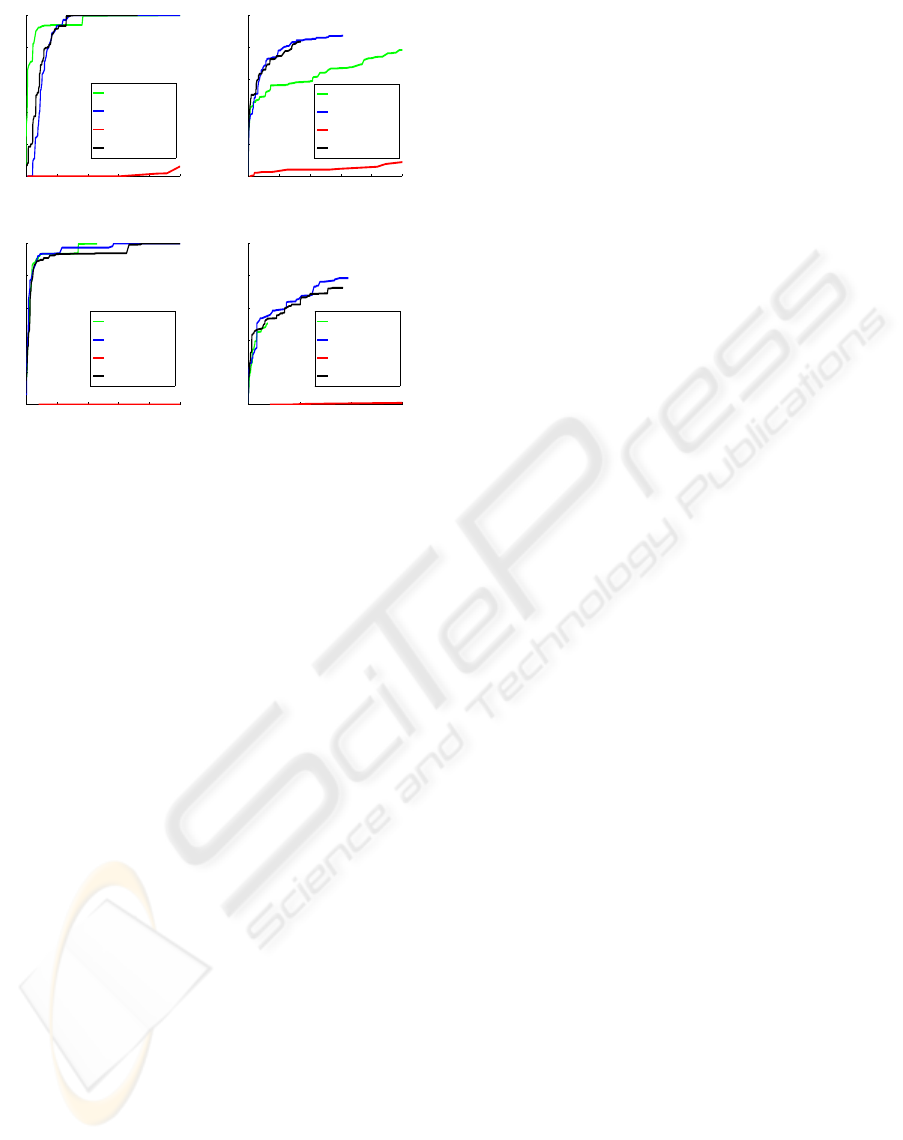
0 0.01 0.02 0.03 0.04 0.05
0
0.2
0.4
0.6
0.8
1
6−bins RGB
12−bins rb
12−bins OEH
12−bins rb+OEH
0 0.01 0.02 0.03 0.04 0.05
0
0.2
0.4
0.6
0.8
1
6−bins RGB
12−bins rb
12−bins OEH
12−bins rb+OEH
(a) (b)
0 0.005 0.01 0.015 0.02 0.025
0
0.2
0.4
0.6
0.8
1
6−bins RGB
12−bins rb
12−bins OEH
12−bins rb+OEH
0 0.005 0.01 0.015
0
0.2
0.4
0.6
0.8
1
6−bins RGB
12−bins rb
12−bins OEH
12−bins rb+OEH
(c) (d)
Figure 6: Comparison of ROC curves obtained on original
image of figure 3(a), with different feature types: 6
3
-bins
RGB color histogram, 12
2
-bins rb color histogram, 12-bins
edge orientation histogram, 12
2
-bins rb color and 12-bins
edge orientation histograms concatenated. In the first col-
umn, ground truth is ”No entry” signs, when in second col-
umn it is subjects’ fixations obtained by eye-tracker from 3
subjects. On the first line, Laplace Kernel is used when in
the second line it is the triangular kernel.
used as ground-truth for building two kinds of ROC
curves. The used parameter to draw the ROC curves
is the threshold on the confidence map for each fea-
ture. Four features were used: 6
3
-bins RGB color
histogram, 12
2
-bins rb color histogram, 12-bins edge
orientation histogram, 12
2
-bins rb color and 12-bins
edge orientation histograms concatenated. In figure 6,
the obtained ROC curves are displayed. On the left
column, the ground truth is ”No entry” signs, when
on the right column it is subjects’ fixations obtained
by eye-tracker from 3 subjects. Two different ker-
nels were used. On the first line, Laplace Kernel is
used when in the second line it is the triangular ker-
nel. In most of the cases, the best result is obtained
using 12
2
-bins rb color histogram.
6 CONCLUSIONS
We propose a new paradigm to define conspicuity in-
cluding visual priors on the object of interest. From
our preliminary experiments with subjects, this new
model seems to outperform the saliency map model.
We investigate the problem of choosing the right fea-
tures to describe a specific sign in images, and we
found that 12
2
-bins rb color histogram gives best per-
formances in most cases. We also investigate the in-
fluence of the choice of the kernel an we found that
triangular kernel leads to better and faster results. In
future work, we will continue to test our model using
the eye-tracker to validate the proposed paradigm and
to refine our conclusions.
REFERENCES
Bremond, R., Tarel, J.-P., Choukour, H., and Deugnier, M.
(2006). La saillance visuelle des objets routiers, un
indicateur de la visibilit´e routi`ere. In Proceedings of
Journ´ees des Sciences de l’Ing´enieur (JSI’06), Marne
la Vall´ee, France.
CIE137 (2000). The conspicuity of traffic signs in com-
plex backgrounds. In CIE137, Technical report of the
Commision Internationale de L’Eclairage (CIE).
Fleuret, F. and Sahbi, H. (2003). Scale-invariance of sup-
port vector machines based on the triangular kernel. In
Proceedings of IEEE International Workshop on Sta-
tistical and Computational Theories of Vision, Nice,
France.
Gao, D. and Vasconcelos, N. (2004). Discriminant saliency
for visual recognition from cluttered scenes. In NIPS.
Itti, L., Koch, C., and Niebur, E. (1998). A model of
saliency-based visual attention for rapid scene anal-
ysis. IEEE Transactions on Pattern Analysis and Ma-
chine Intelligence, 20(11):1254–1259.
Navalpakkam, V. and Itti, L. (2005). Modeling the influence
of task on attention. Vision Research, 45(2):205–231.
Sch¨olkopf, B. and Smola, A. (2002). Learning with Kernels.
MIT Press, Cambridge, MA, USA.
Simon, L., Tarel, J.-P., and Bremond, R. (2007). A new
paradigm for the computation of conspicuity of traffic
signs in road images. In Proceedings of 26th session of
Commision Internationale de L’Eclairage (CIE’07),
volume II, pages 161 – 164, Beijing, China.
Sundstedt, V., Debattista, K., Longhurst, P., Chalmers, A.,
and Troscianko, T. (2005). Visual attention for effi-
cient high-fidelity graphics. In Spring Conference on
Computer Graphics (SCCG 2005), pages 162–168.
Underwood, G., Foulsham, T., van Loon, E., Humphreys,
L., and Bloyce, J. (2006). Eye movements during
scene inspection: A test of the saliency map hy-
pothesis. European Journal of Cognitive Psychology,
18(3):321–342.
Vapnik, V. (1999). The Nature of Statistical Learning The-
ory. Springer Verlag, 2nd edition, New York.
VISAPP 2008 - International Conference on Computer Vision Theory and Applications
328