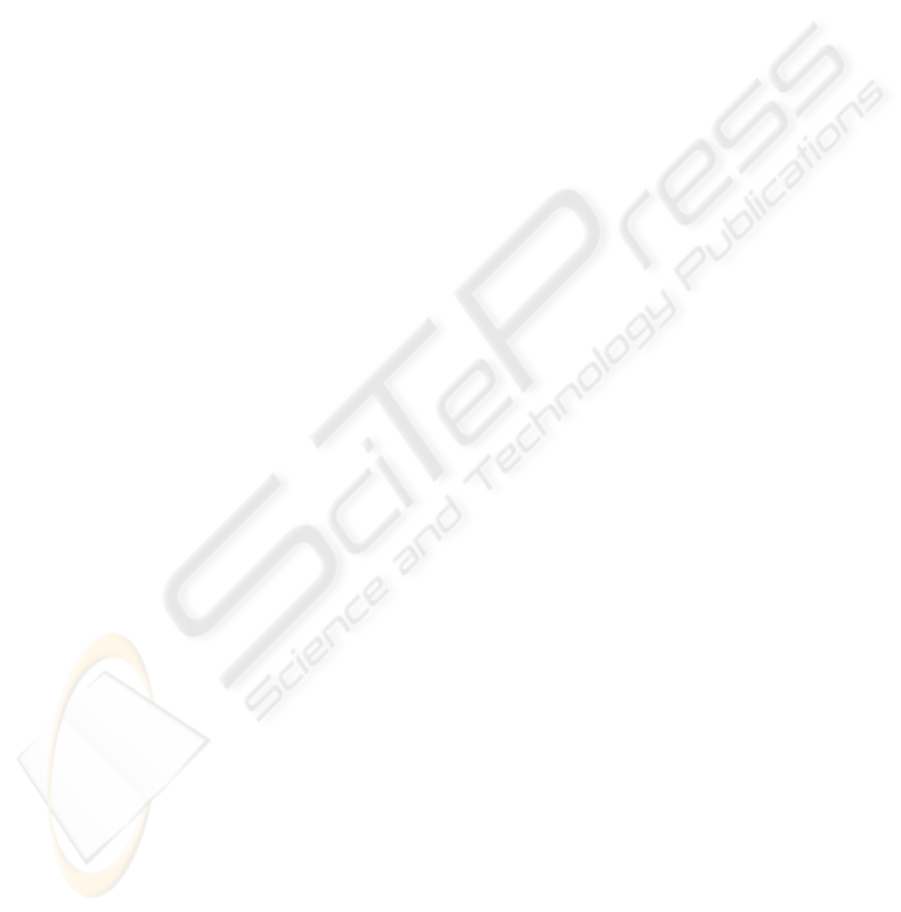
for each pixel and two additional nodes called termi-
nals. There is an edge {p,q} between any two distinct
nodes p and q. A cut C ⊂ E is a set of edges verify-
ing:
• Terminals are separated in the graph G (C ) =
hV ,E \ C i
• No subset of C separates terminals in G (C )
The minimum cut problem consists in finding the cut
C in a given graph with the lowest cost. The cost of a
cut, |C |, is the sum of its edges weights.
In (Boykov et al., 2001), Boykov et al. present two
algorithms based on graph cuts which find a local
minimum of the objective function (3) with respect to
two kinds of large moves: expansion moves and swap
moves. Large moves proceed by changing labels of a
large set of pixels simultaneously to decrease the ob-
jective function. In this work, we use the algorithm
based on swap moves because it handles more gen-
eral energy functionsand deals with the regularization
function that we have chosen.
4 EXPERIMENTATION
To validate the advantages of the method, we present
first experiments with synthetic data for noisy piece-
wise constant images. Then we experiment with real
images. The initialization starts with an arbitrary par-
tition of the desired number of regions to which arbi-
trary labels are assigned. the unique free parameter is
the regularization term penalty α.
4.1 Synthetic Data
Figure 1 shows synthetic images representing geo-
metric shapes in the first row, the segmentations ob-
tained using the method of (Boykovet al., 2001) in the
second row and the results of the segmentations with
our method in the third row. Contrary to the method
of (Boykov et al., 2001) which uses a set of labels
corresponding to all the possible grey levels, we have
the possibility to initialize the process of segmenta-
tion with the right number of regions, which is known
here, and with an arbitrary set of regions parameters.
The method of (Boykov et al., 2001) allows the num-
ber of labels to decrease due to the regularization term
but it initializes with the set of labels correspondingto
all possible grey levels, i.e., 256 grey levels. The final
segmentation is described by a subset of the initial set
of labels and is an implicit oversegmentation since no
explicit number of regions guides the segmentation.
As shown in figures 1(e)-(h), the segmentation is de-
scribed, in all cases, by a number of regions which is
much larger than the right number of regions: figure
1(e) is segmented into 98 regions, 1(f) is segmented
into 51 regions, 1(g) is segmented into 58 regions and
1(h) is segmented into 53 regions. On the other hand,
our method allows regions parameters to vary jointly
with the segmentation; thus, the initial set of labels is
arbitrarily chosen and the number of regions is fixed
equal to the right number.
In addition to that, our method realizes a big improve-
ment in term of running time since we deal with a
small number of regions compared to the method of
(Boykov et al., 2001). We show in Table 1 the running
times of the method of (Boykov et al., 2001) and our
method in the case of figures 1(a)-(d) and the corre-
sponding energies at convergence. In all these exam-
ples, the region merging process allows segmentation
of the images into the right number of regions and we
obtain exactly the same energy and regions parame-
ters at convergence in much less running time.
4.2 Real Data
Though the number of regions is not known, in gen-
eral, for real data, and consequently cannot be fixed in
advance, in most applications a maximum number of
regions is known, i.e., we know that the actual num-
ber of regions is less than some maximum number.
This maximum number is in all cases much smaller
than the number of all possible grey levels as used
in (Boykov et al., 2001). In this section, we deal with
real images and, depending on the application, we ini-
tialize with a number of regions that we consider large
enough to segment the image into an acceptable num-
ber of regions.
The method of (Boykov et al., 2001) is unable to seg-
ment these images into the desired number of regions
and it gives, in all cases, an oversegmentation, in ad-
dition to the much larger time that it takes to converge.
An image such as Figure 2(a) presents smooth varia-
tion of intensity inside the region of interest. Meth-
ods without control on the number of regions frag-
ment that region into multiple segments. The image
in figure 2(c) presents the biggest running time and
takes 2.90 seconds which is very small compared to
the method of (Boykov et al., 2001) that takes, for the
same image, more than 220 seconds because it deals
with all the possible grey levels and segments it into
200 segments.
MULTIREGION GRAPH CUT IMAGE SEGMENTATION
537