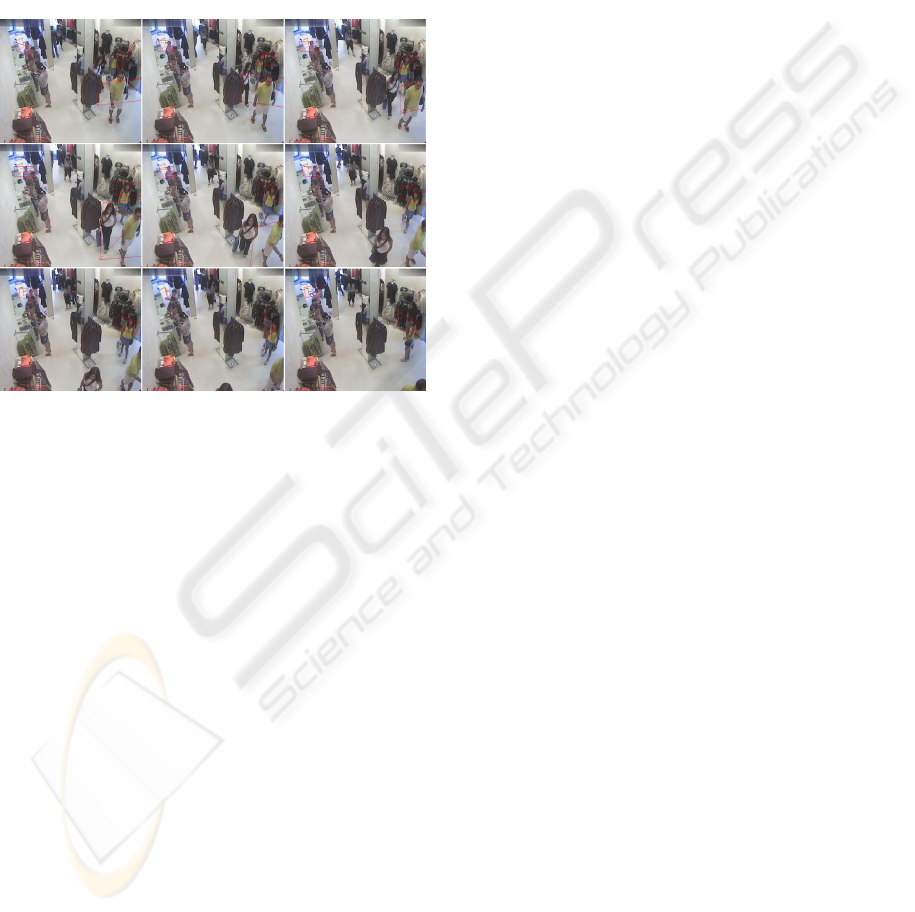
5 EXPERIMENTAL RESULTS
We represent the visual field by a quadrilateral (a
rectangle in the case of a front glance or trapezoidal
form in other cases). We have tested this method on
various datasets (see figures 2, 4, 5 and 6). Finally,
we demonstrate the method on videos taken in a
shop for 3 customers, in figure 6. This example
confirms that when the obstacle is placed far from a
person, the length and the height of his visual field
increase.
Figure 6: This final example demonstrates the method in a
shop for 3 customers.
6 CONCLUSIONS
In this paper, we have established that information
about the head pose and the estimated distance can
be used to compute the visual field of persons. We
demonstrate on a number of datasets that we obtain
the visual field of persons at a distance.
Our future work will focus on an accurate
method to detect automatically the head pose of the
persons. We will also combine this advance with
human behavior recognition to aid automatic
reasoning in video.
ACKNOWLEDGEMENTS
This work has been supported by the European
Commission within the Information Society
Technologies program (FP6-2005-IST-5), through
the project MIAUCE (www.miauce.org).
REFERENCES
Ba, S., Odobez, J., 2005. Evaluation of multiple cues
head-pose tracking algorithms in indoor environments.
In Proc. of Int. Conf. on Multimedia and Expo
(ICME), Amsterdam.
Brown, L., Tian, Y., 2002. A study of coarse head-pose
estimation. In Workshop on Motion and Video
Computing.
Gee, A., Cipolla, R., 1994. Estimating gaze from a single
view of a face. In Proc. International Conference on
Pattern Recognition (ICPR), Jerusalem.
Girard, P.K., 2004. Quaternions, algèbre de Clifford et
physique relativiste. PPUR.
Horprasert, A.T., Yacoob, Y., Davis, L.S., 1996.
Computing 3D head orientation from a monocular
image sequence. In Proc. of Intl. Society of Optical
Engineering (SPIE), Killington.
Liang, G., Zha, H., Liu, H., 2004. 3D model based head
pose tracking by using weighted depth and brightness
constraints. In Image and Graphics.
Moeslund, T.B., Hilton, A., Krüger, V., 2006. A survey of
advances in vision-based human motion capture and
analysis. In Computer Vision and Image
Understanding.
Panero, J., Zenik, M., 1979. Human dimension and
interior space, Withney Library of design,
Architectural Ltd. London.
Rae, R., Ritter, H., 1998. Recognition of human head
orientation based on artificial neural networks. In
IEEE Trans. on Neural Networks, 9(2):257–265.
Robertson, N.M., Reid, I.D., Brady, J.M., 2005. What are
you looking at? Gaze recognition in medium-scale
images, In Human Activity Modelling and
Recognition, British Machine Vision Conference.
Smith, K., Ba, S.O., Gatica-Perez, D., Odobez, J-M., 2006.
Tracking the multi person wandering visual focus of
attention. In ICMI '06: Proceedings of the 8th
international conference on Multimodal interfaces,
Banff.
Srinivasan, S., Boyer, K., 2002. Head-pose estimation
using view based eigenspaces. In Proc. of Intl.
Conference on Pattern Recognition (ICPR), Quebec.
Stiefelhagen, R., Finke, M., Waibel, A., 1996. A model-
based gaze tracking system. In Proc. of Intl. Joint
Symposia on Intelligence and Systems.
Yang, R., Zhang, Z., 2001. Model-based head-pose
tracking with stereo vision. In Technical Report MSR-
TR-2001-102, Microsoft Research.
Zhao, L., Pingali, G., Carlbom, I., 2002. Real-time head
orientation estimation using neural networks. In Proc.
of the Intl. Conference on Image Processing (ICIP),
Rochester, NY.
VISAPP 2008 - International Conference on Computer Vision Theory and Applications
316