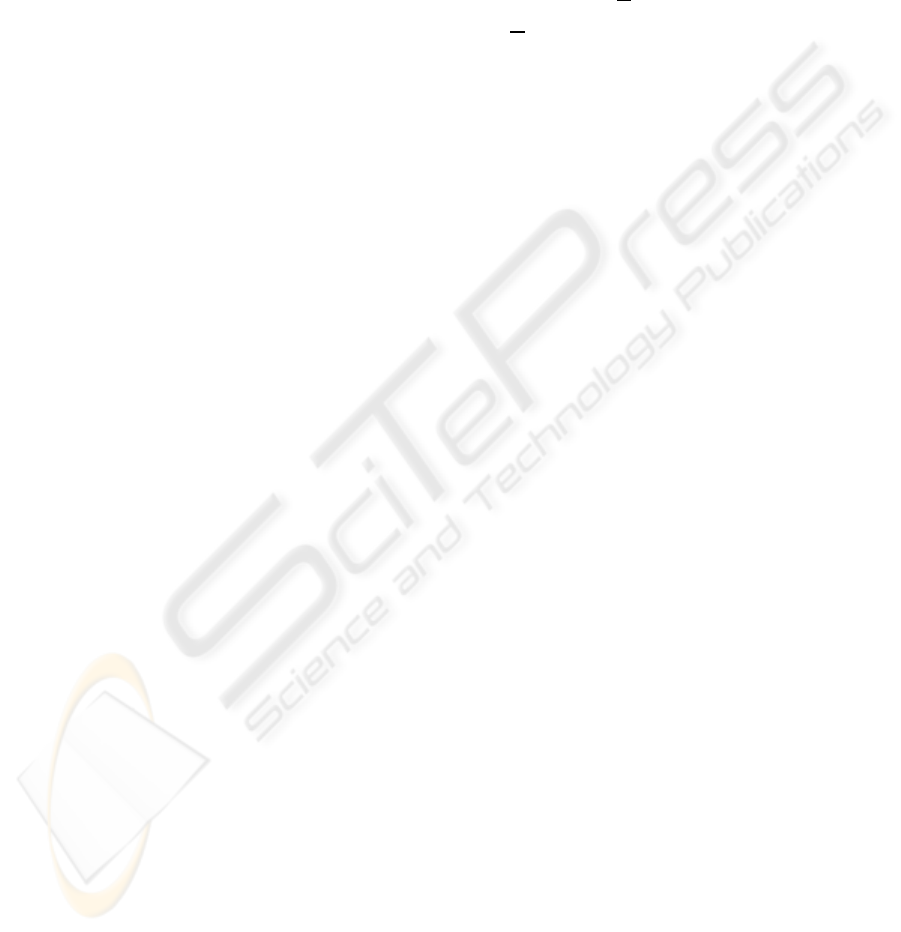
In this paper, we describe a brief implementation
of AAM, then we examine AAM on hand object
and finally compare AAM performance with another
model-based method named Active Shape Model
(ASM).
2 OFFLINE STAGE
This stage utilizes some statistical analysis by using
Principle Component Analysis (PCA) on the shape
variations and also texture variations of some gath-
ered images in a training set.
2.1 Manual Labeling
In the analyzed model, the shape is represented by
a set of points (or landmarks). These landmarks
are placed manually for each shape in the training
set. The corresponding landmarks in the shapes must
be approximately in the same location because each
point represents a particular part of the object or
its boundary. To increase accuracy some additional
points are added between two points when the dis-
tance is more than a threshold.
2.2 Shape Alignment
All objects in the training set has different scaling,
rotation and x-y position(or translation), named pose
parameters, compare to the others. In order to re-
move the pose differences and only remain the object
shape variations the alignment procedure is used. The
center of mass of the shape is calculated and moved
to the coordinate origin for removing X-Y transla-
tion. After removing the X-Y translation the obtained
shape becomes unity scale by dividing the shape on
its L
2
− norm. To remove the rotation, another shape
is needed as a reference. It can be proved mathe-
matically that Singular Value Decomposition (SVD)
calculates the rotation matrix between the shape and
the reference shape. A comprehensive explanation of
shape alignment with its procedure can be found in
(Babaii Rizvandi et al., 2007). The mentioned proce-
dure is only for one shape. For the shapes in the train-
ing set, we align all shapes to the first shape and cal-
culate the mean shape. Then we align all shapes to the
mean shape and we recalculate the mean shape. This
procedure, which aligns to the mean shape and recal-
culates the mean shape, is continued until the mean
shape does not change significantly in two iterations.
2.3 Statistical Shape Model
The 2N elements are highly correlated, so it is possi-
ble to represent them much more compactly. One ap-
proach is Principle Component Analysis (PCA) that
is widely used in pattern recognition to reduce the di-
mension. Using PCA the number of elements reduces
from 2N to M while M << 2N. The final shape model
is
X = X + Φ
T
shape
.b
shape
(1)
where X is the mean shape, Φ
shape
contains the shape
eigenvectors and b
shape
includes the shape parame-
ters.
2.4 Texture Sampling
The question to make a texture model is which gray
values must be used in the model and how the model
should be defined. The answer to the first question
is that only pixels including the object are necessary.
Dividing the shape into a combination of triangles by
delaunay triangulation is the common solution for the
second question.
The problem with delaunay triangulation is that
these triangles cover all regions including background
of the convex hull (Stegmann, 2000), (T.F.Cootes and
J.Graham, 1995). So in order to form a suitable tex-
ture model, a convex hull algorithm must be used.
After removing the background pixels, the next
step is to find the corresponding pixels in the object
textures and warp these pixels positions. To do this
task, the pixels inside the mean shape are sampled
and the related pixels in the other images textures in
the training set are obtained by using the correspond-
ing triangles. (Stegmann, 2000) and (Babaii Rizvandi
et al., 2007) explain the complete algorithm.
2.5 Texture Alignment
Within the object there are usually some variations
in gray values because of different illumination in-
tensities. Since the goal is to build a stable model
without these unwanted effects, these variations must
be eliminated. The common method is to align all
textures to the standardized mean texture, with zero
mean and unit variance, and continue this procedure
till the difference between the standardized mean tex-
ture in two following iterations is less than a threshold
[(T.F.Cootes and C.J.Taylor, 2001) ,(Stegmann, 2000)
and (Babaii Rizvandi et al., 2007)].
VISAPP 2008 - International Conference on Computer Vision Theory and Applications
540