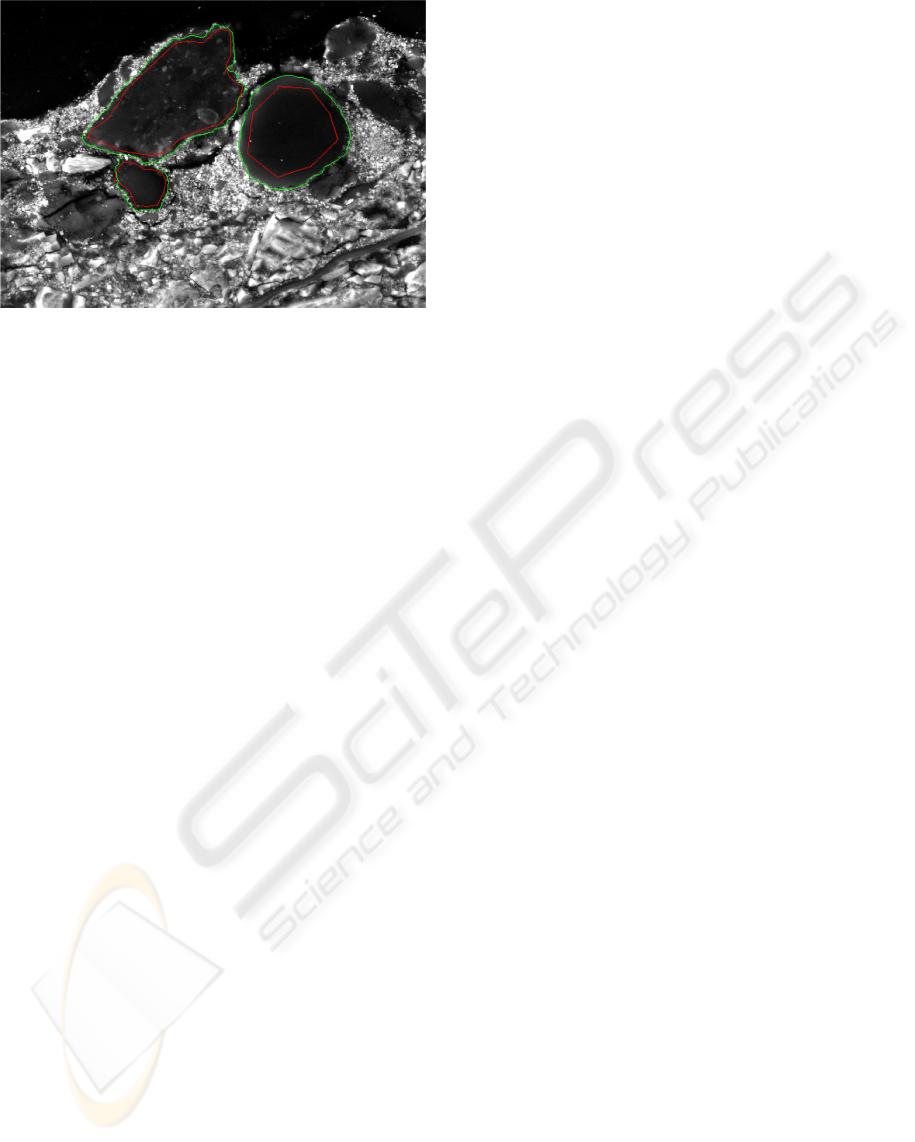
Figure 4: Example of grains segmentation. Green border
represents the resulting segmentation.
they will improve the ability to uncover the authors
of the artwork by revealing the characteristics of their
work with pigments, binders and other components.
One of the first necessary step towards is the segmen-
tation of the grains in the single layers. The best data
source for such task is the SEM data, where individual
grains can be most clearly distinguished. Our algo-
rithmic solution of the grain segmentation is based on
the Parametric Snakes (Xu and Prince, 1998). Fig. 4
shows the example of segmented grains in a SEM im-
age.
5 CONCLUSIONS
The proposed system Nephele can facilitate the work
of material scientists and consequently restorers and
offer them a better access to the archived reports they
use. To our knowledge, no other similar system has
been published up to now. The introduced digital
image processing methods enable acquired data pre-
processing for further analyses as well as improve of
querying above the reports database. The preprocess-
ing of the VS and UV specimen images, used for the
identification of pigment and binder present in the art-
work, consists of image registration, which makes use
of the mutual information approach, and segmenta-
tion technique based on the modified k-means clus-
tering. The included image retrieval system is able
to provide fetching of reports with visually similar
specimen data. The image retrieval is built upon
the VS and UV images of the specimens. They
are represented using the Haralick descriptors of co-
occurrence matrices together with the color descrip-
tors. In the future, single layers will be characterized
by means of the selected sets of features for a bet-
ter definition of the used materials. Recently, the first
step - grains segmentation - was implemented, based
on the parametric snakes model. The presented exam-
ples of achieved results show the applicability of the
system.
ACKNOWLEDGEMENTS
This work was partially supported by the Grant
Agency of Charles University under the project No.
72507, partially by the Ministry of Education of the
Czech Republic under the projects No. 1M0572 (re-
search center) and No. MSM6046144603, and finally
by the Grant Agency of the Czech Republic under the
project No. 203/07/1324.
REFERENCES
Addis, M., Boniface, M., Goodall, S., Grimwood, P., Kim,
S., Lewis, P., Martinez, K., and Stevenson, A. (2003).
Integrated image content and metadata search and re-
trieval across multiple databases. LNCS, 2728:91–
100.
Beckmann, N., Kriegel, H.-P., Schneider, R., and Seeger,
B. (1990). The R
∗
-tree: An efficient and robust ac-
cess method for points and rectangles. In 1990 ACM
SIGMOD International Conference on Management
of Data, volume 19, pages 322–331.
Goodall, S., Lewis, P. H., Martinez, K., Sinclair, P.,
Giorgini, F., Addis, M., Boniface, M., Lahanier, C.,
and Stevenson, J. (2004). Sculpteur: Multimedia re-
trieval for museums. LNCS, 3115:638–646.
Haralick, R. M., Shanmugam, K., and Dinstein, I. (1973).
Textural features for image classification. IEEE
Transactions on Systems, Man and Cybernetics,
SMC-3(6):610–621.
Penney, G. P., Weese, J., Little, J. A., Desmedt, P., Hill,
D. L. G., and Hawkes, D. J. (1998). A comparison of
similarity measures for use in 2D–3D medical image
registration. IEEE Trans. Medical Imaging, 17:586–
595.
Veltkamp, R. C. and Tanase, M. (2000). Content-based im-
age retrieval systems: A survey. Technical Report,
UU-CS-2000-34.
Viola, P. and Wells, W. M. (1997). Alignment by maximiza-
tion of mutual information. Int’l. Journal of Computer
Vision, 24:137–154.
Xu, C. and Prince, J. L. (1998). Snakes, shapes, and gra-
dient vector flow. IEEE Trans. on Image Processing,
7(3):359–369.
VISAPP 2008 - International Conference on Computer Vision Theory and Applications
524