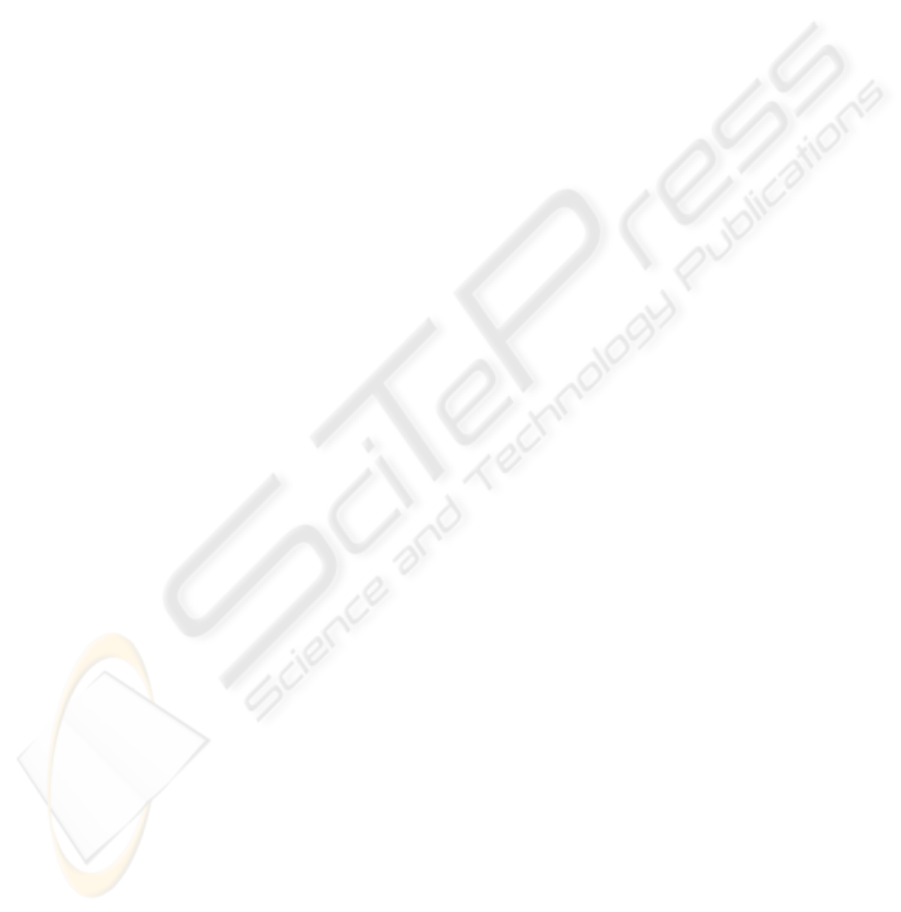
The mean error distance was up to 20 percent smaller
(for w = 0.6) using the modified tracker.
6 CONCLUSIONS AND
OUTLOOK
In this paper we showed a method to modify the well-
known KLT tracker incorporating knowledge about
the extrinsic and intrinsic camera parameters. The ad-
ditional prior knowledge is utilized to reparameterize
the warping function. With respect to noise in prac-
tical applications, uncertainty is modeled within the
optimization rule. While the mean trail length could
only be improved very slightly, the experiments per-
formed show a better accuracy when using the mod-
ified tracker. Remarkable is the fact that the epipolar
optimization directions alone have a positive effect on
the tracking result.
For the future, this modification of the KLT
tracker offers lots of further topics to be investigated.
Setting the weighting factor w to a certain value may
be replaced by an automatic detection concerning the
amount of uncertainty of the camera parameters. We
also think about changing w during the optimization
process.
Another step is the concurrent improvement of ac-
curacy and trail length. At the current stage, accu-
racy is addressed already. When aiming at longer trail
lengths, a closer look at the reasons of losing a feature
has to be taken. One of these reasons, surely, is a too
large error measured (cf. expression (1)) between cor-
responding patches. That means, the selected trans-
formation is not able to model all changes between
the patches within the error bound set. But with re-
gard to the (soft) epipolar constraint of the modified
tracker, this error bound may be raised without the op-
timization process losing its way. Another possibility
to be explored is random jumping along the epipolar
line, when a feature is lost.
REFERENCES
Baker, S. and Matthews, I. (2004). Lucas-kanade 20 years
on: A unifying framework. International Journal of
Computer Vision, 56:221–255.
Cox, I., Roy, S., and Hingorani, S. L. (1995). Dynamic
histogram warping of image pairs for constant image
brightness. IEEE International Conference on Image
Processing, 2:366–369.
Fusiello, A., Trucco, E., Tommasini, T., and Roberto, V.
(1999). Improving feature tracking with robust statis-
tics. Pattern Analysis and Applications, 2:312–320.
Hartley, R. and Zisserman, A. (2003). Multiple View Geom-
etry in computer vision, Second Edition. Cambridge
University Press.
Kuehmstedt, P., Notni, G., Hintersehr, J., and Gerber, J.
(2001). Cad-cam-system for dental purpose – an in-
dustrial application. In The 4th International Work-
shop on Automatic Processing of Fringe Patterns.
Lucas, B. and Kanade, T. (1981). An iterative image regis-
tration technique with an application to stereo vision.
In Proceedings of 7th International Joint Conference
on Artificial Intelligence.
Rav-Acha, A. and Peleg, S. (2006). Lucas-kanade without
iterative warping. In Proceedings of 2006 IEEE Inter-
national Conference on Image Processing.
Shi, J. and Tomasi, C. (1994). Good features to track. In
Proceedings of IEEE Computer Society Conference
on Computer Vision and Pattern Recognition.
Trummer, M., Denzler, J., and Suesse, H. (2006). Precise 3d
measurement with standard means and minimal user
interaction – extended single-view reconstruction. In
Proceedings of 17th International Conference on the
Application of Computer Science and Mathematics in
Architecture and Civil Engineering.
Wenhardt, S., Deutsch, B., Hornegger, J., Niemann, H., and
Denzler, J. (2006). An information theoretic approach
for next best view planning in 3-d reconstruction. In
The 18th International Conference on Pattern Recog-
nition.
Zinsser, T., Graessl, C., and Niemann, H. (2005). High-
speed feature point tracking. In Proceedings of Con-
ference on vision, Modeling and Visualization.
KLT TRACKING USING INTRINSIC AND EXTRINSIC CAMERA PARAMETERS IN CONSIDERATION OF
UNCERTAINTY
351