. After five iterations, P20 has been raised
from 42.20% to 67.20% and from 49.82% to 70.28%
respectively.
ACKNOWLEDGEMENTS
This research was partially supported by the 973
Program of China (2006CB303105) and BIT
Excellent Young Scholars Research Fund
(2006Y1202).
REFERENCES
Chen, S.C., Rubin, S.H., Shyu, M.L., Zhang, C., 2006. A
dynamic user concept pattern learning framework for
content-based image retrieval. IEEE Transactions on
Systems, Man and Cybernetics Part C: Applications
and Reviews, 36(6): 772-783.
Cheng, J., Wang, K., 2007. Active learning for image
retrieval with Co-SVM. Pattern Recognition, 40(1):
330-334.
Deng, Y., Liu, X., Jia, Y., 2007. Learning Semantic
Concepts for Image Retrieval Using the Max-min
Posterior Pseudo-Probabilities Method. In ICME 2007,
IEEE International Conference on Multimedia and
Expo.
Goldmann, L., Thiele, L., Sikora, T., 2006. Online image
retrieval system using long term relevance feedback.
In CIVR 2006, International Conference on Image and
Video Retrieval.
Gosselin, P.H., Cord, M., 2004. RETIN AL: an active
learning strategy for image category retrieval. In ICIP
2004, International Conference on Image Processing.
Liu, X., Jia, Y., Chen, X., Deng, Y., Fu, H., 2006. Max-
Min Posterior Pseudo-Probabilities Estimation of
Posterior Class Probabilities to Maximize Class
Separability. Technical Report BIT-CS-TP-
20060002, Beijing: Beijing Institute of Technology.
(http://www.mcislab.org.cn/technicalreports/MMP.pdf)
Liu, Y., Zhang, D., Lu, G., Ma, W.Y., 2007. A Survey of
Content-Based Image Retrieval with High-level
Semantics. Pattern Recognition, 40(1): 262-282.
Peng, X., King, I., 2006. Imbalanced learning in relevance
feedback with biased minimax probability machine for
image retrieval tasks. In ICONIP 2006, International
Conference on Neural Information Processing.
Rao, Y., Mundur, P., Yesha, Y., 2006. Fuzzy SVM
Ensembles for Relevance Feedback in Image
Retrieval. In CIVR 2006, International Conference on
Image and Video Retrieval.
Tao, D., Tang, X., Li, X., Wu, X., 2006. Asymmetric
bagging and random subspace for support vector
machines-based relevance feedback in image retrieval.
IEEE Transactions on Pattern Analysis and Machine
Intelligence, 28(7): 1088-1099.
Yu, J., Lu, Y., Xu, Y., Sebe, N., Tian, Q., 2007.
Integrating relevance feedback in boosting for content-
based image retrieval. In ICASSP 2007, IEEE
International Conference on Acoustics, Speech, and
Signal Processing.
Zhang, R., Zhang, Z., 2006. BALAS: Empirical bayesian
learning in the relevance feedback for image retrieval.
Image and Vision Computing, 24(3): 211-223.
Zhou, X.S., Huang, T.S., 2003. Relevance feedback in
image retrieval: A comprehensive review. Multimedia
Systems, 8(6): 536-544.
RELEVANCE FEEDBACK WITH MAX-MIN POSTERIOR PSEUDO-PROBABILITY FOR IMAGE RETRIEVAL
289